Combining stochastic density functional theory with deep potential molecular dynamics to study warm dense matter
MATTER AND RADIATION AT EXTREMES(2024)
Abstract
In traditional finite-temperature Kohn-Sham density functional theory (KSDFT), the partial occupation of a large number of high-energy KS eigenstates restricts the use of first-principles molecular dynamics methods at extremely high temperatures. However, stochastic density functional theory (SDFT) can overcome this limitation. Recently, SDFT and the related mixed stochastic-deterministic density functional theory, based on a plane-wave basis set, have been implemented in the first-principles electronic structure software ABACUS [Q. Liu and M. Chen, Phys. Rev. B 106, 125132 (2022)]. In this study, we combine SDFT with the Born-Oppenheimer molecular dynamics method to investigate systems with temperatures ranging from a few tens of eV to 1000 eV. Importantly, we train machine-learning-based interatomic models using the SDFT data and employ these deep potential models to simulate large-scale systems with long trajectories. Subsequently, we compute and analyze the structural properties, dynamic properties, and transport coefficients of warm dense matter.
MoreTranslated text
Key words
molecular dynamics,stochastic density
AI Read Science
Must-Reading Tree
Example
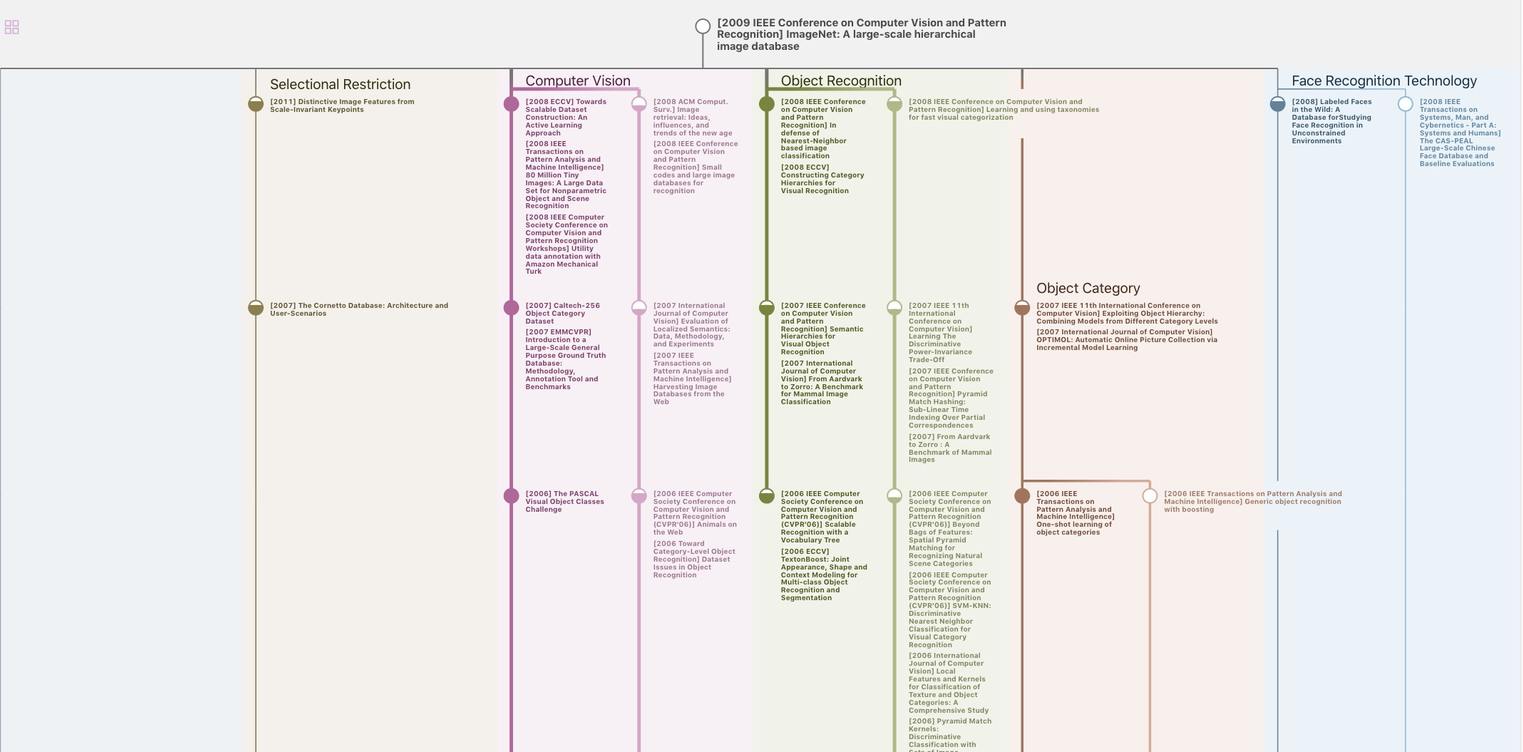
Generate MRT to find the research sequence of this paper
Chat Paper
Summary is being generated by the instructions you defined