Deep Hybrid Neural Network With Attention Mechanism for Video Hash Retrieval Method.
IEEE Access(2023)
摘要
With the advent of 5G technology, a large amount of video data will be generated and uploaded to the Internet every day, which makes it an urgent problem to effectively retrieve similar video content from massive video databases. However, most of the existing video retrieval methods usually ignore the time information contained in the video, which causes the problem that the temporal information feature is not obvious when extracting video features. Therefore, this paper proposes a deep hybrid neural network with attention mechanism for video hash retrieval method. The method first feeds the extracted video key frames into the residual network embedded with the attention mechanism and the bidirectional gated recurrent unit network to extract the spatio-temporal information features of the video, so as to improve the representation ability of the features, and then integrates the hash learning technology into the network framework to generate the binary hash code of the video to shorten the video retrieval time. By designing objective function to capture the information of the global data structure using the similarity relationship between codes, the class label information can be effectively used to improve the retrieval performance. Compared with the existing methods, the experimental results show that the method has a certain improvement in the retrieval accuracy on the commonly used video datasets UCF-101 and JHMDB, which verififies the feasibility of the method.
更多查看译文
关键词
Feature extraction, Neural networks, Data mining, Encoding, Visualization, Convolutional neural networks, Binary codes, Video retrieval, attention mechanism, residual network, bidirectional gated recurrent unit network, hash learning
AI 理解论文
溯源树
样例
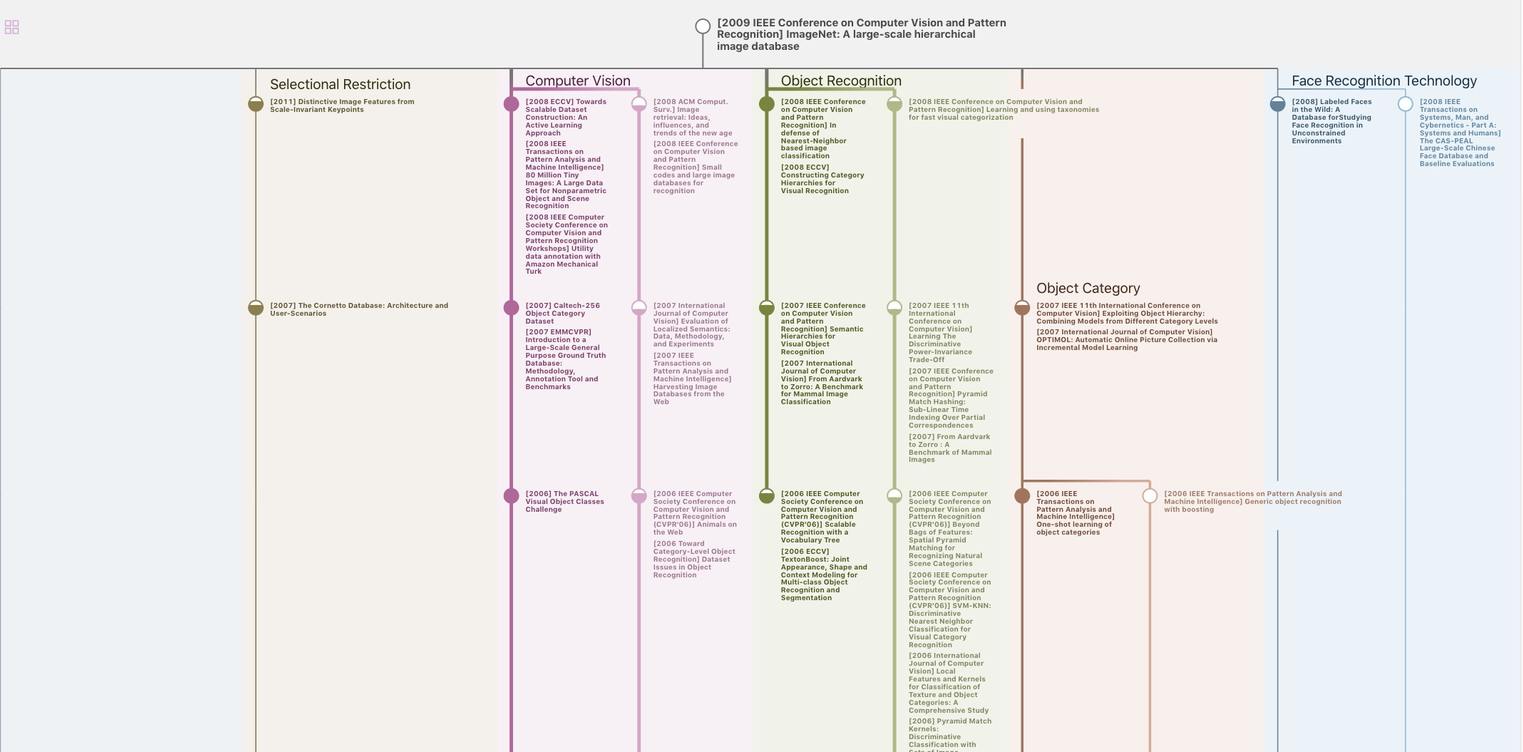
生成溯源树,研究论文发展脉络
Chat Paper
正在生成论文摘要