Generation-Based Data Augmentation for Offensive Language Detection: Is It Worth It?
17TH CONFERENCE OF THE EUROPEAN CHAPTER OF THE ASSOCIATION FOR COMPUTATIONAL LINGUISTICS, EACL 2023(2023)
摘要
been presented in several works as a way to improve offensive language detection. However, the effectiveness of generative DA has been shown only in limited scenarios, and the potential injection of biases when using generated data to classify offensive language has not been investigated. Our aim is that of analyzing the feasibility of generative data augmentation more in-depth with two main focuses. First, we investigate the robustness of models trained on generated data in a variety of data augmentation setups, both novel and already presented in previous work, and compare their performance on four widely-used English offensive language datasets that present inherent differences in terms of content and complexity. In addition to this, we analyze models using the HateCheck suite, a series of functional tests created to challenge hate speech detection systems. Second, we investigate potential lexical bias issues through a qualitative analysis of the generated data. We find that the potential positive impact of generative data augmentation on model performance is unreliable, and generative DA can also have unpredictable effects on lexical bias.
更多查看译文
AI 理解论文
溯源树
样例
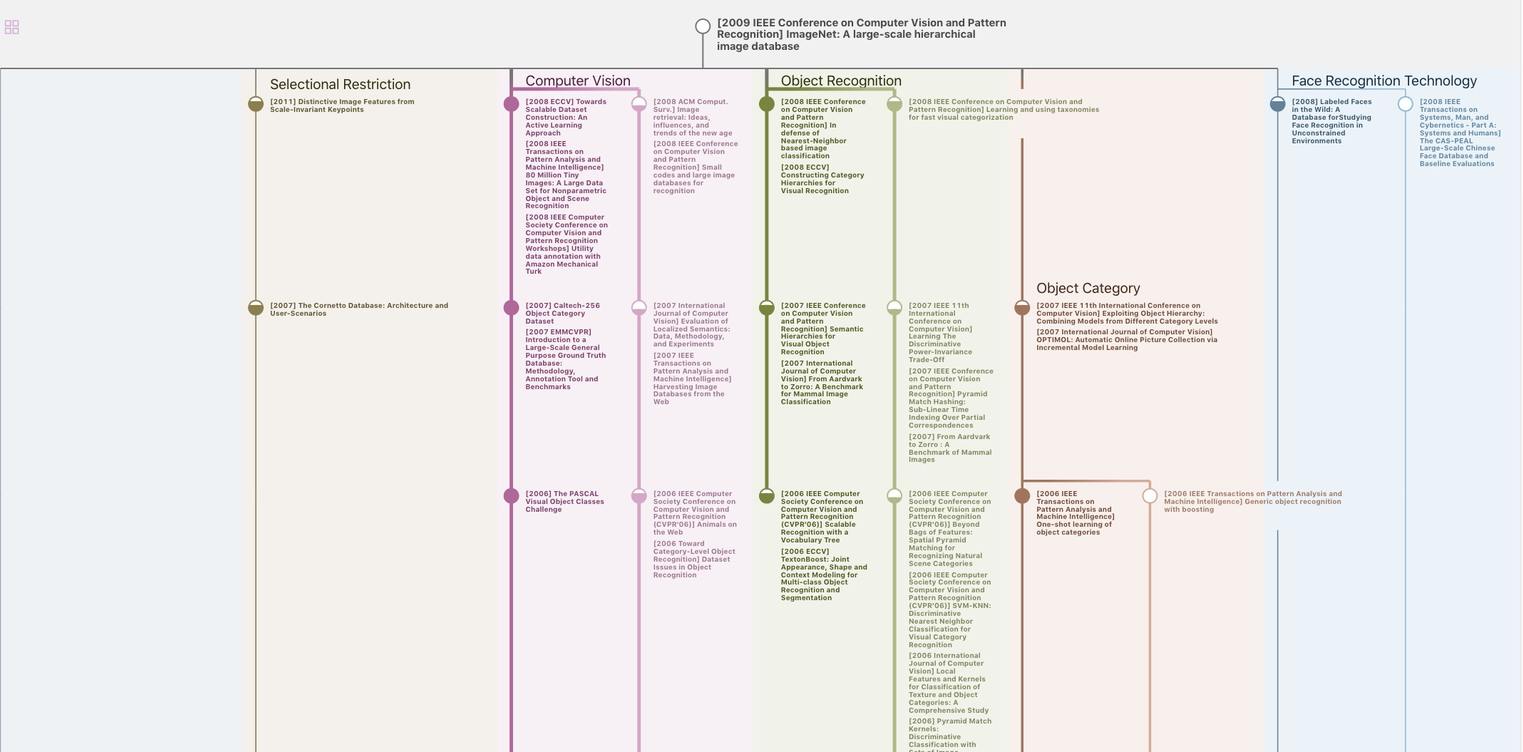
生成溯源树,研究论文发展脉络
Chat Paper
正在生成论文摘要