Programmable Tanh-, ELU-, Sigmoid-, and Sin-Based Nonlinear Activation Functions for Neuromorphic Photonics
IEEE Journal of Selected Topics in Quantum Electronics(2023)
Abstract
We demonstrate a programmable analog opto-electronic (OE) circuit that can be configured to provide a range of nonlinear activation functions for incoherent neuromorphic photonic circuits at up to 10 Gbaud line-rates. We present a set of well-known activation functions that are typically used to train DL models including tanh-, sigmoid-, ReLU- and inverted ReLU-like activations, introducing also a series of novel photonic nonlinear functions that are referred to as Rectified Sine Squared (ReSin), Sine Squared with Exponential tail (ExpSin) and Double Sine Squared. Experimental validation for all these activation functions is performed at 10 Gbaud operation. The ability of the mathematically modelled photonic activation functions to train Deep Neural Networks (DNNs) has been verified through their employment in Deep Learning (DL) models for MNIST and CIFAR10 classification purposes, comparing their performance against corresponding NNs that utilize an ideal ReLU activation function.
MoreTranslated text
Key words
Nonlinear activation function,opto-electronic activation function,opto-electro-optic activation function,programmable analog device
AI Read Science
Must-Reading Tree
Example
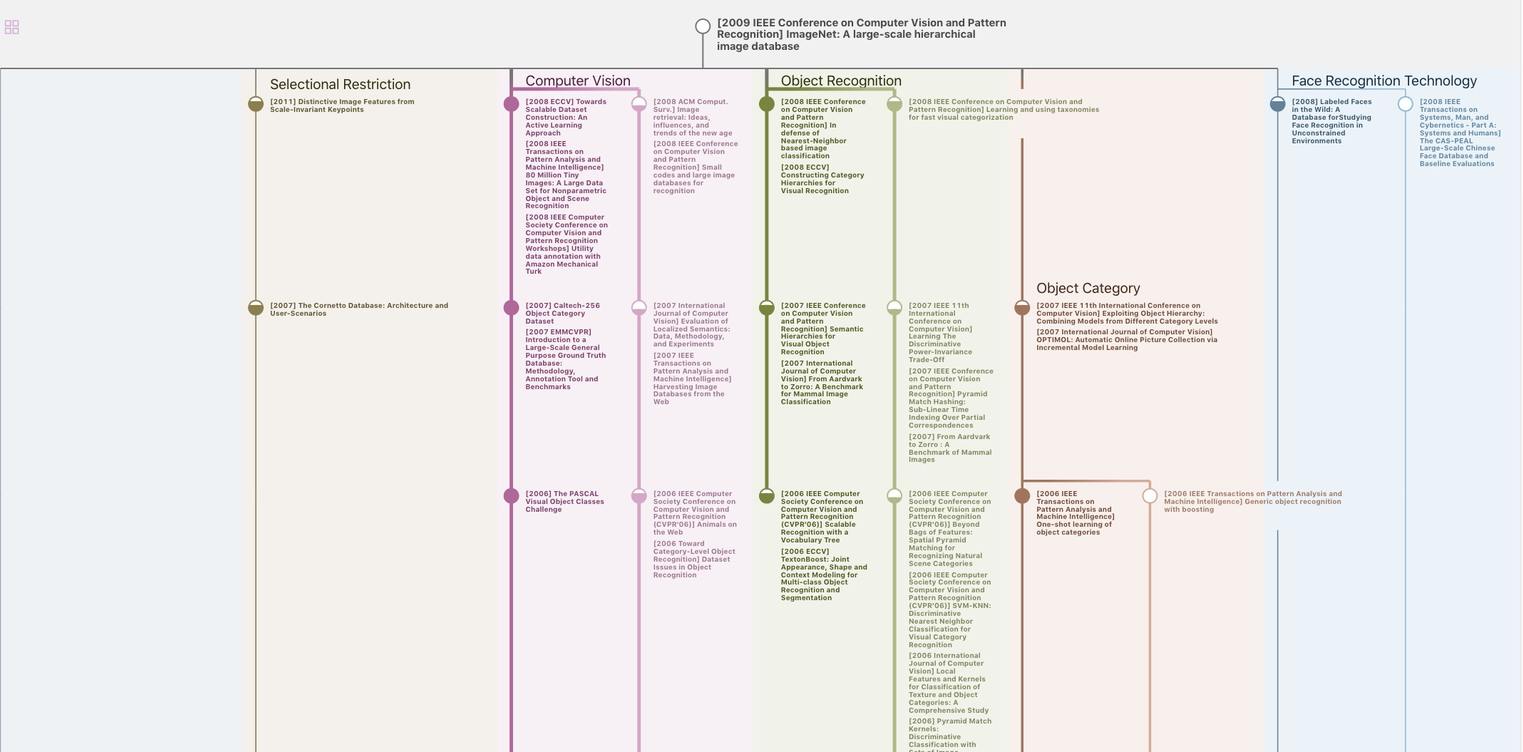
Generate MRT to find the research sequence of this paper
Chat Paper
Summary is being generated by the instructions you defined