Machine Learning for Intrusion Detection: Stream Classification Guided by Clustering for Sustainable Security in IoT
GLSVLSI '23: Proceedings of the Great Lakes Symposium on VLSI 2023(2023)
摘要
The Internet of Things (IoT) has brought about unprecedented connectivity and convenience in our daily lives, but with this newfound interconnectedness comes the threat of cyber-attacks. With ever-increasing IoT devices being connected to the internet, securing IoT devices is becoming increasingly urgent. Machine learning (ML) is among the most popular techniques used by intrusion detection systems (IDS) to enhance their detection performance when securing IoT. However, a key obstacle of ML-based IDS for IoT is learning from nonstationary streaming data, also known as concept drift. One of the most challenging learning scenarios under concept drift is extreme verification latency (EVL), which occurs when only unlabeled nonstationary streaming data is available after a small set of initial labeled data. Stream Classification Algorithm Guided by Clustering (SCARGC) is an algorithm that can effectively deal with the nonstationary data streams in EVL scenarios. Applying an EVL implementation provides the capability of adapting to nonstationary environments within the IoT domain. The SCARGC model, as an integrated IoT intrusion detection system, allows for sustainable security as new threats are identified in this non-stationary environment. Hence, in this project, we develop an innovative IoT intrusion detection approach by natively integrating SCARGC and intrusion detection to address the EVL challenges to provide sustainable security as the model adapts to nonstationary environments. We evaluated the proposed approach on real-world IoT cybersecurity datasets. The results demonstrate the feasibility of the proposed approach, which can lead to the development of sophisticated intrusion detection systems for IoT.
更多查看译文
关键词
IoT Security, Extreme Verification Latency, Nonstationary Environments, Datastream, Machine Learning for Security, Intrusion Detection Systems
AI 理解论文
溯源树
样例
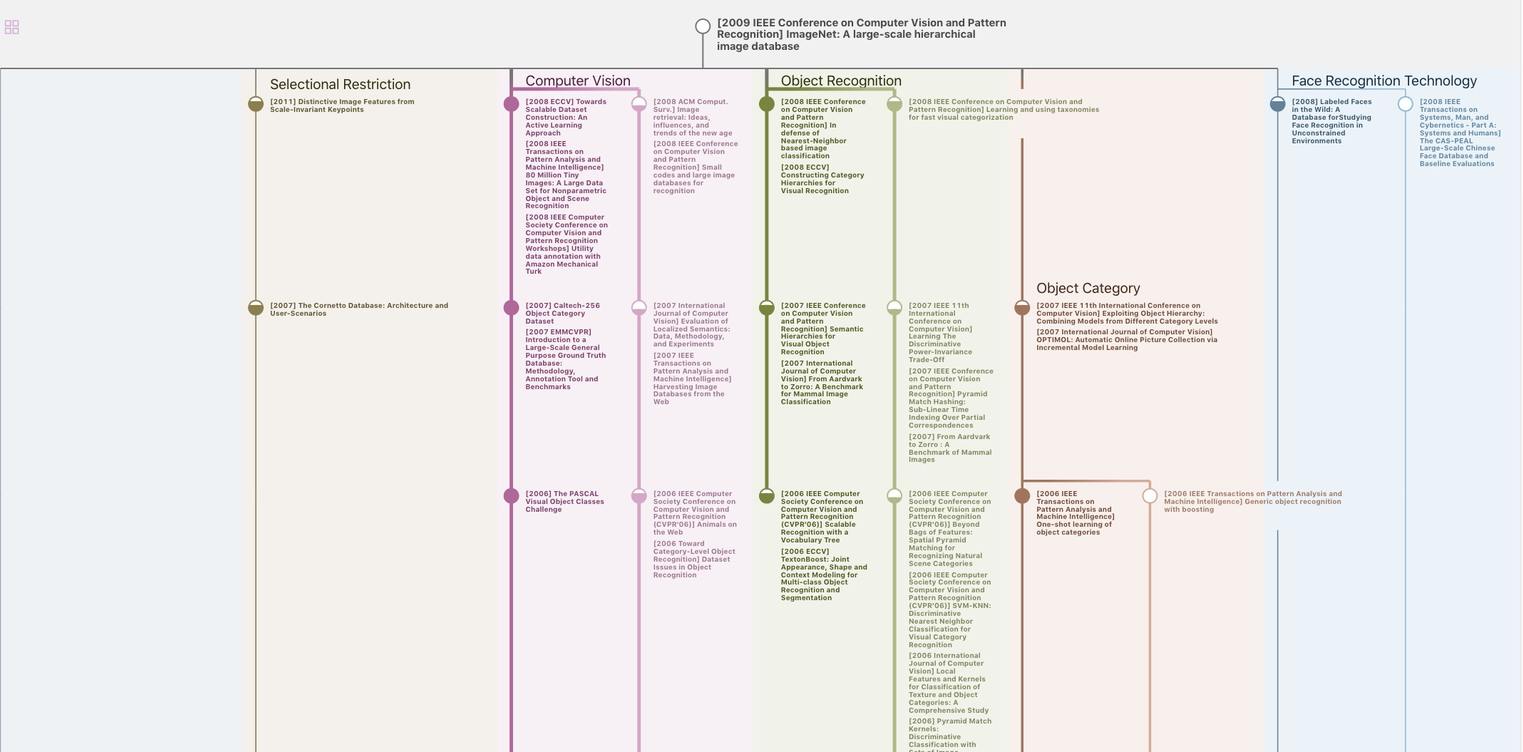
生成溯源树,研究论文发展脉络
Chat Paper
正在生成论文摘要