Meta adversarial learning improves low-resource speech recognition
COMPUTER SPEECH AND LANGUAGE(2024)
Abstract
Low-resource automatic speech recognition is a challenging task. To resolve this issue, multilin-gual meta-learning learns a better model initialization from many source languages, allowing for rapid adaption to target languages. However, differences in data scales and learning difficulties vary greatly from one language to another. As a result, the model favors large-scale and simple source languages. Moreover, the shared semantic space of various languages is difficult to learn due to a lack of restrictions on multilingual pre-training. In this paper, we propose a meta adversarial learning approach to address this problem. The meta-learner will be guided to learn language-independent information by using an adversarial auxiliary objective of language identification, which makes the shared semantic space more compact and improves model generalization. Additionally, we optimize adversarial training using Wasserstein distance and temporal normalization, enabling more stable and simple training. Experiment results on IARPA BABEL and OpenSLR show a significant performance improvement. It also outperforms state-of-the-art results by a large margin in all target languages, and especially in few-shot settings. Finally, we demonstrate how our method is superior by using t-SNE visualization.
MoreTranslated text
Key words
Adversarial training,Meta learning,Low-resource,Speech recognition,IARPA-BABEL,OpenSLR
AI Read Science
Must-Reading Tree
Example
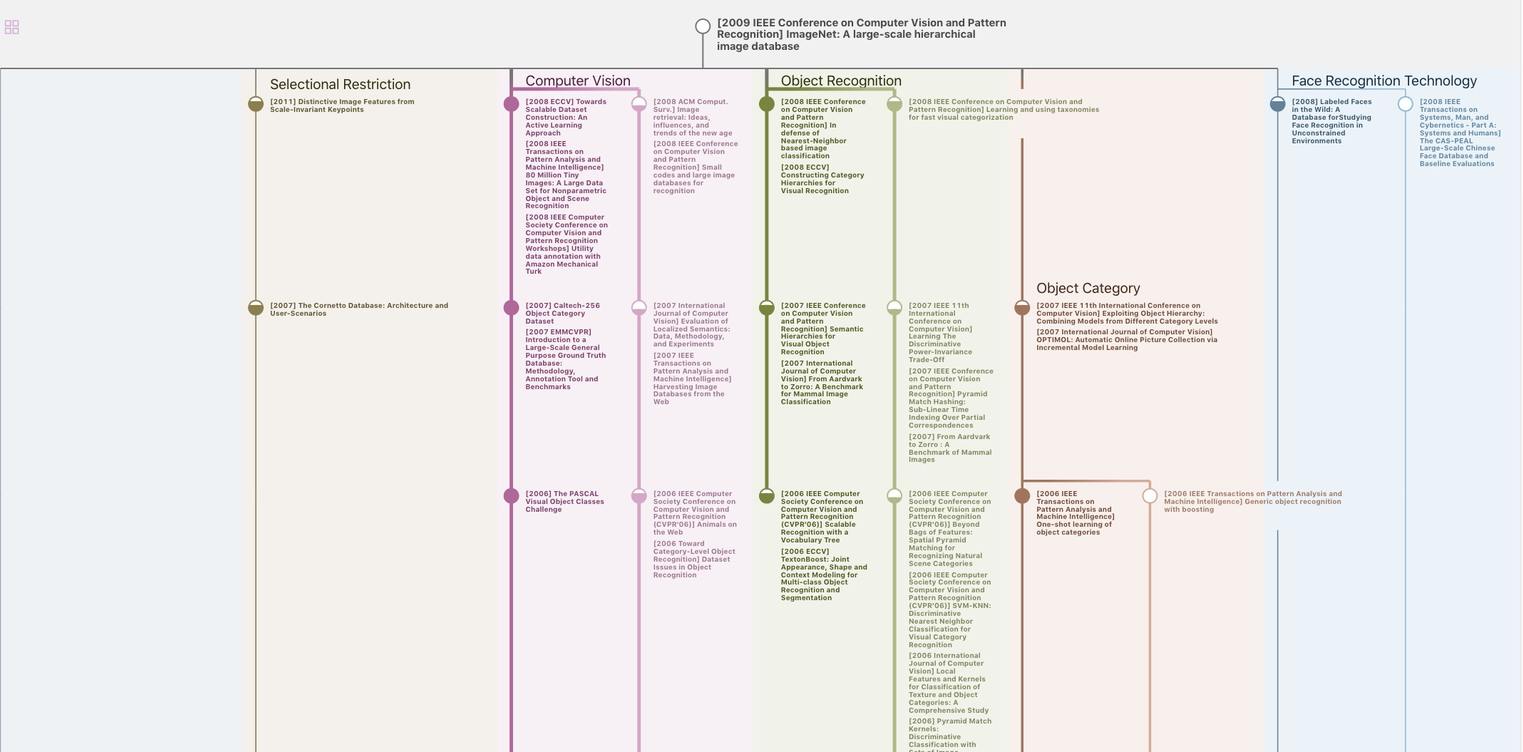
Generate MRT to find the research sequence of this paper
Chat Paper
Summary is being generated by the instructions you defined