SM-CNN: Separability Measure-Based CNN for SAR Target Recognition.
IEEE Geosci. Remote. Sens. Lett.(2023)
摘要
With the maturity of deep learning algorithm in synthetic aperture radar (SAR) target recognition field, convolutional neural network (CNN) has become the most effective model. However, the interpretability and the separability of feature maps extracted from convolution layers have not been specially analyzed neither qualitatively nor quantitatively, which makes the traditional model work like a "black box." To alleviate the problem, a novel model based on separability measure (SM)CNN is proposed in this letter, which introduces the principle of maximal coding rate reduction (MCR2) to the backbone module. SM-CNN quantitatively analyzes the separability of the feature maps and takes the value as a vital part of the loss function to guide the training process of the model. The calculation process of the SM values can be strictly derived mathematically, so it is more interpretable, turning the black box into a "gray box." In addition, the proposed model can achieve comparable recognition performance of the backbone networks with reduced computational complexity. Comparative experiments based on moving and stationary target acquisition and recognition (MSTAR) and OpenSARShip datasets verify the effectiveness and practicability of the method proposed in this letter.
更多查看译文
关键词
sar target,separability measure,recognition,sm-cnn
AI 理解论文
溯源树
样例
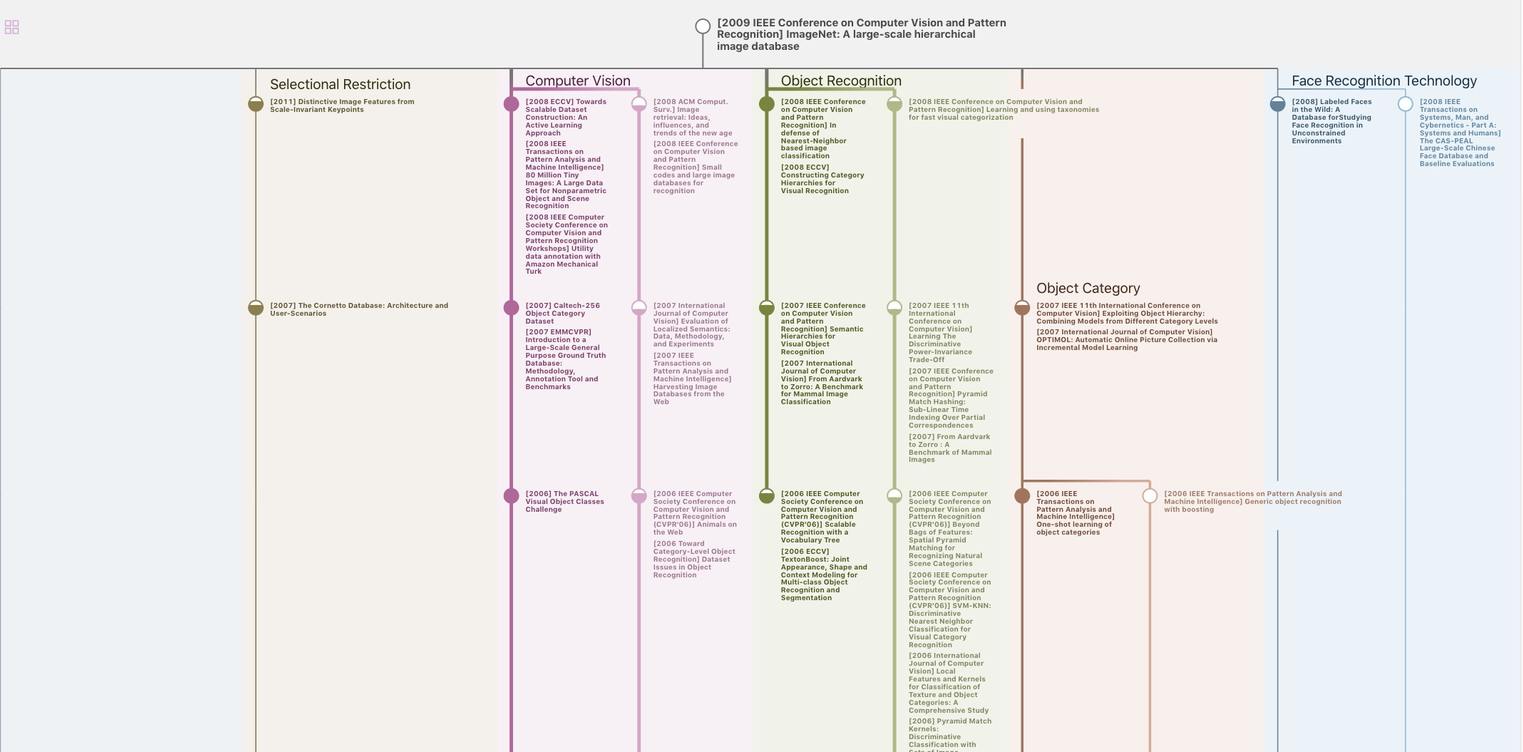
生成溯源树,研究论文发展脉络
Chat Paper
正在生成论文摘要