Relational Action Bank with Semantic-Visual Attention for Few-Shot Action Recognition.
Future Internet(2023)
摘要
Recently, few-shot learning has attracted significant attention in the field of video action recognition, owing to its data-efficient learning paradigm. Despite the encouraging progress, identifying ways to further improve the few-shot learning performance by exploring additional or auxiliary information for video action recognition remains an ongoing challenge. To address this problem, in this paper we make the first attempt to propose a relational action bank with semantic–visual attention for few-shot action recognition. Specifically, we introduce a relational action bank as the auxiliary library to assist the network in understanding the actions in novel classes. Meanwhile, the semantic–visual attention is devised to adaptively capture the connections to the foregone actions via both semantic correlation and visual similarity. We extensively evaluate our approach via two backbone models (ResNet-50 and C3D) on HMDB and Kinetics datasets, and demonstrate that the proposed model can obtain significantly better performance compared against state-of-the-art methods. Notably, our results demonstrate an average improvement of about 6.2% when compared to the second-best method on the Kinetics dataset.
更多查看译文
关键词
semantic attention,visual attention,relational action bank,few-shot learning,action recognition
AI 理解论文
溯源树
样例
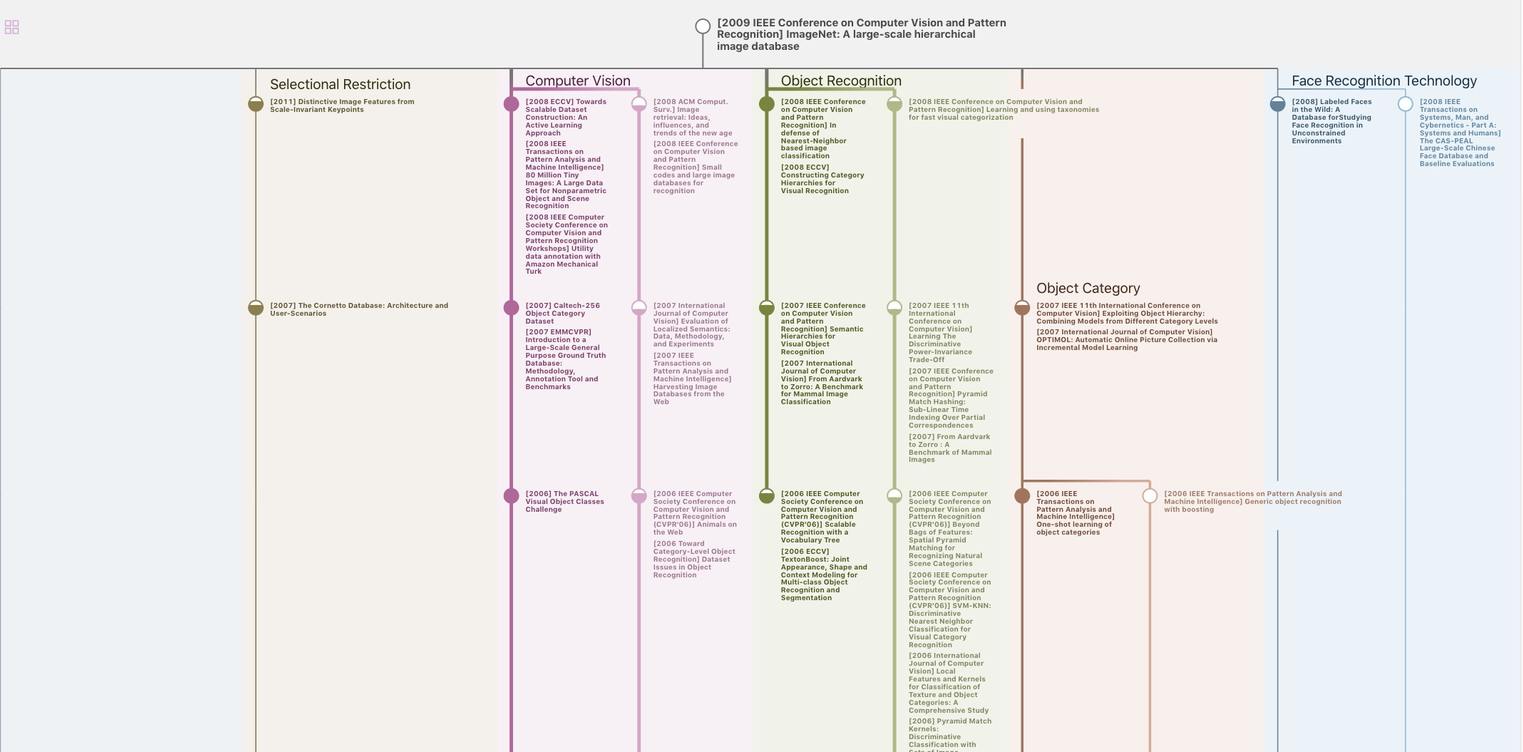
生成溯源树,研究论文发展脉络
Chat Paper
正在生成论文摘要