Probabilistic Robustness for Data Filtering
17TH CONFERENCE OF THE EUROPEAN CHAPTER OF THE ASSOCIATION FOR COMPUTATIONAL LINGUISTICS, EACL 2023(2023)
摘要
We introduce our probabilistic robustness rewarded data optimization (PRoDO) approach as a framework to enhance the model's generalization power by selecting training data that optimizes our probabilistic robustness metrics. We use proximal policy optimization (PPO) reinforcement learning to approximately solve the computationally intractable training subset selection problem. The PPO's reward is defined as our (alpha,epsilon,gamma)-Robustness that measures performance consistency over multiple domains by simulating unknown test sets in real-world scenarios using a leaving-one-out strategy. We demonstrate that our PRoDO effectively filters data that lead to significantly higher prediction accuracy and robustness on unknown-domain test sets. Our experiments achieve up to +17.2% increase of accuracy (+25.5% relatively) in sentiment analysis, and 28.05 decrease of perplexity (-32.1% relatively) in language modeling. In addition, our probabilistic (alpha,epsilon,gamma)-Robustness definition serves as an evaluation metric with higher levels of agreement with human annotations than typical performance-based metrics.
更多查看译文
AI 理解论文
溯源树
样例
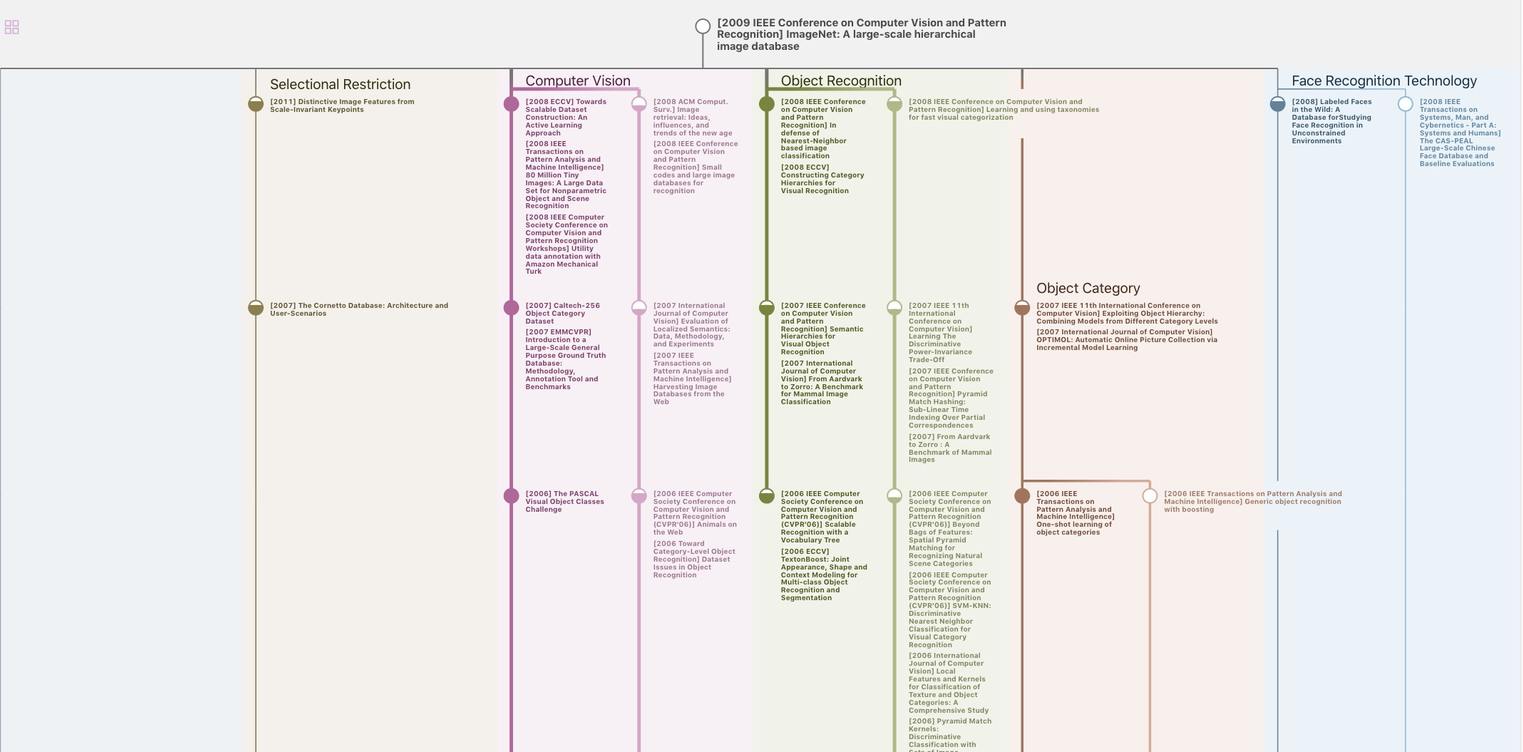
生成溯源树,研究论文发展脉络
Chat Paper
正在生成论文摘要