Cross-Modal Vertical Federated Learning for MRI Reconstruction.
CoRR(2024)
摘要
Federated learning enables multiple hospitals to cooperatively learn a shared model without privacy disclosure. Existing methods often take a common assumption that the data from different hospitals have the same modalities. However, such a setting is difficult to fully satisfy in practical applications, since the imaging guidelines may be different between hospitals, which makes the number of individuals with the same set of modalities limited. To this end, we formulate this practical-yet-challenging cross-modal vertical federated learning task, in which data from multiple hospitals have different modalities with a small amount of multi-modality data collected from the same individuals. To tackle such a situation, we develop a novel framework, namely Federated Consistent Regularization constrained Feature Disentanglement (Fed-CRFD), for boosting MRI reconstruction by effectively exploring the overlapping samples (i.e., same patients with different modalities at different hospitals) and solving the domain shift problem caused by different modalities. Particularly, our Fed-CRFD involves an intra-client feature disentangle scheme to decouple data into modality-invariant and modality-specific features, where the modality-invariant features are leveraged to mitigate the domain shift problem. In addition, a cross-client latent representation consistency constraint is proposed specifically for the overlapping samples to further align the modality-invariant features extracted from different modalities. Hence, our method can fully exploit the multi-source data from hospitals while alleviating the domain shift problem. Extensive experiments on two typical MRI datasets demonstrate that our network clearly outperforms state-of-the-art MRI reconstruction methods. The source code is available at https://github.com/IAMJackYan/FedCRFD.
更多查看译文
关键词
Cross-Modal,Domain Shift,MRI Reconstruction,Vertical Federated Learning
AI 理解论文
溯源树
样例
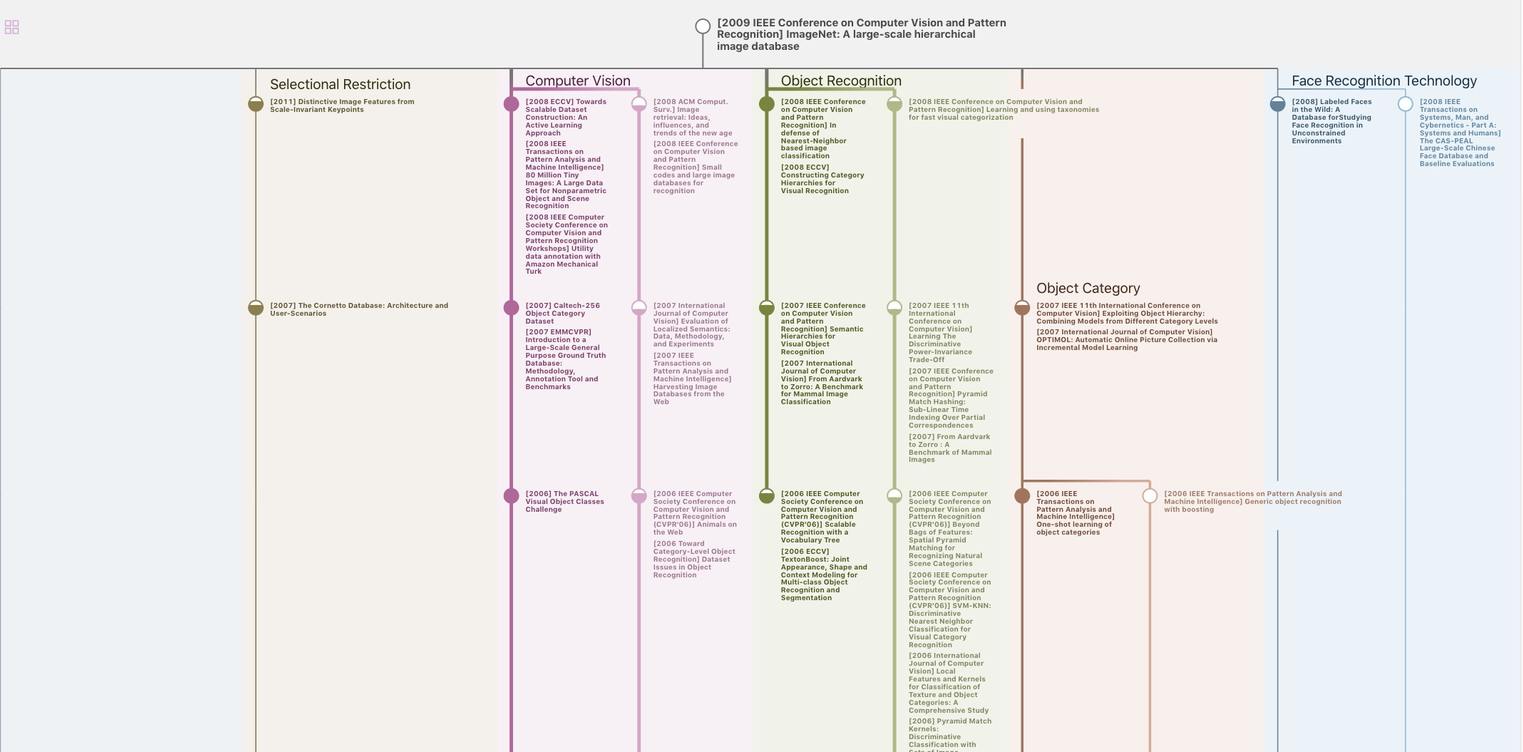
生成溯源树,研究论文发展脉络
Chat Paper
正在生成论文摘要