Bad Habits: Policy Confounding and Out-of-Trajectory Generalization in RL
CoRR(2023)
摘要
Reinforcement learning agents may sometimes develop habits that are effective only when specific policies are followed. After an initial exploration phase in which agents try out different actions, they eventually converge toward a particular policy. When this occurs, the distribution of state-action trajectories becomes narrower, and agents start experiencing the same transitions again and again. At this point, spurious correlations may arise. Agents may then pick up on these correlations and learn state representations that do not generalize beyond the agent's trajectory distribution. In this paper, we provide a mathematical characterization of this phenomenon, which we refer to as policy confounding, and show, through a series of examples, when and how it occurs in practice.
更多查看译文
关键词
policy confounding,habits,out-of-trajectory
AI 理解论文
溯源树
样例
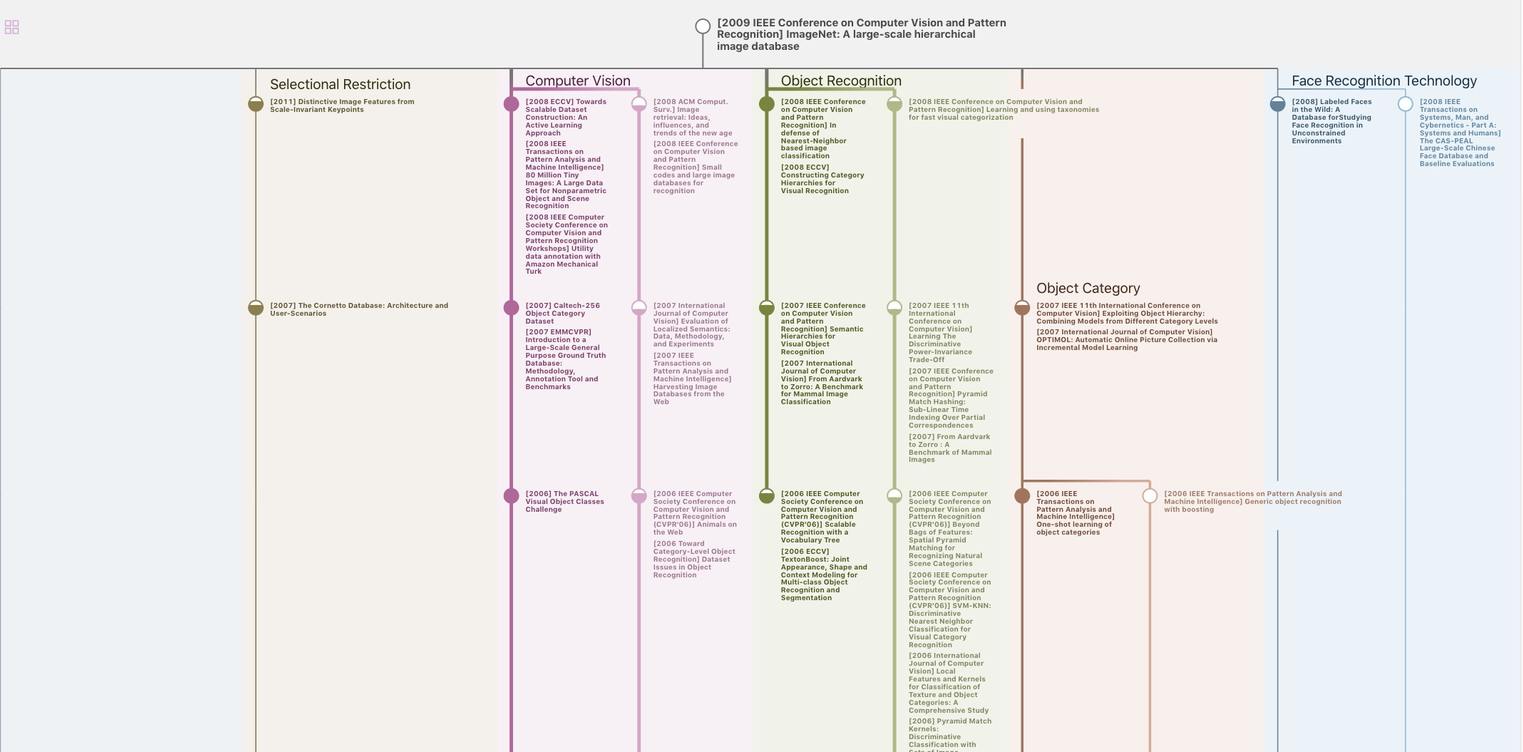
生成溯源树,研究论文发展脉络
Chat Paper
正在生成论文摘要