Maximum Likelihood Training of Autoencoders
CoRR(2023)
摘要
Maximum likelihood training has favorable statistical properties and is popular for generative modeling, especially with normalizing flows. On the other hand, generative autoencoders promise to be more efficient than normalizing flows due to the manifold hypothesis. In this work, we introduce successful maximum likelihood training of unconstrained autoencoders for the first time, bringing the two paradigms together. To do so, we identify and overcome two challenges: Firstly, existing maximum likelihood estimators for free-form networks are unacceptably slow, relying on iteration schemes whose cost scales linearly with latent dimension. We introduce an improved estimator which eliminates iteration, resulting in constant cost (roughly double the runtime per batch of a vanilla autoencoder). Secondly, we demonstrate that naively applying maximum likelihood to autoencoders can lead to divergent solutions and use this insight to motivate a stable maximum likelihood training objective. We perform extensive experiments on toy, tabular and image data, demonstrating the competitive performance of the resulting model. We call our model the maximum likelihood autoencoder (MLAE).
更多查看译文
关键词
autoencoders,training
AI 理解论文
溯源树
样例
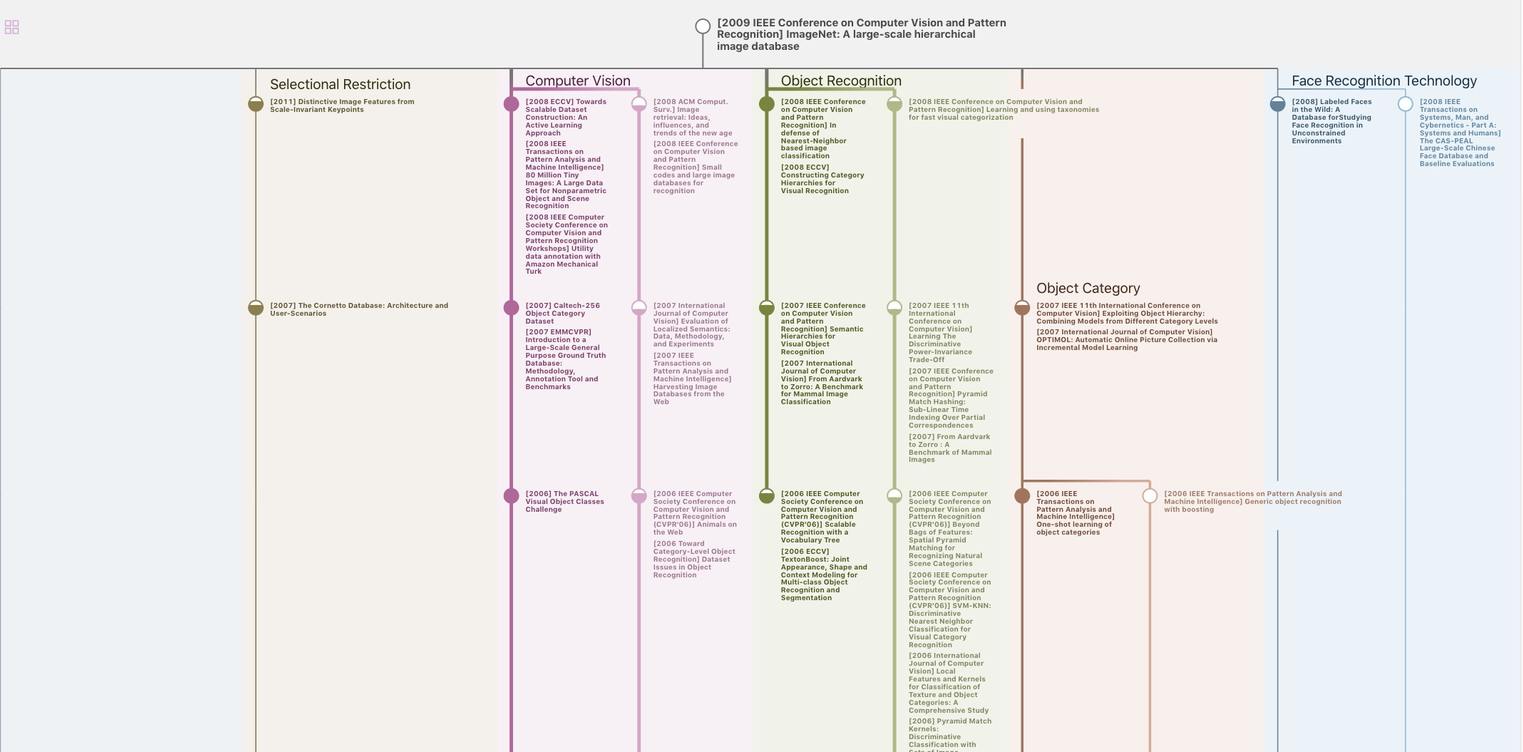
生成溯源树,研究论文发展脉络
Chat Paper
正在生成论文摘要