Balanced image captioning with task-aware decoupled learning and fusion.
Neurocomputing(2023)
Abstract
Image captioning aims to generate natural language descriptions for images. Word occurrences usually obey Zipf’s Law, the imbalance phenomenon makes the conventional training bias to majority data. However, this imbalance distribution has not been considered adequately in captioning works. In this paper, we match the imbalance learning methods in classification with image captioning, making the empirical study. We also propose a Task-aware Decoupled Learning and Fusion (TDLF) approach, which outperforms the former. Image captioning differs from classification in three main aspects: 1) captions are sequential labels that exist co-occurrence, 2) the generation methods usually follow the autoregressive manner, 3) the imbalance ratio is extremely large. To deal with these problems, our TDLF method introduces multi-task learning into the re-balancing approach. The model is composed of a shared autoregressor and two task classifiers, i.e., a conventional training classifier, and a balance-training classifier. The model is further equipped with a task-aware decoupling strategy, we propose the Task Perception Indication (TPI) to measure whether the conventional training is shifted. The balance-training classifier is trained by the biased data separately and the generations of two tasks are fused according to the TPI. Experiments on the MSCOCO database show that our model outperforms the state-of-the-art methods on generation accuracy and word diversity, demonstrating the effectiveness of the proposed method.
MoreTranslated text
Key words
Vision -and -language, Image captioning, Imbalance learning, Multi -task learning
AI Read Science
Must-Reading Tree
Example
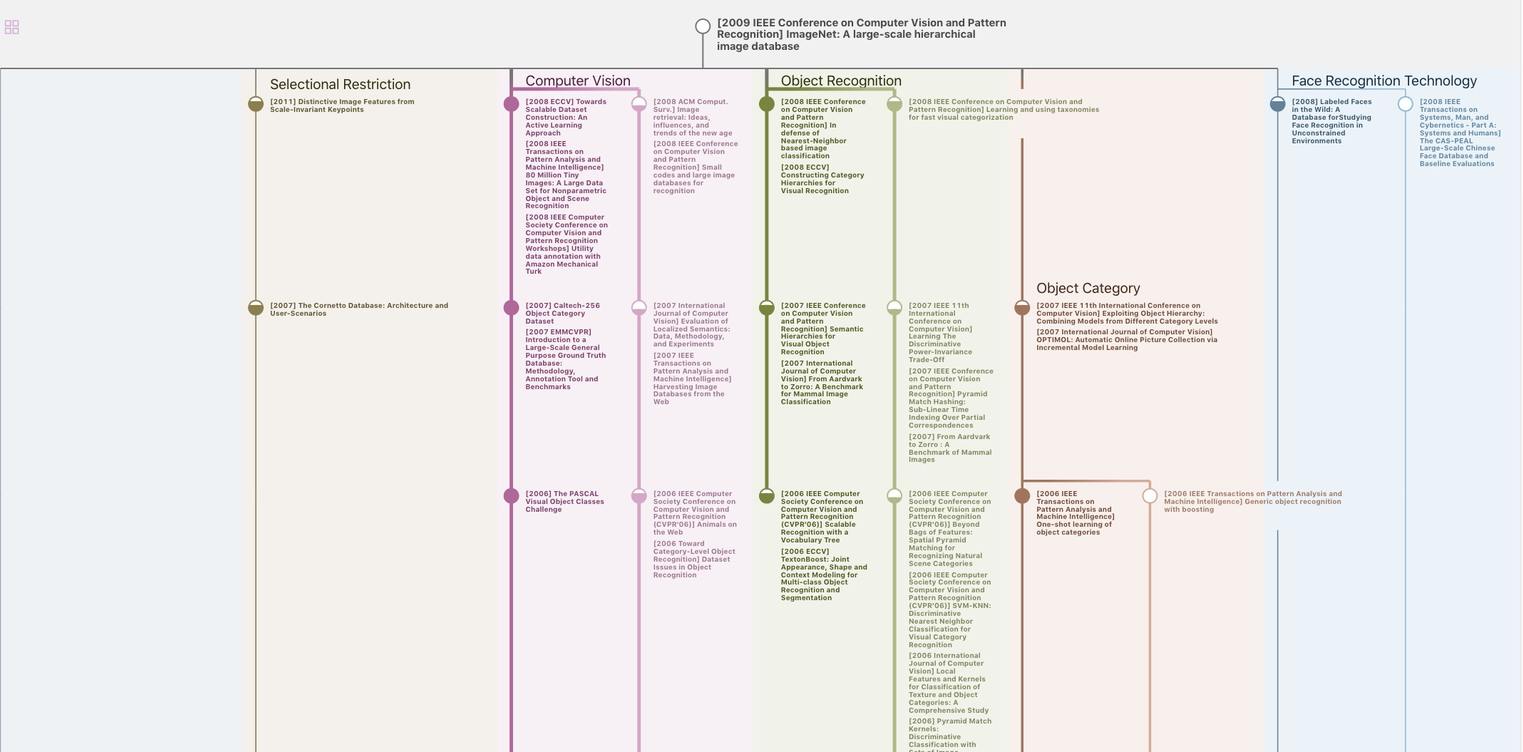
Generate MRT to find the research sequence of this paper
Chat Paper
Summary is being generated by the instructions you defined