INDigo: An INN-Guided Probabilistic Diffusion Algorithm for Inverse Problems
2023 IEEE 25TH INTERNATIONAL WORKSHOP ON MULTIMEDIA SIGNAL PROCESSING, MMSP(2023)
摘要
Recently it has been shown that using diffusion models for inverse problems can lead to remarkable results. However, these approaches require a closed-form expression of the degradation model and can not support complex degradations. To overcome this limitation, we propose a method (INDigo) that combines invertible neural networks (INN) and diffusion models for general inverse problems. Specifically, we train the forward process of INN to simulate an arbitrary degradation process and use the inverse as a reconstruction process. During the diffusion sampling process, we impose an additional data-consistency step that minimizes the distance between the intermediate result and the INN-optimized result at every iteration, where the INN-optimized image is composed of the coarse information given by the observed degraded image and the details generated by the diffusion process. With the help of INN, our algorithm effectively estimates the details lost in the degradation process and is no longer limited by the requirement of knowing the closed-form expression of the degradation model. Experiments demonstrate that our algorithm obtains competitive results compared with recently leading methods both quantitatively and visually. Moreover, our algorithm performs well on more complex degradation models and real-world low-quality images.
更多查看译文
关键词
inverse problems,diffusion models,invertible neural networks
AI 理解论文
溯源树
样例
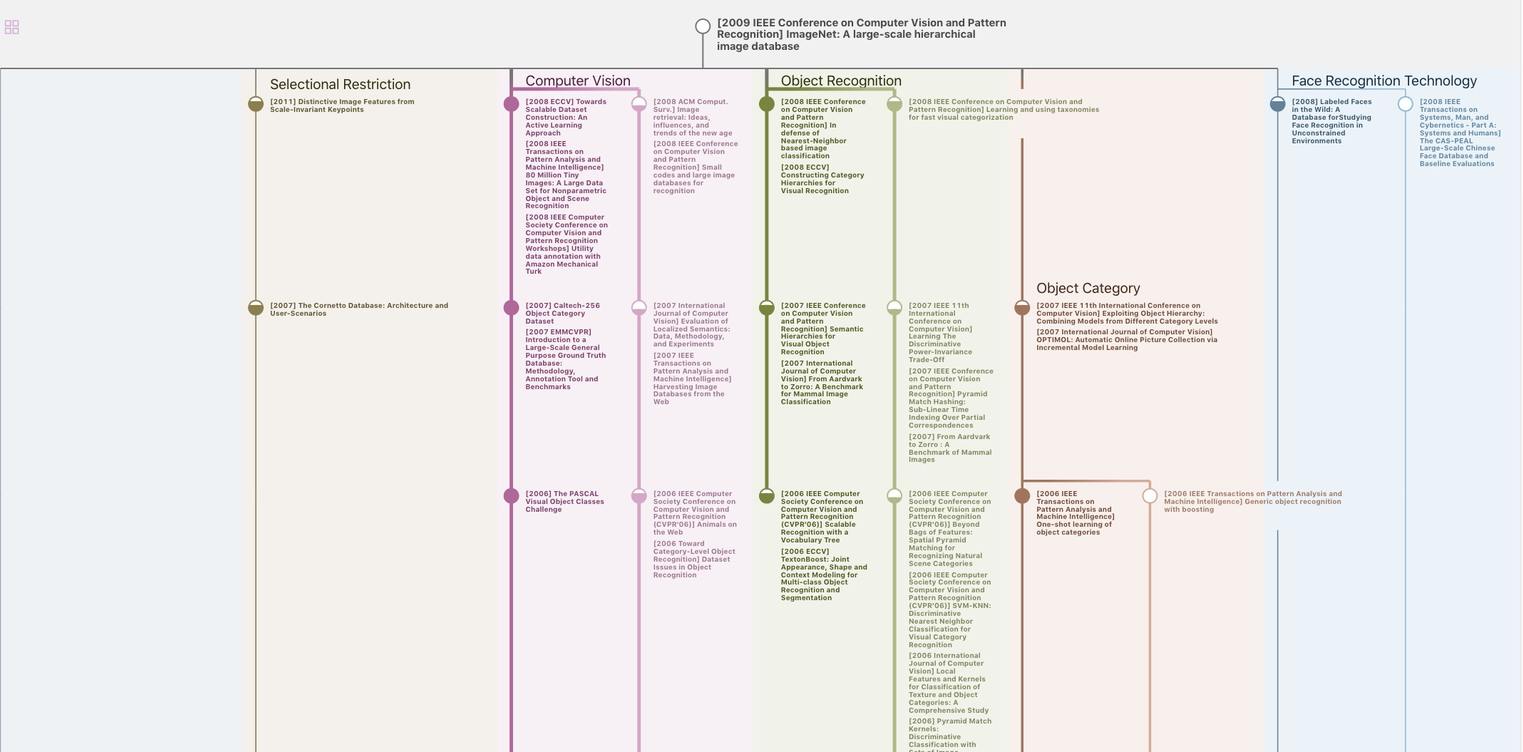
生成溯源树,研究论文发展脉络
Chat Paper
正在生成论文摘要