Self-Supervised Pretraining via Multimodality Images With Transformer for Change Detection.
IEEE Trans. Geosci. Remote. Sens.(2023)
摘要
Self-supervised learning (SSL) has shown remarkable success in image representation learning. Among these methods, masked image modeling and contrastive learning are the most recent and dominant methods. However, these two approaches will behave differently after being transferred into various downstream tasks. In this article, we propose a red, green, and blue (RGB)-elevation contrastive and image mask prediction pretraining framework. The elevation is normalized digital surface model. Then, we evaluate the learned representation by transferring the pretrained model into change detection (CD) task. To this end, we leverage the recently proposed vision transformer's capability of attending to objects and combine it with the pretext task which consists of masked image modeling and instance discriminant for fine-tuning the spatial tokens. In addition, the CD task also requires us to do information interaction between the two temporal remote sensing images. To counter this problem, we propose a plug-in temporal fusion module based on masked cross attention, and then, we evaluate its effectiveness in three open CD datasets in terms of initializing the supervised training weights. Our method achieves improvements in comparison to supervised learning methods and two mainstream SSL methods momentum contrast (MoCo) and DINO on CD task. The results of our experiment also achieve the state-of-the-art in four CD datasets. The code will be available at URL.
更多查看译文
关键词
Change detection (CD),self-supervised learning (SSL),temporal fusion
AI 理解论文
溯源树
样例
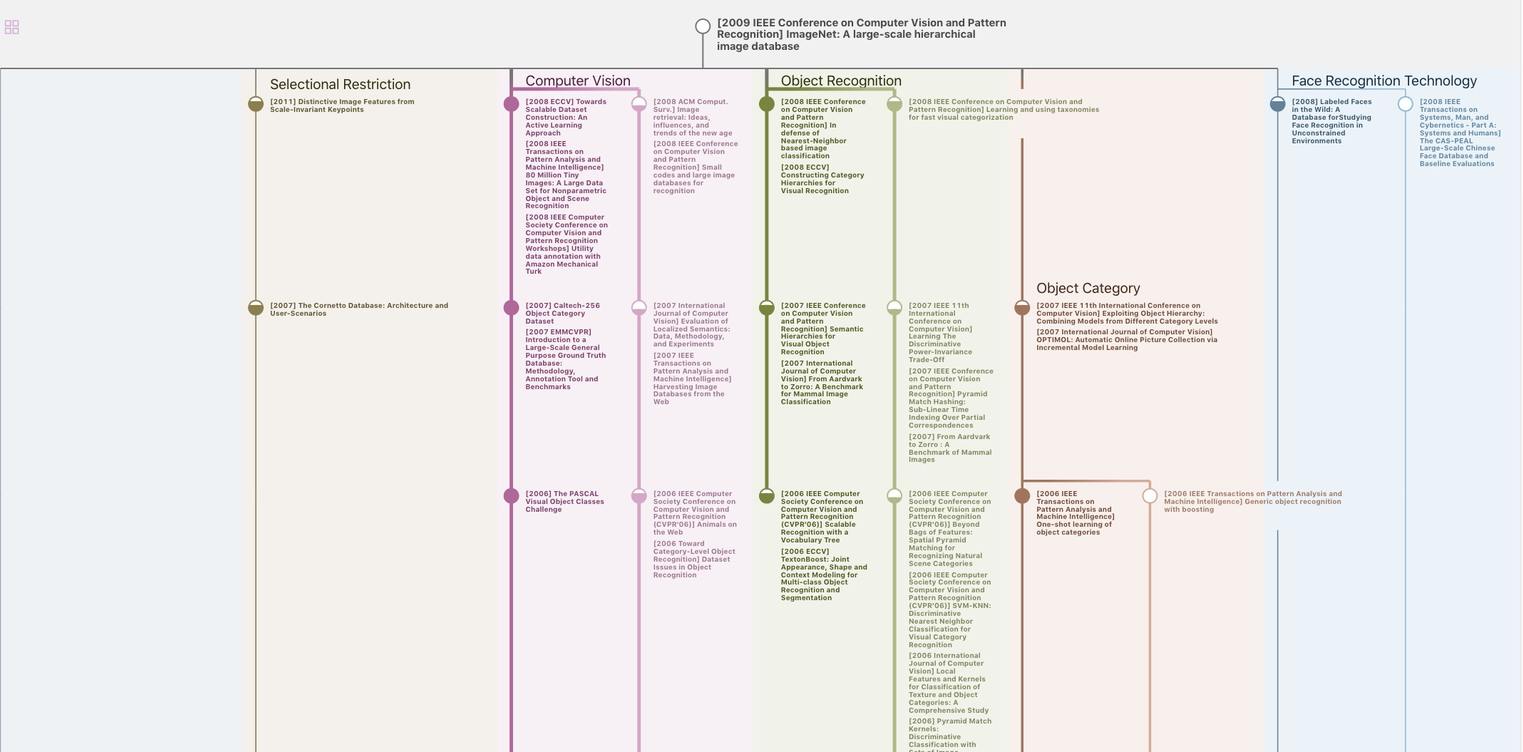
生成溯源树,研究论文发展脉络
Chat Paper
正在生成论文摘要