A Deep Learning-Based In Situ Analysis Framework for Tropical Cyclogenesis Prediction.
HIPC(2022)
Abstract
Tropical cyclone is one of the most violent natural disasters causing massive devastation. Accurate forecasting of cyclones with high lead times is an important problem. We propose a framework to predict tropical cyclogenesis (i.e. cyclone formation). This framework executes along with a parallel weather simulation model (WRF) and analyzes the simulation output as soon as they are generated. Our framework has two major components - a trigger function and a deep predictive model. The trigger function acts as a basic filter to identify cyclones from non-cyclones. The proposed deep learning model is based on convolutional neural networks (CNNs). The best track data from Indian Meteorological Department (IMD) is used as a reference for labeling data points into disturbances and tropical cyclones. The framework achieves a probability of detection (POD) value of approximately 95% with a false alarm ratio (FAR) of 21.69% overall. The predictions made by the framework have a lead time of up to 150 hours from the time that a disturbance transforms into a tropical cyclone.
MoreTranslated text
Key words
tropical cyclones, cyclogenesis, deep learning, CNN, ConvLSTM, trigger, WRF
AI Read Science
Must-Reading Tree
Example
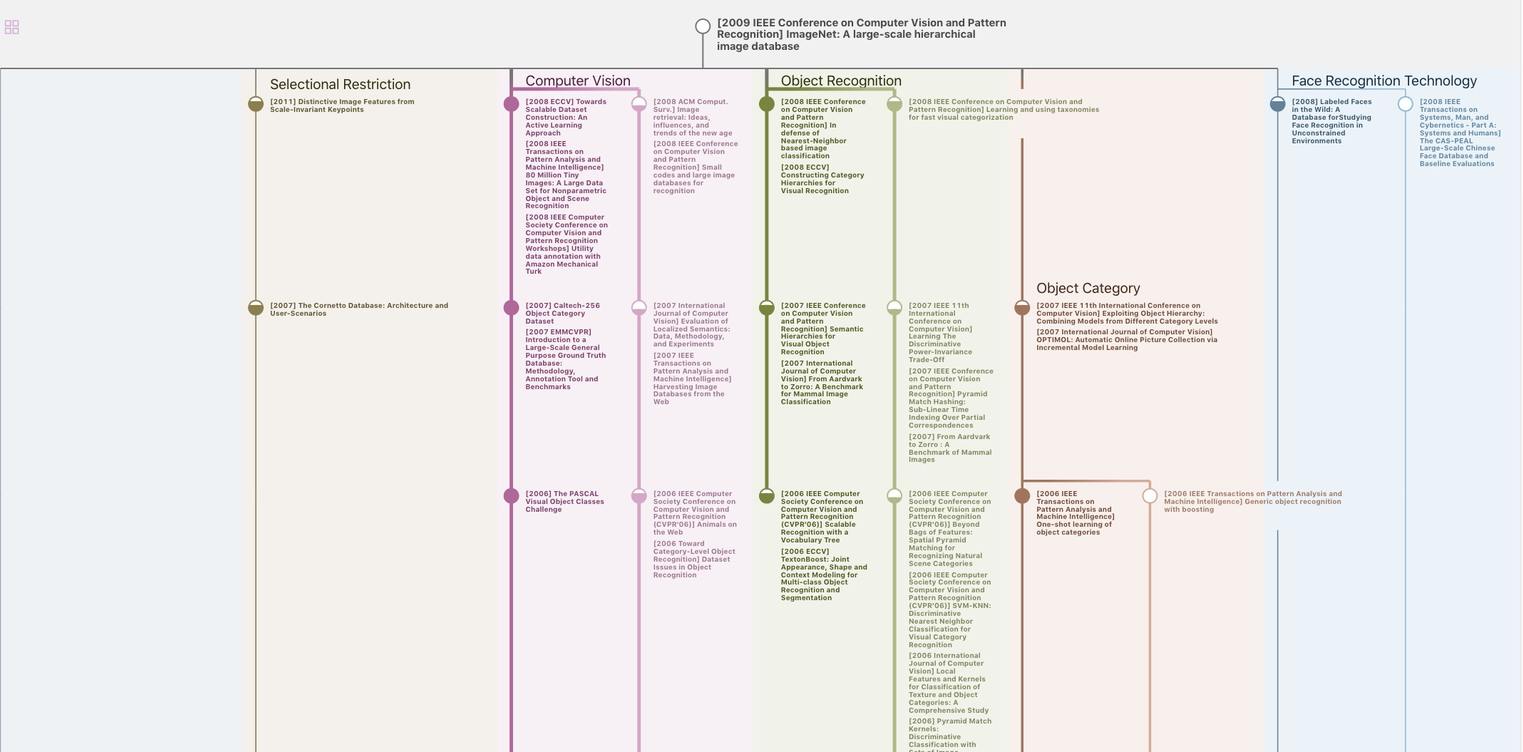
Generate MRT to find the research sequence of this paper
Chat Paper
Summary is being generated by the instructions you defined