Stochastic perturbation of subgradient algorithm for nonconvex deep neural networks
Comput. Appl. Math.(2023)
摘要
Choosing a learning rate is a necessary part of any subgradient method optimization. With deeper models such as convolutional neural networks of image classification, fine-tuning the learning rate can quickly become tedious, and it does not always result in optimal convergence. In this work, we suggest a variation of the subgradient method in which the learning rate is updated by a control step in each iteration of each epoch. Stochastic Perturbation Subgradient Algorithm (SPSA) is our approach for tackling image classification issues with deep neural networks including convolutional neural networks. Used MNIST dataset, the numerical results reveal that our SPSA method is faster than Stochastic Gradient Descent and its variants with a fixed learning rate. However SPSA and convolutional neural network model improve the results of image classification including loss and accuracy.
更多查看译文
关键词
subgradient algorithm,stochastic perturbation,neural networks,nonconvex
AI 理解论文
溯源树
样例
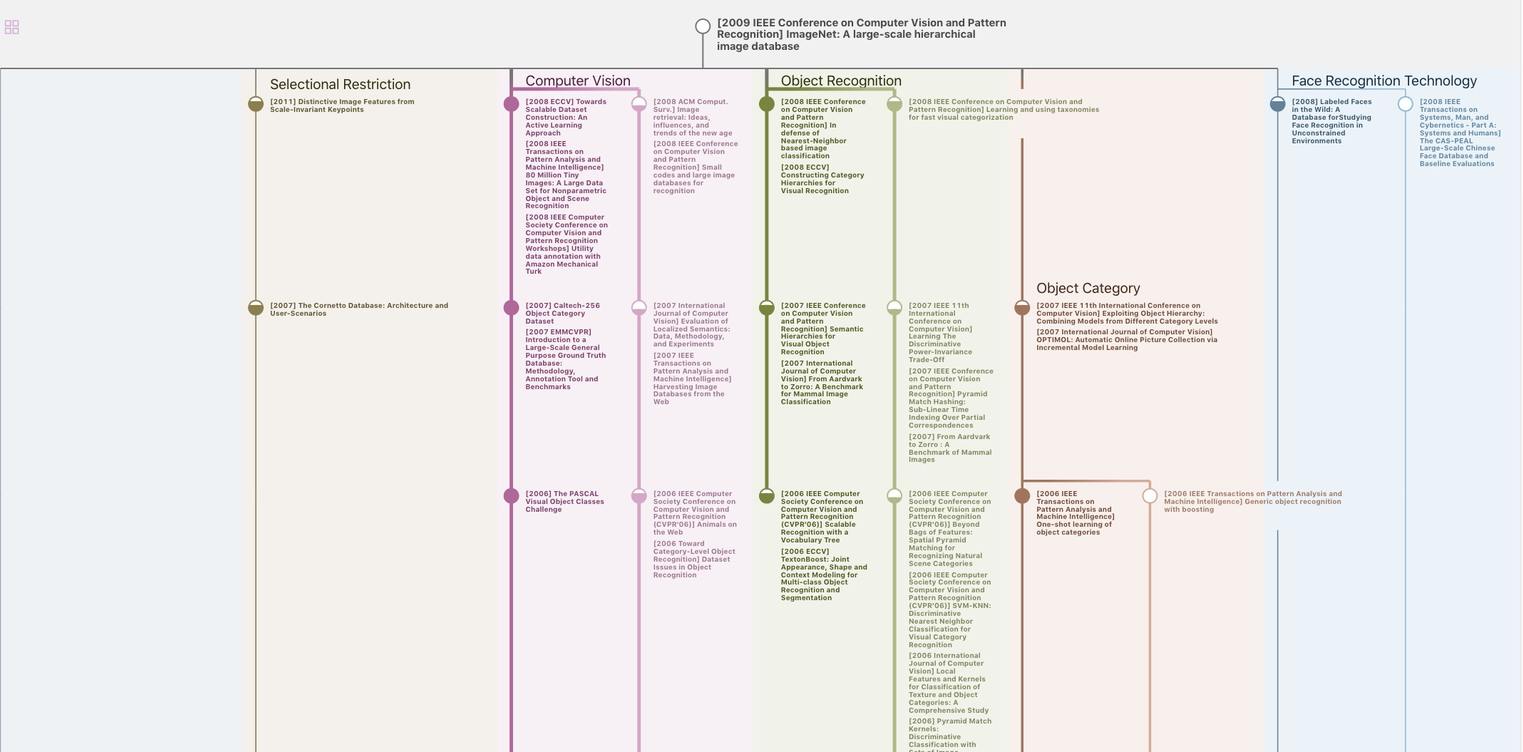
生成溯源树,研究论文发展脉络
Chat Paper
正在生成论文摘要