ISOP: Machine Learning-Assisted Inverse Stack-Up Optimization for Advanced Package Design
2023 DESIGN, AUTOMATION & TEST IN EUROPE CONFERENCE & EXHIBITION, DATE(2023)
摘要
Future computing calls for heterogeneous integration, e.g., the recent adoption of the chiplet methodology. However, high-speed cross-chip interconnects and packaging shall be critical for the overall system performance. As an example of advanced packaging, a high-density interconnect (HDI) printed circuit board (PCB) has been widely used in complex electronics from cell phones to computing servers. A modern HDI PCB may have over 20 layers, each with its unique material properties and geometrical dimensions, i.e., stack-up, to meet various design constraints and performance optimizations. However, stackup design is usually done manually in the industry, where experienced designers may devote many hours to adjusting the physical dimensions and materials to meet the desired specifications. This process, however, is time-consuming, tedious, and sub-optimal, largely depending on the designer's expertise. In this paper, we propose to automate the stackup design with a new framework, ISOP, using machine learning for inverse stack-up optimization for advanced package design. Given a target design specification, ISOP automatically searches for ideal stack-up design parameters while optimizing performance. We develop a novel machine learning-assisted hyper-parameter optimization method to make the search efficient and reliable. Experimental results demonstrate that ISOP is better in figure-of-merit (FoM) than conventional simulated annealing and Bayesian optimization algorithms, with all our design targets met with a shorter runtime. We also compare our fully-automated ISOP with expert designers in the industry and achieve very promising results, with orders of magnitude reduction of turn-around time.
更多查看译文
AI 理解论文
溯源树
样例
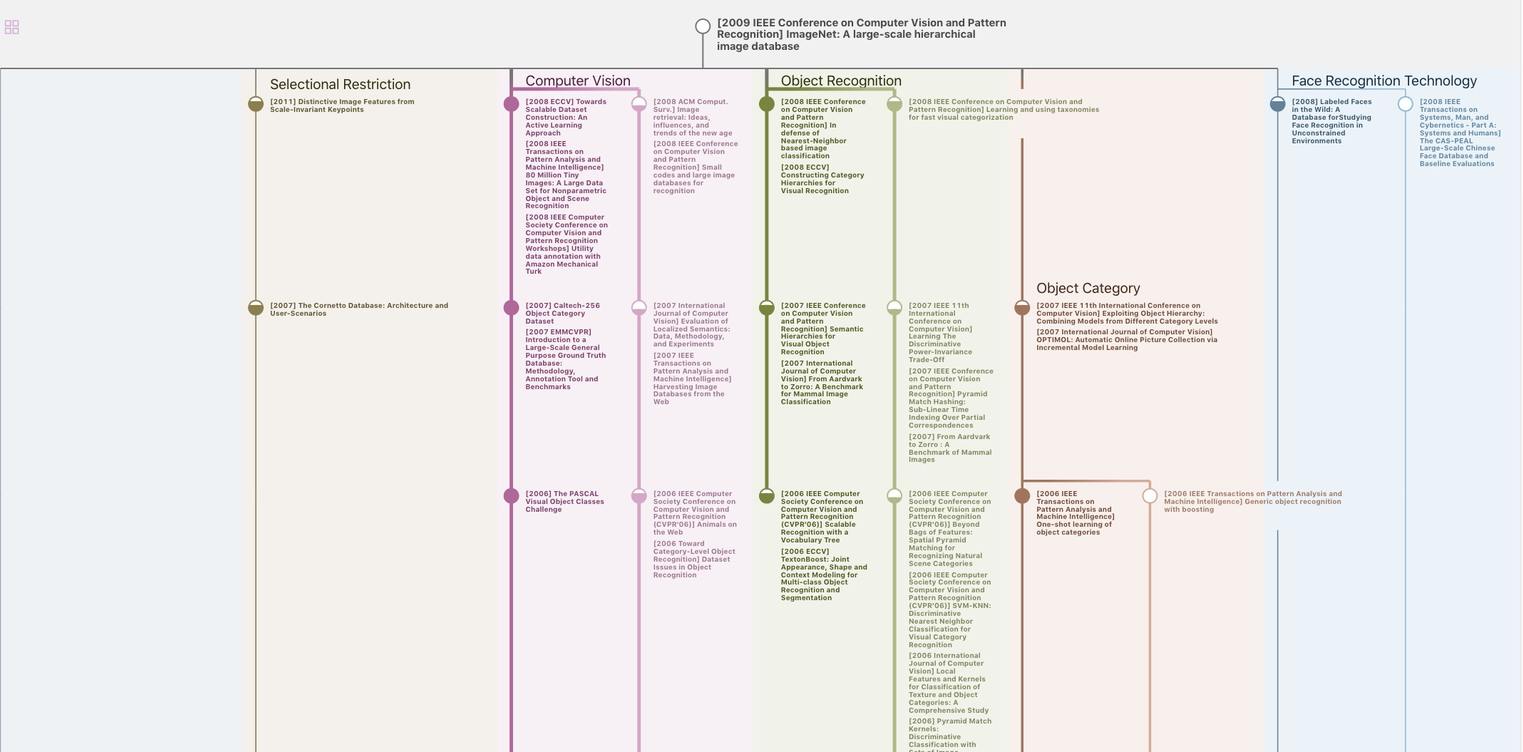
生成溯源树,研究论文发展脉络
Chat Paper
正在生成论文摘要