FedPerC: Federated Learning for Language Generation with Personal and Context Preference Embeddings
17TH CONFERENCE OF THE EUROPEAN CHAPTER OF THE ASSOCIATION FOR COMPUTATIONAL LINGUISTICS, EACL 2023(2023)
Abstract
Federated learning is a training paradigm that learns from multiple distributed users without aggregating data on a centralized server, promising the ability to deploy machine-learning to a diverse population of users without first collecting large, labeled datasets. As federated learning involves averaging gradient updates across a decentralized population, there is a growing need for personalization of federated learning systems (i.e. conversational agents must personalize to individual users and the context of an interaction). In this work, we propose a new direction for personalization research within federated learning, leveraging both personal embeddings and shared context embeddings. We also present an approach to predict these "preference" embeddings, enabling personalization without back-propagation. Compared to state-of-the-art personalization baselines, our approach achieves a 50% improvement in test-time perplexity using 0.001% of the memory required by baseline approaches, and achieving greater sample- and compute-efficiency.
MoreTranslated text
AI Read Science
Must-Reading Tree
Example
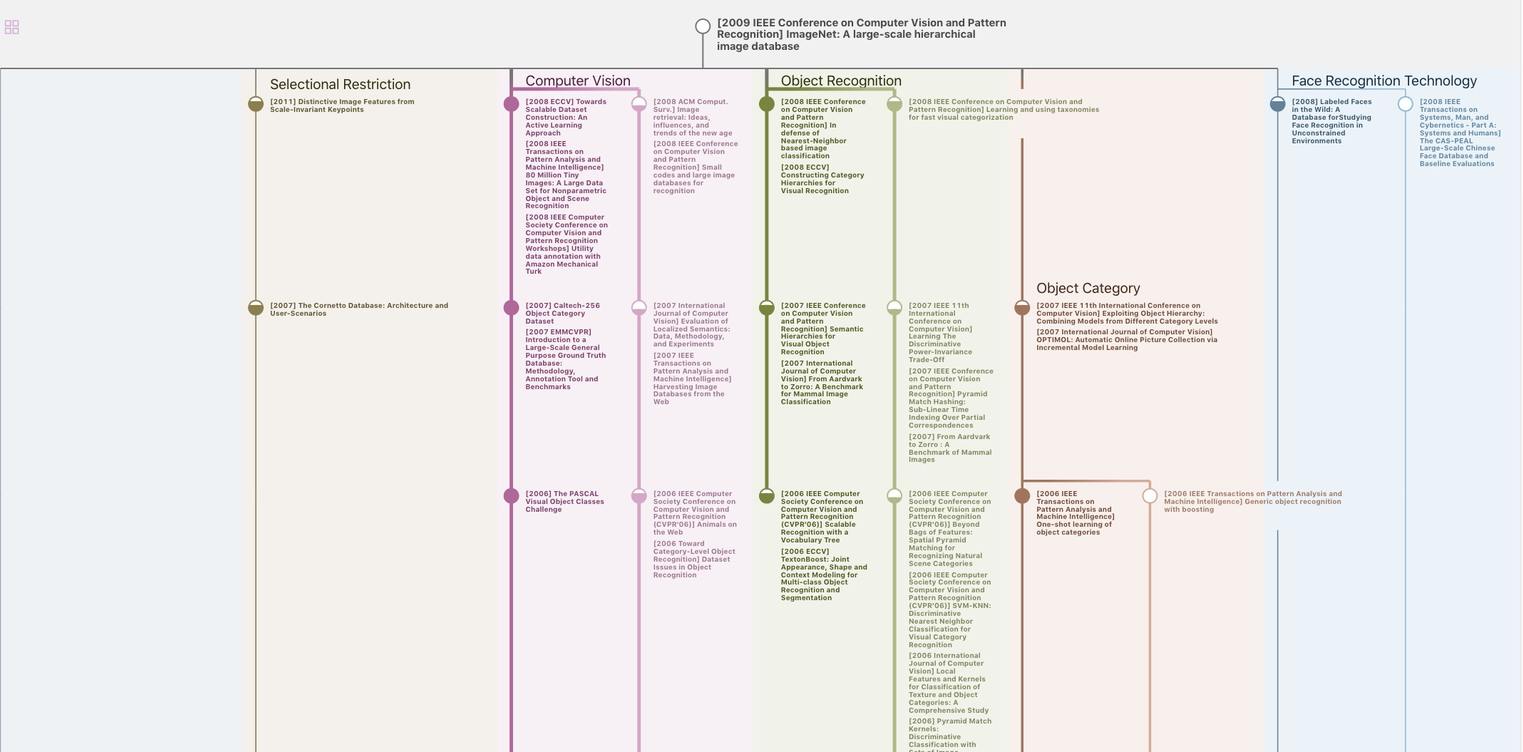
Generate MRT to find the research sequence of this paper
Chat Paper
Summary is being generated by the instructions you defined