Towards Poisoning of Federated Support Vector Machines with Data Poisoning Attacks
PROCEEDINGS OF THE 13TH INTERNATIONAL CONFERENCE ON CLOUD COMPUTING AND SERVICES SCIENCE, CLOSER 2023(2023)
Abstract
Federated Support Vector Machine (F-SVM) is a technology that enables distributed edge devices to collectively learn a common SVM model without sharing data samples. Instead, edge devices submit local updates to the global machine, which are then aggregated and sent back to edge devices. Due to the distributed nature of federated learning, edge devices are vulnerable to poisoning attacks, especially during training. Attackers in adversarial edge devices can poison the dataset to hamper the global machine's accuracy. This study investigates the impact of data poisoning attacks on federated SVM classifiers. In particular, we adopt two widespread data poisoning attacks for SVM named label flipping and optimal poisoning attacks for F-SVM and evaluate their impact on the MNIST and CIFAR10 datasets. We measure the impact of these poisoning attacks on the precision of global training. Results show that 33% of adversarial edge devices can reduce accuracy up to 30%. Furthermore, we also investigate some basic defense strategies against poisoning attacks on federated SVM.
MoreTranslated text
Key words
Support Vector Machine,Poisoning Attack,Outlier Detection,Federated Learning
AI Read Science
Must-Reading Tree
Example
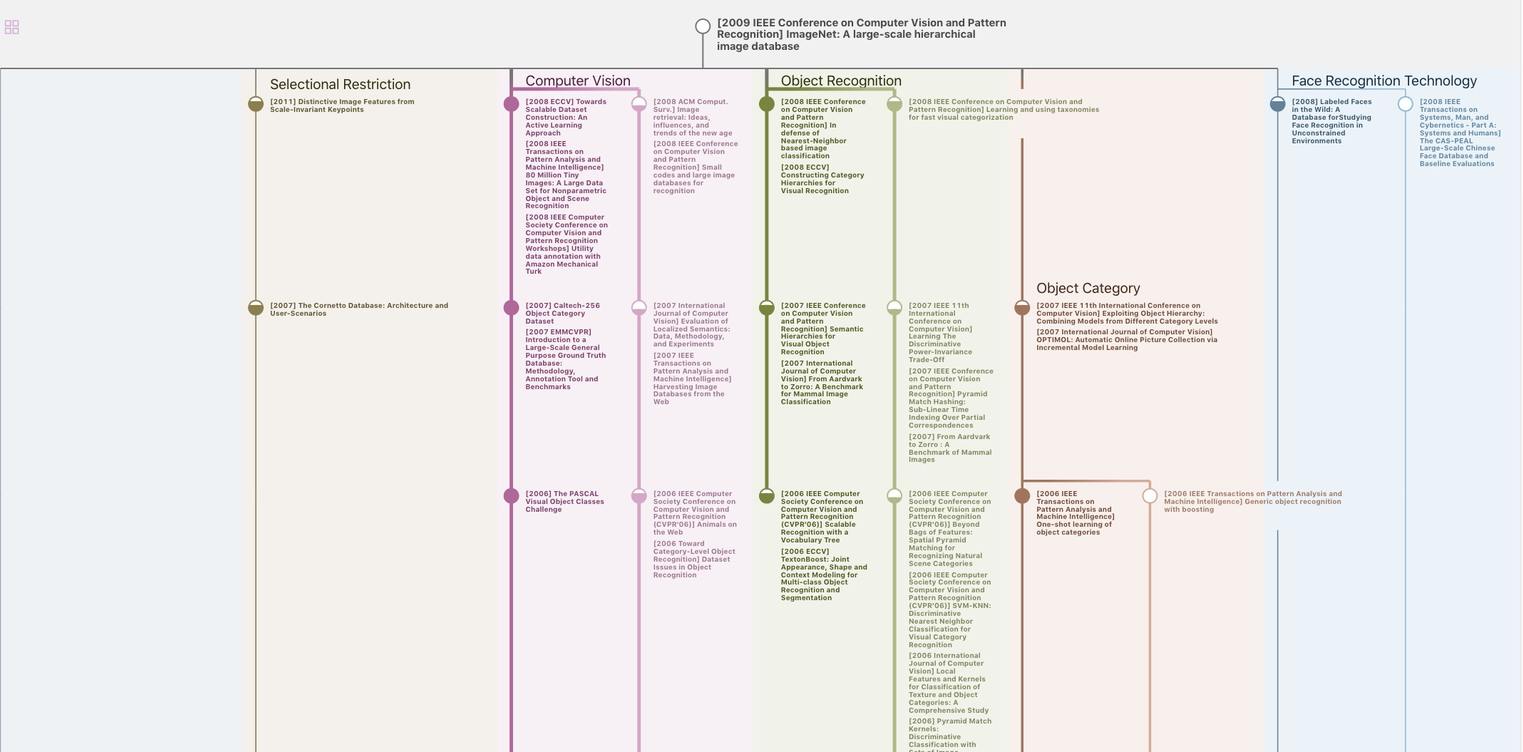
Generate MRT to find the research sequence of this paper
Chat Paper
Summary is being generated by the instructions you defined