A Privacy-Preserving Federated Learning Approach for Kernel methods
CoRR(2023)
摘要
It is challenging to implement Kernel methods, if the data sources are distributed and cannot be joined at a trusted third party for privacy reasons. It is even more challenging, if the use case rules out privacy-preserving approaches that introduce noise. An example for such a use case is machine learning on clinical data. To realize exact privacy preserving computation of kernel methods, we propose FLAKE, a Federated Learning Approach for KErnel methods on horizontally distributed data. With FLAKE, the data sources mask their data so that a centralized instance can compute a Gram matrix without compromising privacy. The Gram matrix allows to calculate many kernel matrices, which can be used to train kernel-based machine learning algorithms such as Support Vector Machines. We prove that FLAKE prevents an adversary from learning the input data or the number of input features under a semi-honest threat model. Experiments on clinical and synthetic data confirm that FLAKE is outperforming the accuracy and efficiency of comparable methods. The time needed to mask the data and to compute the Gram matrix is several orders of magnitude less than the time a Support Vector Machine needs to be trained. Thus, FLAKE can be applied to many use cases.
更多查看译文
关键词
federated learning approach,privacy-preserving privacy-preserving,learning approach
AI 理解论文
溯源树
样例
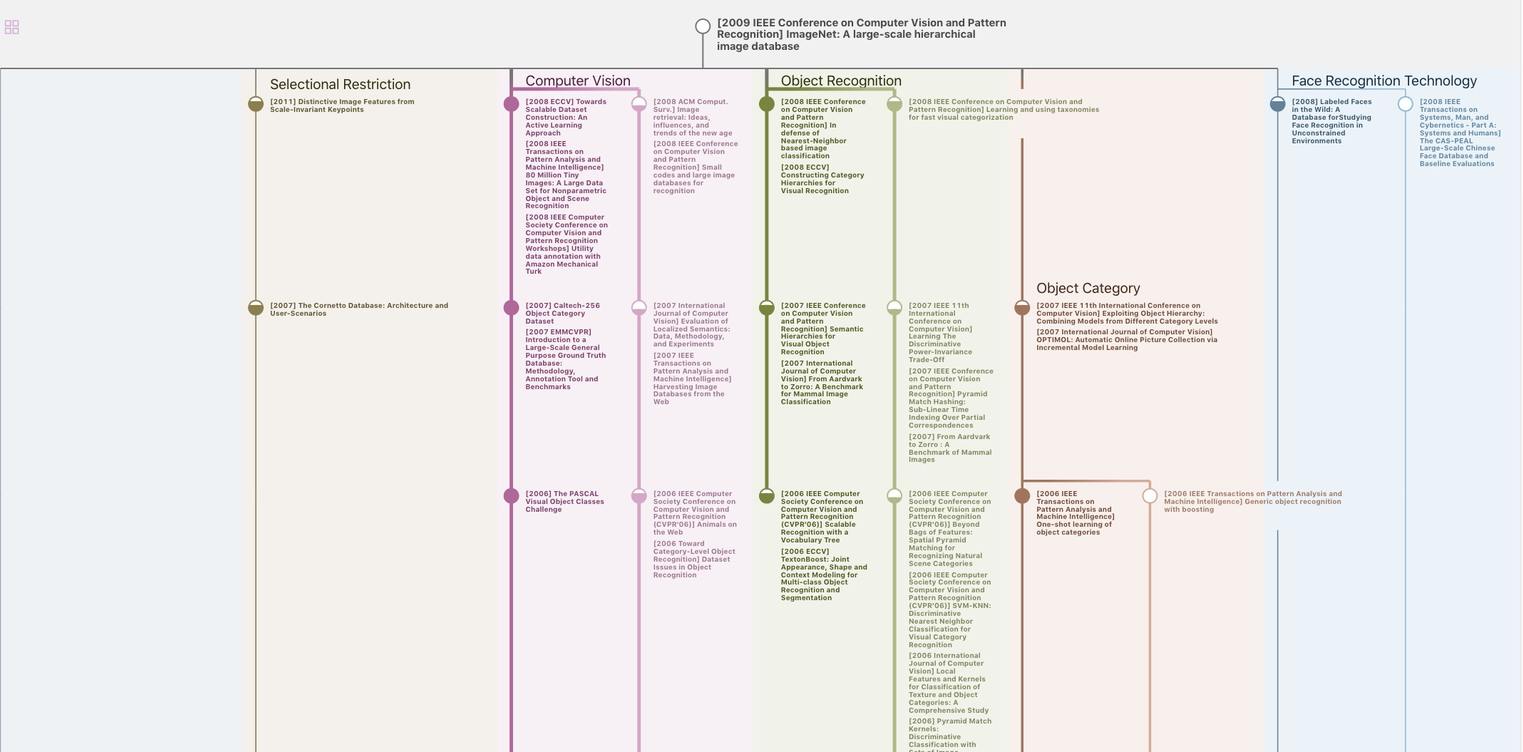
生成溯源树,研究论文发展脉络
Chat Paper
正在生成论文摘要