Cross-Resolution Semi-Supervised Adversarial Learning for Pansharpening.
IEEE Trans. Geosci. Remote. Sens.(2023)
摘要
Existing deep neural network (DNN)-based methods have produced good pansharpened images. However, supervised DNN-based pansharpening methods suffer from performance degradation when fusing low spatial resolution multispectral (LR MS) and panchromatic (PAN) images at full resolution. Unsupervised DNN-based methods alleviate the issue, but their training becomes difficult owing to the absence of reference images. This article establishes a novel semi-supervised framework to jointly learn the reconstructions of fused images from reduced- and full-resolution datasets. Specifically, we propose a cross-resolution semi-supervised adversarial learning network (CrossNet), which is composed of a supervised module and an unsupervised module. In these two modules, reduced- or full-resolution source images are disentangled as resolution-invariant components and resolution-aware components by reconstructing the fused images. Moreover, cross-resolution fused images are synthesized to enhance the disentanglement of the two kinds of components. Through the reconstruction of cross-resolution fused images, supervised and unsupervised modules are also coupled efficiently. Then, the semi-supervised framework can simultaneously make use of the supervised information in reduced-resolution datasets and mitigate the performance degradation via full-resolution datasets. Besides, adversarial learning is employed to improve the consistency between resolution-invariant components of source images at different resolutions. Finally, extensive experiments on QuickBird, GeoEye-1, WorldView-2, and WorldView-3 datasets demonstrate that the proposed CrossNet can produce state-of-the-art fusion results in terms of qualitative and quantitative evaluations. The source code is available at https://github.com/RSMagneto/CrossNet.
更多查看译文
关键词
pansharpening,learning,cross-resolution,semi-supervised
AI 理解论文
溯源树
样例
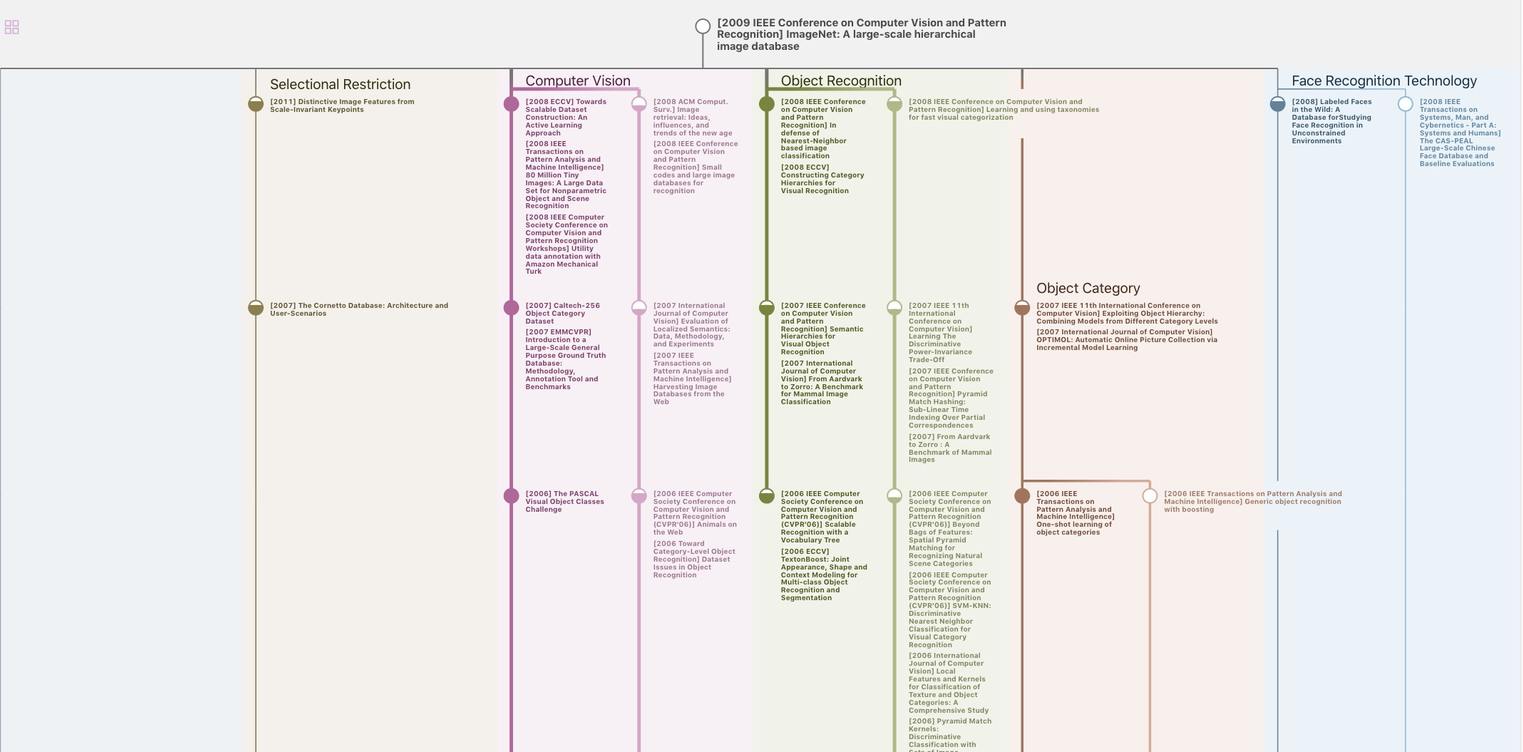
生成溯源树,研究论文发展脉络
Chat Paper
正在生成论文摘要