SMAC-tuned Deep Q-learning for Ramp Metering.
SM(2023)
摘要
The demand for transportation increases as the population of a city grows, and significant expansion is not conceivable because of spatial, financial, and environmental limitations. As a result, improving infrastructure efficiency is becoming increasingly critical. Ramp metering with deep reinforcement learning (RL) is a method to tackle this problem. However, fine-tuning RL hyperparameters for RM is yet to be explored in the literature, potentially leaving performance improvements on the table. In this paper, the Sequential Model-based Algorithm Configuration (SMAC) method is used to finetune the values of two essential hyperparameters for the deep reinforcement learning ramp metering model, the discount factor and the decay of the explore/exploit ratio. Around 350 experiments with different configurations were run with PySMAC (a python interface to the hyperparameter optimization tool SMAC) and compared to Random search as a baseline. It is found that the best reward discount factor reflects that the RL agent should focus on immediate rewards and not pay much attention to future rewards. On the other hand, the selected value for the exploration ratio decay rate shows that the RL agent should prefer to decrease the exploration rate early. Both random search and SMAC show the same performance improvement of 19
更多查看译文
关键词
Ramp metering, Reinforcement learning, Hyperparameter tuning
AI 理解论文
溯源树
样例
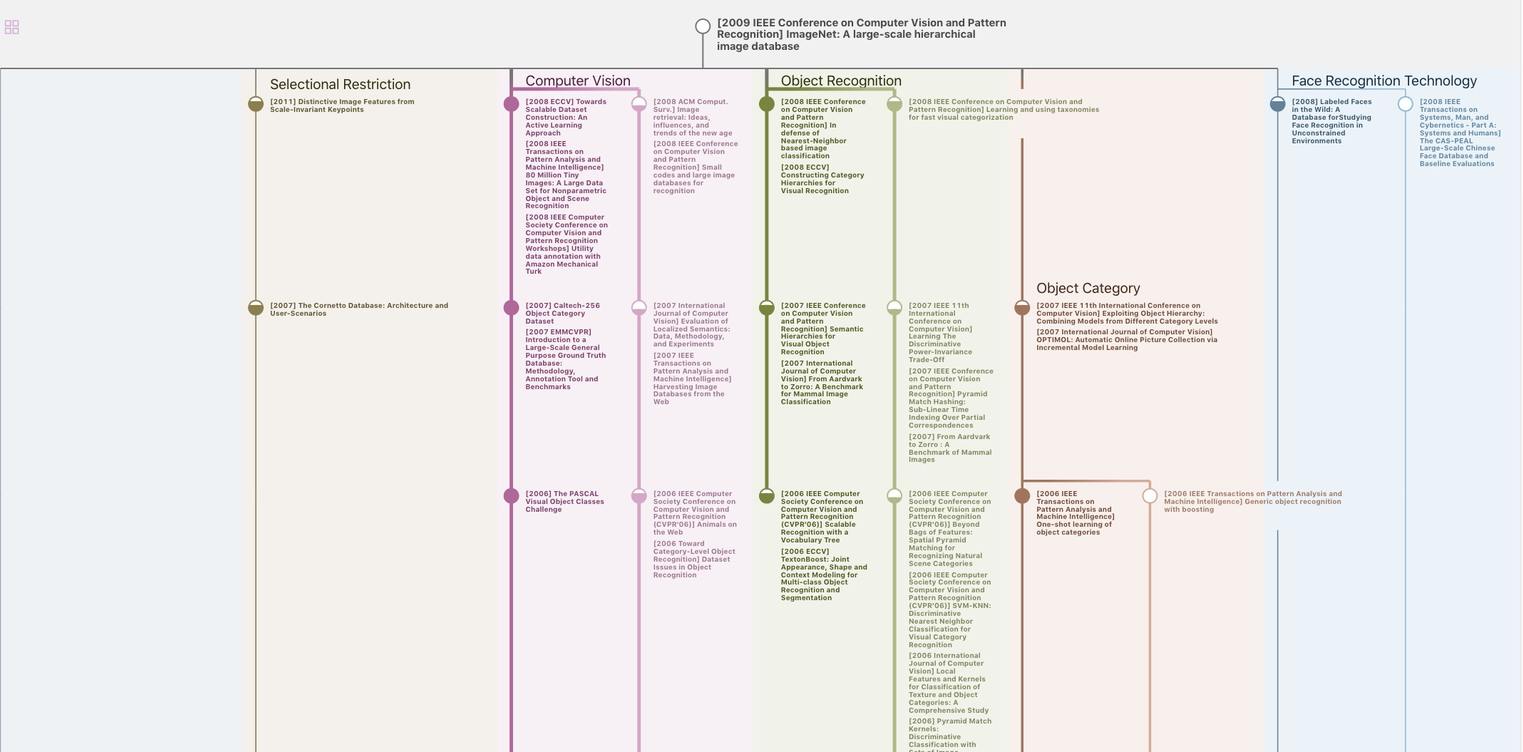
生成溯源树,研究论文发展脉络
Chat Paper
正在生成论文摘要