UNCER: A framework for uncertainty estimation and reduction in neural decoding of EEG signals.
Neurocomputing(2023)
摘要
EEG decoding systems based on deep neural networks have been widely used in the decision-making of brain-computer interfaces (BCI). Their predictions, however, can be unreliable given the significant variance and noise in EEG signals. Previous works on EEG analysis mainly focus on the exploration of noise patterns in the source signal, while the uncertainty during the decoding process is largely unexplored. Automatically detecting and reducing such decoding uncertainty is important for BCI motor imagery applications such as robotic arm control etc. In this work, we proposed an uncertainty estimation and reduction model (UNCER) to quantify and mitigate the uncertainty during the EEG decoding process. It utilized a combination of dropout oriented method and Bayesian neural network for uncertainty estimation to incorporate both the uncertainty in the input signal and the uncertainty in the model parameters. We further proposed an adaptive data augmentation-based approach for uncertainty reduction. The model can be integrated into the current widely used EEG neural decoders without change of the architecture. We performed extensive experiments for uncertainty estimation and its reduction in both intra-subject EEG decoding and cross-subject EEG decoding on two public motor imagery datasets, where the proposed model achieves significant improvement both in the quality of estimated uncertainty and the effectiveness of uncertainty reduction.
更多查看译文
关键词
EEG decoding,Uncertainty estimation and reduction,Brain computer interfaces
AI 理解论文
溯源树
样例
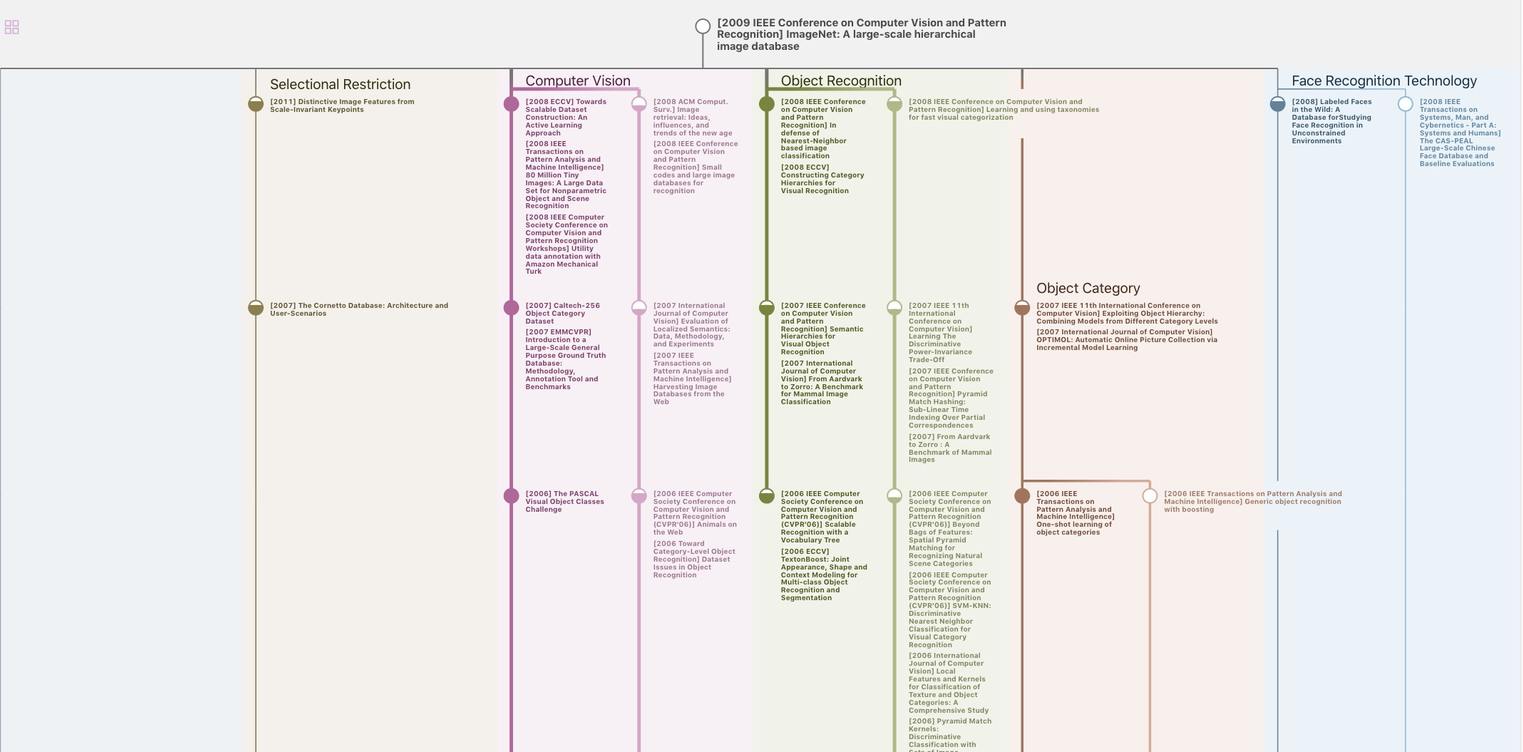
生成溯源树,研究论文发展脉络
Chat Paper
正在生成论文摘要