LSTM-ReGAT: A network-centric approach for cryptocurrency price trend prediction
Decis. Support Syst.(2023)
摘要
Predicting price trends of cryptocurrencies is a challenging task due to the highly speculative cryptocurrency market. Prior studies mainly investigate predictors such as historical trading data, macroeconomic development, and public interests in cryptocurrencies for price trend prediction while ignoring the predictive role of the relations between cryptocurrencies. In fact, the price movement of a cryptocurrency may be affected by those of other cryptocurrencies, thus, incorporating cryptocurrency interrelations can further improve the prediction performance. In this paper, we propose a novel end-to-end model for price trend prediction using long short-term memory (LSTM) and relationwise graph attention network (ReGAT), in which both individual cryptocurrency features and cryptocurrency relations are considered. Specifically, a cryptocurrency network is built by using shared features between cryptocurrencies. Further, LSTM is used to profile sequential patterns of individual cryptocurrency features. To fully extract network features, ReGAT is designed to aggregate information conveyed by different types of relations while automatically differentiating their importance. Finally, the conventional and network features are concatenated to predict the price trend. The effectiveness of LSTM-ReGAT is validated using real-world cryptocurrency market data. The trading simulations for Bitcoin and portfolios reveal that our model obtains the highest profits. Our study provides insightful implications for investment decision support in cryptocurrency market.
更多查看译文
关键词
Cryptocurrency,Price trend prediction,Cryptocurrency network,Graph attention network
AI 理解论文
溯源树
样例
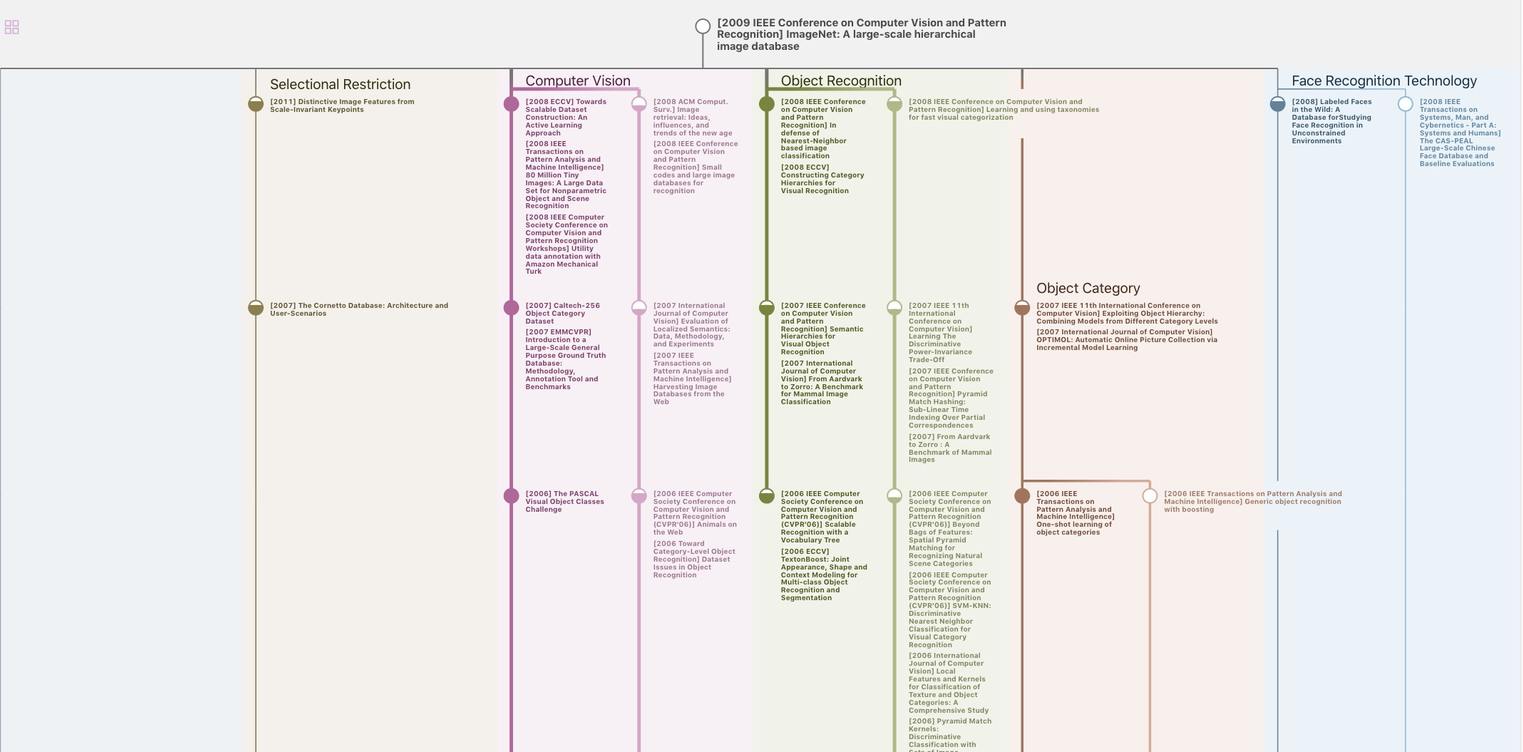
生成溯源树,研究论文发展脉络
Chat Paper
正在生成论文摘要