Multi-user reinforcement learning based multi-reward for spectrum access in cognitive vehicular networks
Telecommun. Syst.(2023)
Abstract
Cognitive Vehicular Networks (CVNs) can improve spectrum utilization by intelligently using idle spectrum, so as to fulfill the needs of communication. The previous researches only considered vehicle-to-vehicle(V2V) links or vehicle-to-infrastructure (V2I) links and ignored the influence of spectrum sensing errors. Therefore, in this paper, V2V links and V2I links are simultaneously discussed in the presence of spectrum sensing errors in the CVNs communication environment that we establish, and a dynamic spectrum access problem aiming at spectrum utilization is framed. In order to solve the above problems, the reinforcement learning method is introduced in this paper. But the impact of two kinds of collisions on the spectrum access rate of cognitive vehicles is neglected in the reinforcement learning method, and the above collisions which exist between cognitive vehicles, between cognitive vehicles and primary vehicles. Hence, different reward functions are designed according to different collision situations, and an improved reinforcement learning method is utilized to improve the success probability of spectrum access. To verify the effectiveness of the improved method, the performance and convergence of the proposed method are significantly better than other methods by comparing with the Myopic method, DQN and traditional DDQN in Python.
MoreTranslated text
Key words
Cognitive Vehicular Networks,Dynamic spectrum access,Vehicle-to-vehicle links,Vehicle-to-infrastructure links,Spectrum sensing errors
AI Read Science
Must-Reading Tree
Example
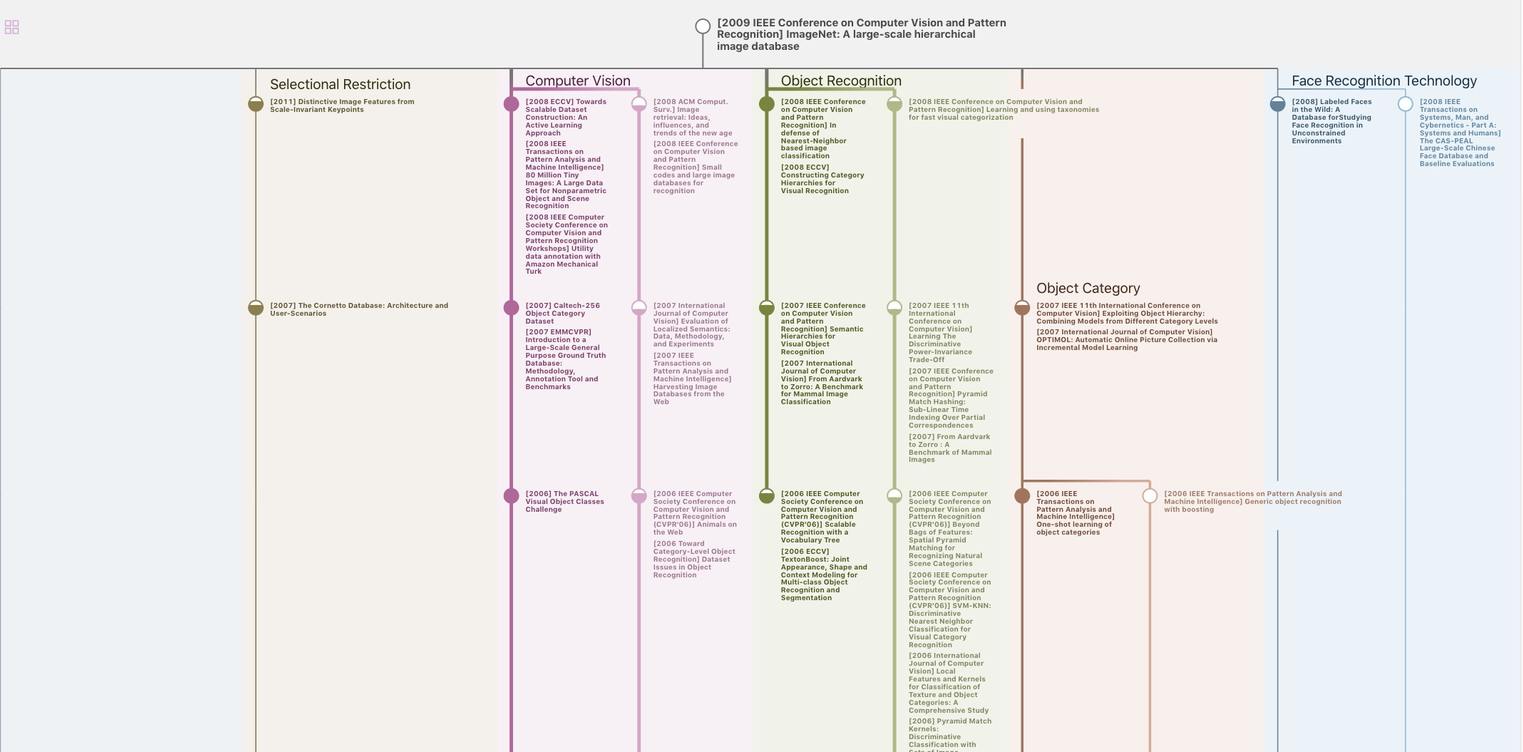
Generate MRT to find the research sequence of this paper
Chat Paper
Summary is being generated by the instructions you defined