Exploring the Versatility of Zero-Shot CLIP for Interstitial Lung Disease Classification
CoRR(2023)
摘要
Interstitial lung diseases (ILD) present diagnostic challenges due to their varied manifestations and overlapping imaging features. To address this, we propose a machine learning approach that utilizes CLIP, a multimodal (image and text) self-supervised model, for ILD classification. We extensively integrate zero-shot CLIP throughout our workflow, starting from the initial extraction of image patches from volumetric CT scans and proceeding to ILD classification using "patch montages". Furthermore, we investigate how domain adaptive pretraining (DAPT) CLIP with task-specific images (CT "patch montages" extracted with ILD-specific prompts for CLIP) and/or text (lung-specific sections of radiology reports) affects downstream ILD classification performance. By leveraging CLIP-extracted "patch montages" and DAPT, we achieve strong zero-shot ILD classification results, including an AUROC of 0.893, without the need for any labeled training data. This work highlights the versatility and potential of multimodal models like CLIP for medical image classification tasks where labeled data is scarce.
更多查看译文
关键词
interstitial lung
AI 理解论文
溯源树
样例
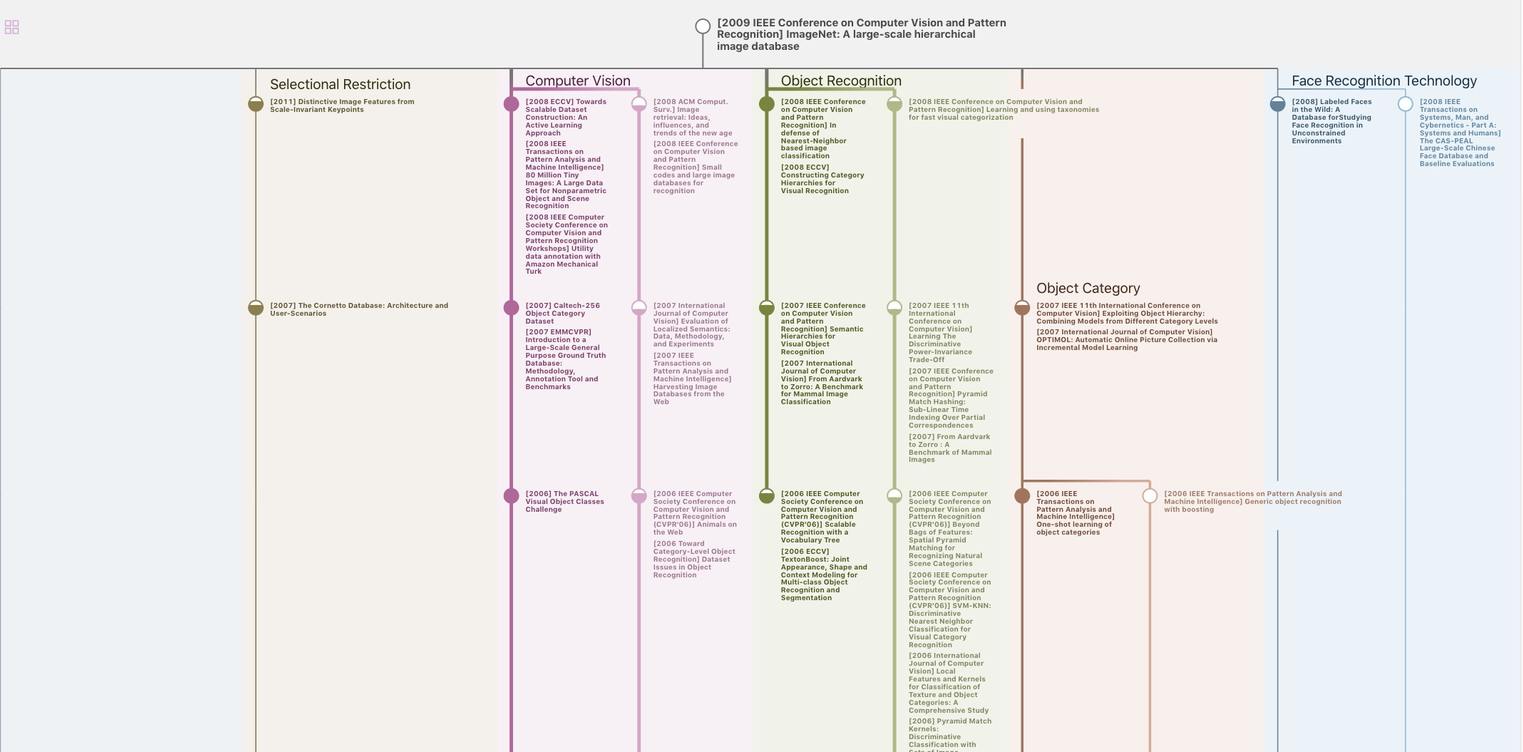
生成溯源树,研究论文发展脉络
Chat Paper
正在生成论文摘要