A Scalable BFloat16 Dot-Product Architecture for Deep Learning
GLSVLSI '23: Proceedings of the Great Lakes Symposium on VLSI 2023(2023)
Abstract
BFloat16(BF16) format has recently driven the development of deep learning due to its higher energy efficiency and less memory consumption than the traditional format. This paper presents a scalable BF16 dot-product(DoP) architecture for high-performance deep-learning computing. A novel 4-term DoP unit is proposed as a fundamental module in the architecture, which performs 4-term DoP operation in three cycles. More-term DoP units are constructed through the extension of the fundamental unit, in which early exponent comparison is performed to hide latency, and intermediate normalization and rounding are omitted to improve accuracy and further reduce latency. Compared with the discrete design, the proposed architecture reduces latency by 22.8% for 4-term DoP, and a larger proportion of latency is reduced as the size of the DoP operation increases. Compared with existing designs for BF16, the proposed architecture at 64-term exhibits better-normalized energy efficiency and higher throughput with at least 1.88× and 20.3× improvement, respectively.
MoreTranslated text
Key words
BF16, dot-product operation, scalable architecture, deep learning
AI Read Science
Must-Reading Tree
Example
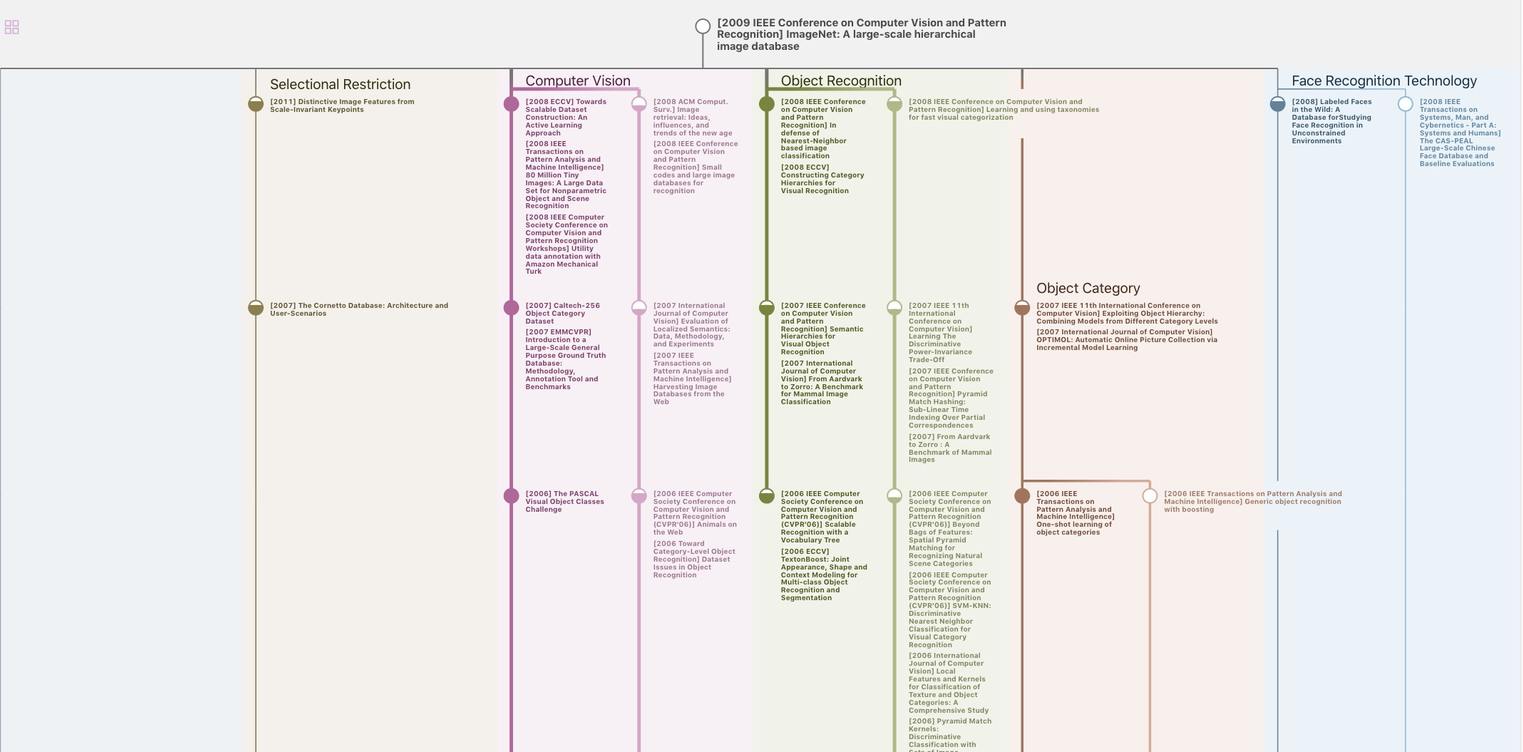
Generate MRT to find the research sequence of this paper
Chat Paper
Summary is being generated by the instructions you defined