LEMON: Alternative Sampling for More Faithful Explanation Through Local Surrogate Models.
IDA(2023)
摘要
Local surrogate learning is a popular and successful method for machine learning explanation. It uses synthetic transfer data to approximate a complex reference model. The sampling technique used for this transfer data has a significant impact on the provided explanation, but remains relatively unexplored in literature. In this work, we explore alternative sampling techniques in pursuit of more faithful and robust explanations, and present LEMON: a sampling technique that samples directly from the desired distribution instead of reweighting samples as done in other explanation techniques (e.g., LIME). Next, we evaluate our technique in a synthetic and UCI dataset-based experiment, and show that our sampling technique yields more faithful explanations compared to current state-of-the-art explainers.
更多查看译文
关键词
models,sampling,more faithful explanation,surrogate
AI 理解论文
溯源树
样例
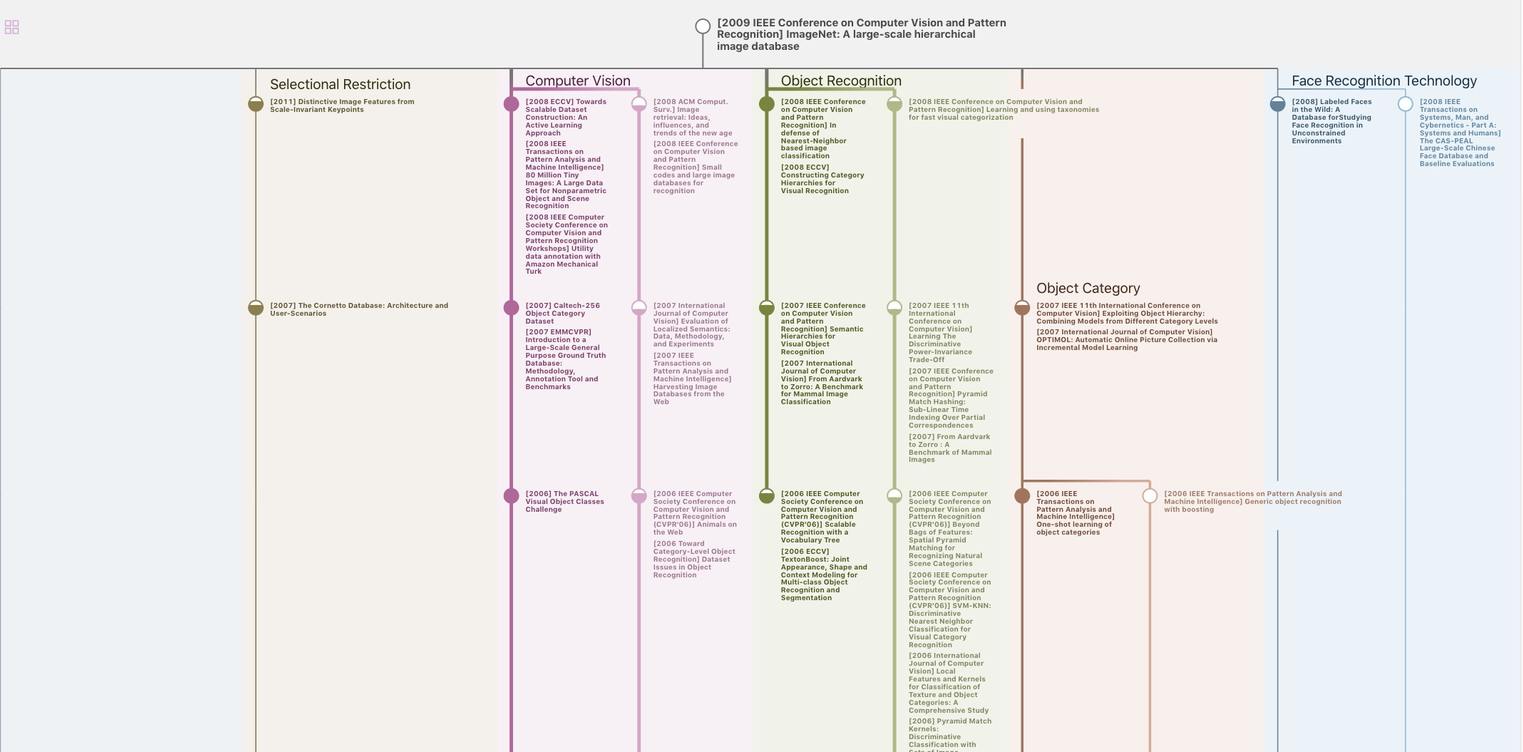
生成溯源树,研究论文发展脉络
Chat Paper
正在生成论文摘要