Scale Guided Hypernetwork for Blind Super-Resolution Image Quality Assessment
CoRR(2023)
摘要
With the emergence of image super-resolution (SR) algorithm, how to blindly evaluate the quality of super-resolution images has become an urgent task. However, existing blind SR image quality assessment (IQA) metrics merely focus on visual characteristics of super-resolution images, ignoring the available scale information. In this paper, we reveal that the scale factor has a statistically significant impact on subjective quality scores of SR images, indicating that the scale information can be used to guide the task of blind SR IQA. Motivated by this, we propose a scale guided hypernetwork framework that evaluates SR image quality in a scale-adaptive manner. Specifically, the blind SR IQA procedure is divided into three stages, i.e., content perception, evaluation rule generation, and quality prediction. After content perception, a hypernetwork generates the evaluation rule used in quality prediction based on the scale factor of the SR image. We apply the proposed scale guided hypernetwork framework to existing representative blind IQA metrics, and experimental results show that the proposed framework not only boosts the performance of these IQA metrics but also enhances their generalization abilities. Source code will be available at https://github.com/JunFu1995/SGH.
更多查看译文
关键词
super-resolution super-resolution,quality
AI 理解论文
溯源树
样例
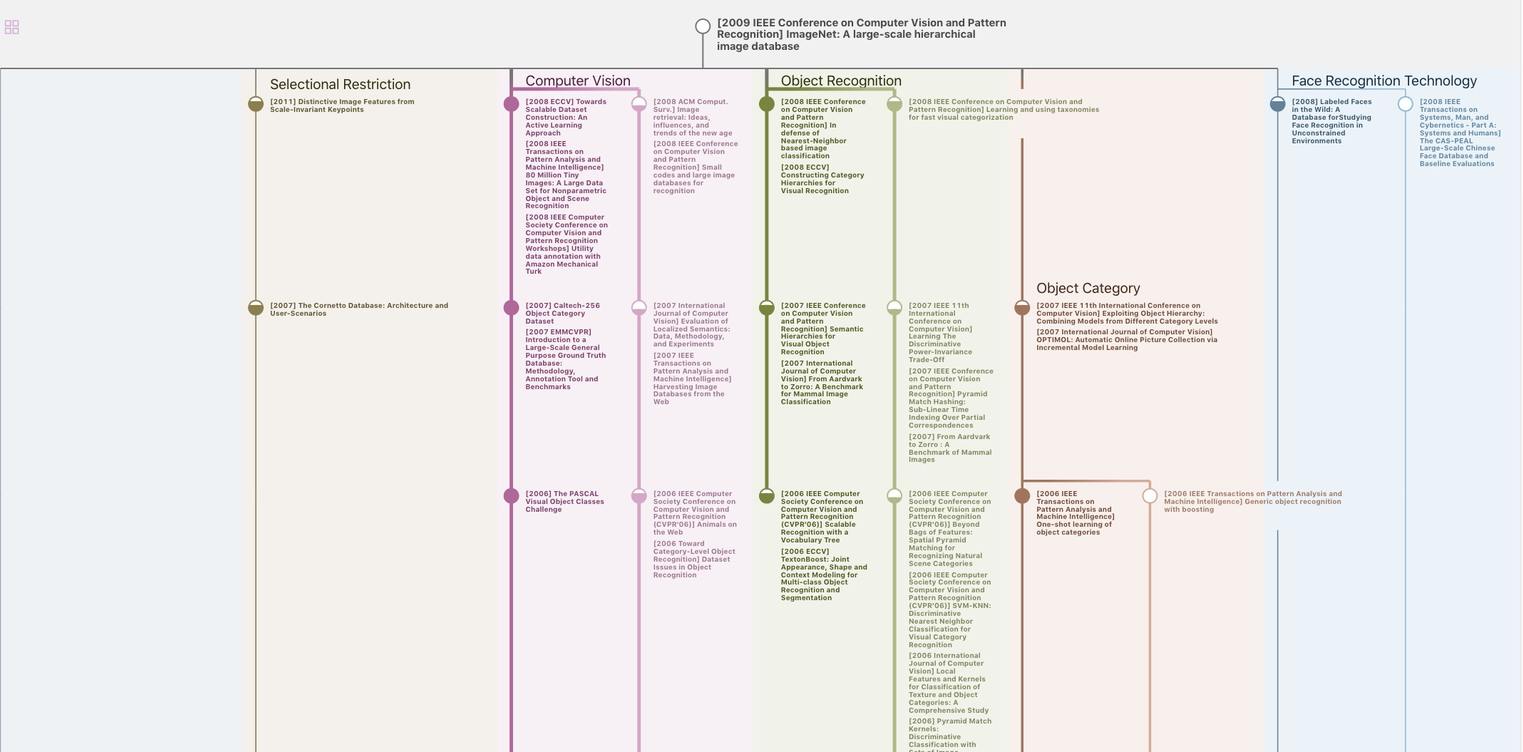
生成溯源树,研究论文发展脉络
Chat Paper
正在生成论文摘要