KL-Divergence Guided Temperature Sampling
CoRR(2023)
摘要
Temperature sampling is a conventional approach to diversify large language model predictions. As temperature increases, the prediction becomes diverse but also vulnerable to hallucinations -- generating tokens that are sensible but not factual. One common approach to mitigate hallucinations is to provide source/grounding documents and the model is trained to produce predictions that bind to and are attributable to the provided source. It appears that there is a trade-off between diversity and attribution. To mitigate any such trade-off, we propose to relax the constraint of having a fixed temperature over decoding steps, and a mechanism to guide the dynamic temperature according to its relevance to the source through KL-divergence. Our experiments justifies the trade-off, and shows that our sampling algorithm outperforms the conventional top-k and top-p algorithms in conversational question-answering and summarization tasks.
更多查看译文
关键词
temperature,kl-divergence
AI 理解论文
溯源树
样例
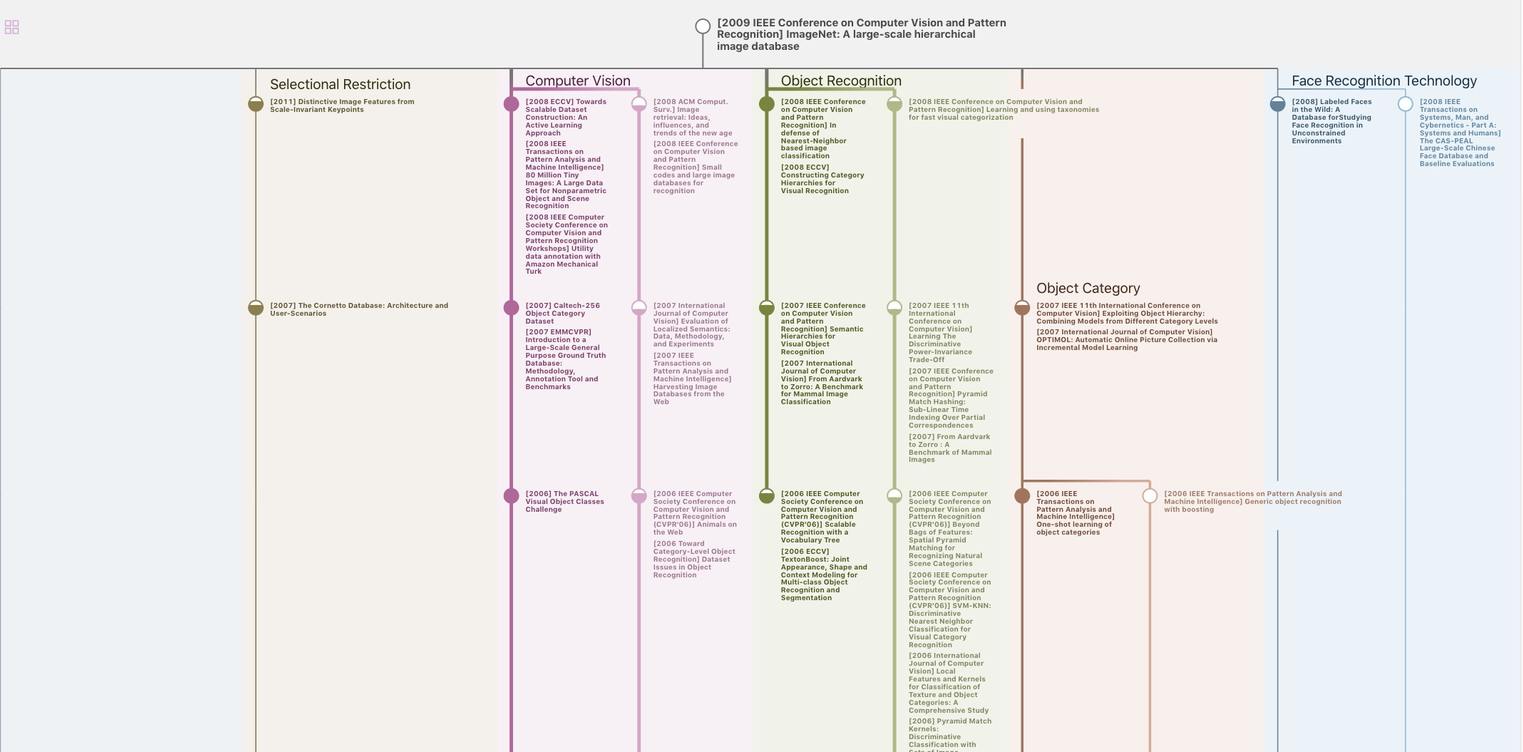
生成溯源树,研究论文发展脉络
Chat Paper
正在生成论文摘要