Reconfigurable Mapping Algorithm based Stuck-At-Fault Mitigation in Neuromorphic Computing Systems
GLSVLSI '23: Proceedings of the Great Lakes Symposium on VLSI 2023(2023)
摘要
Stuck-At-Fault (SAF) defect of memristor generated from immature fabrication and heavy device utilization makes neuromorphic computing systems commercially unavailable. To mitigate this problem, a Reconfigurable Mapping Algorithm (RMA) is proposed in this paper. Based on the analysis for the VGG8 model with CIFAR10 dataset, the experiment results show that the RMA is efficient in restoring the inference accuracy up to 90% (the original accuracy without SAF) under SAFs from 0.1% to 50%, where Stuck-At-One (SA1): Stuck-At-Zero (SA0) = 5:1, 1:5, and 1:1. Additionally, the RMA improves the accuracy more than 50% in presence of high nonlinearity LTP = 4 and LTD = -4 and the standard conductance drift (10 years at 85 degrees Celsius) nearly has no influence on the inference accuracy of the DNN with the RMA.
更多查看译文
关键词
memristor, deep neural network (DNN), neuromporphic computing system, stuck-at-fault (SAF), inference accuracy
AI 理解论文
溯源树
样例
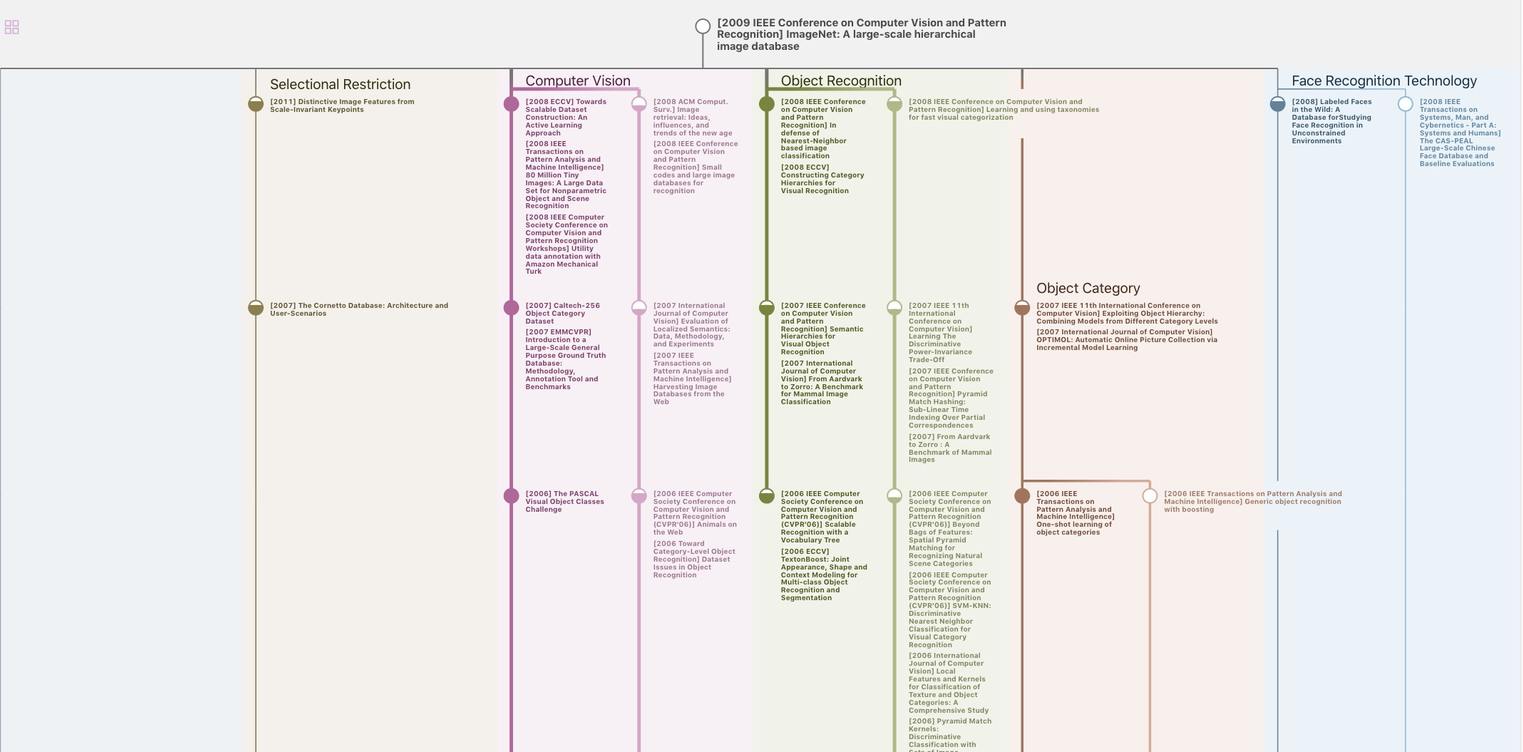
生成溯源树,研究论文发展脉络
Chat Paper
正在生成论文摘要