EffCRN: An Efficient Convolutional Recurrent Network for High-Performance Speech Enhancement
arXiv (Cornell University)(2023)
摘要
Fully convolutional recurrent neural networks (FCRNs) have shown state-of-the-art performance in single-channel speech enhancement. However, the number of parameters and the FLOPs/second of the original FCRN are restrictively high. A further important class of efficient networks is the CRUSE topology, serving as reference in our work. By applying a number of topological changes at once, we propose both an efficient FCRN (FCRN15), and a new family of efficient convolutional recurrent neural networks (EffCRN23, EffCRN23lite). We show that our FCRN15 (875K parameters) and EffCRN23lite (396K) outperform the already efficient CRUSE5 (85M) and CRUSE4 (7.2M) networks, respectively, w.r.t. PESQ, DNSMOS and DeltaSNR, while requiring about 94% less parameters and about 20% less #FLOPs/frame. Thereby, according to these metrics, the FCRN/EffCRN class of networks provides new best-in-class network topologies for speech enhancement.
更多查看译文
关键词
efficient convolutional recurrent network,enhancement,speech,high-performance
AI 理解论文
溯源树
样例
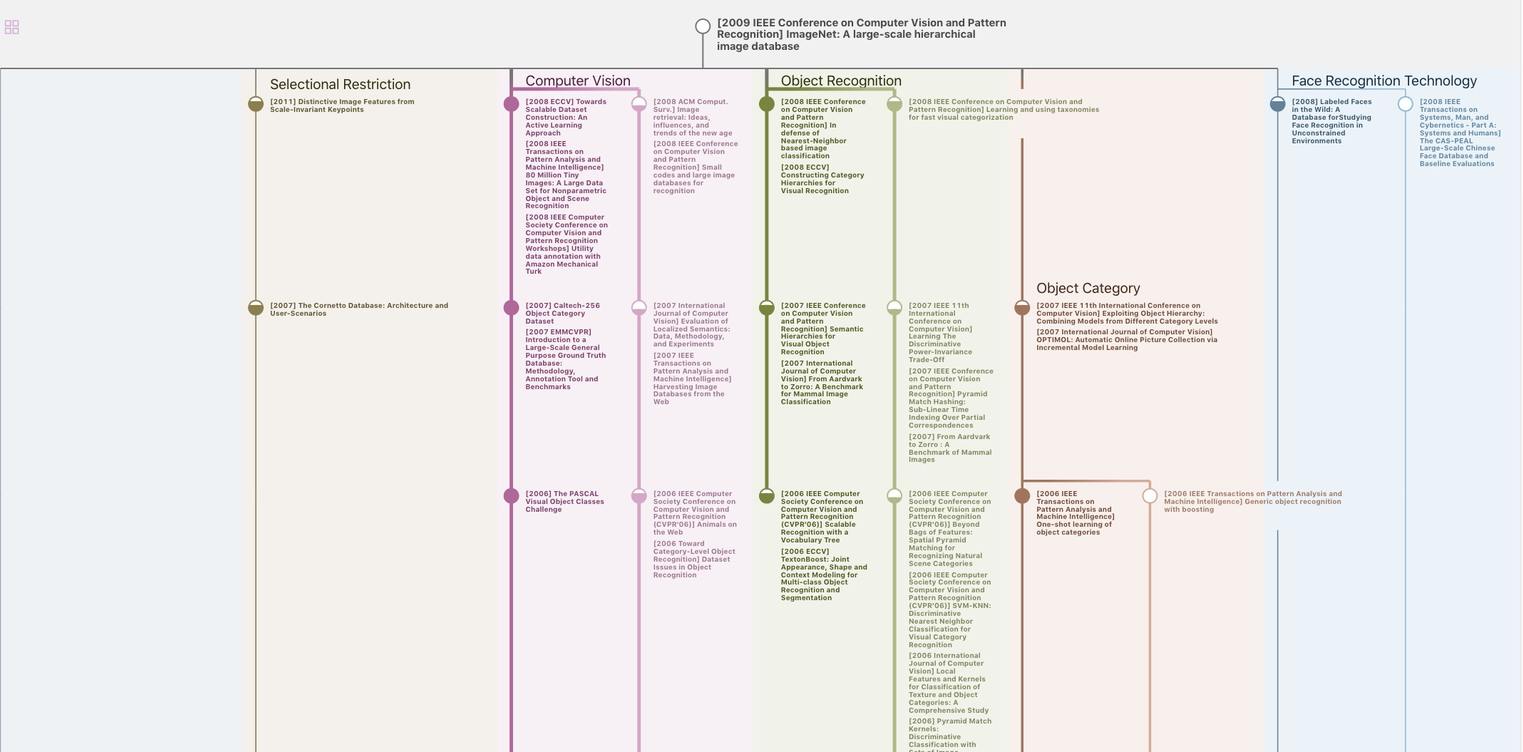
生成溯源树,研究论文发展脉络
Chat Paper
正在生成论文摘要