A Novel Explainable Link Forecasting Framework for Temporal Knowledge Graphs Using Time-Relaxed Cyclic and Acyclic Rules.
PAKDD (1)(2023)
摘要
Link forecasting in a temporal Knowledge Graph (tKG) involves predicting a future event from a given set of past events. Most previous studies suffered from reduced performance as they disregarded acyclic rules and enforced a tight constraint that all past events must exist in a strict temporal order. This paper proposes a novel explainable rule-based link forecasting framework by introducing two new concepts, namely ‘relaxed temporal cyclic and acyclic random walks’ and ‘link-star rules’. The former concept involves generating rules by performing cyclic and acyclic random walks on a tKG by taking into account the real-world phenomenon that the order of any two events may be ignored if their occurrence time gap is within a threshold value. Link-star rules are a special class of acyclic rules generated based on the natural phenomenon that history repeats itself after a particular time. Link-star rules eliminate the problem of combinatorial rule explosion, thereby making our framework practicable. Experimental results demonstrate that our framework outperforms the state-of-the-art by a substantial margin. The evaluation measures hits@1 and mean reciprocal rank were improved by 45% and 23%, respectively.
更多查看译文
关键词
Knowledge Graphs, Graph Analytics, Forecasting
AI 理解论文
溯源树
样例
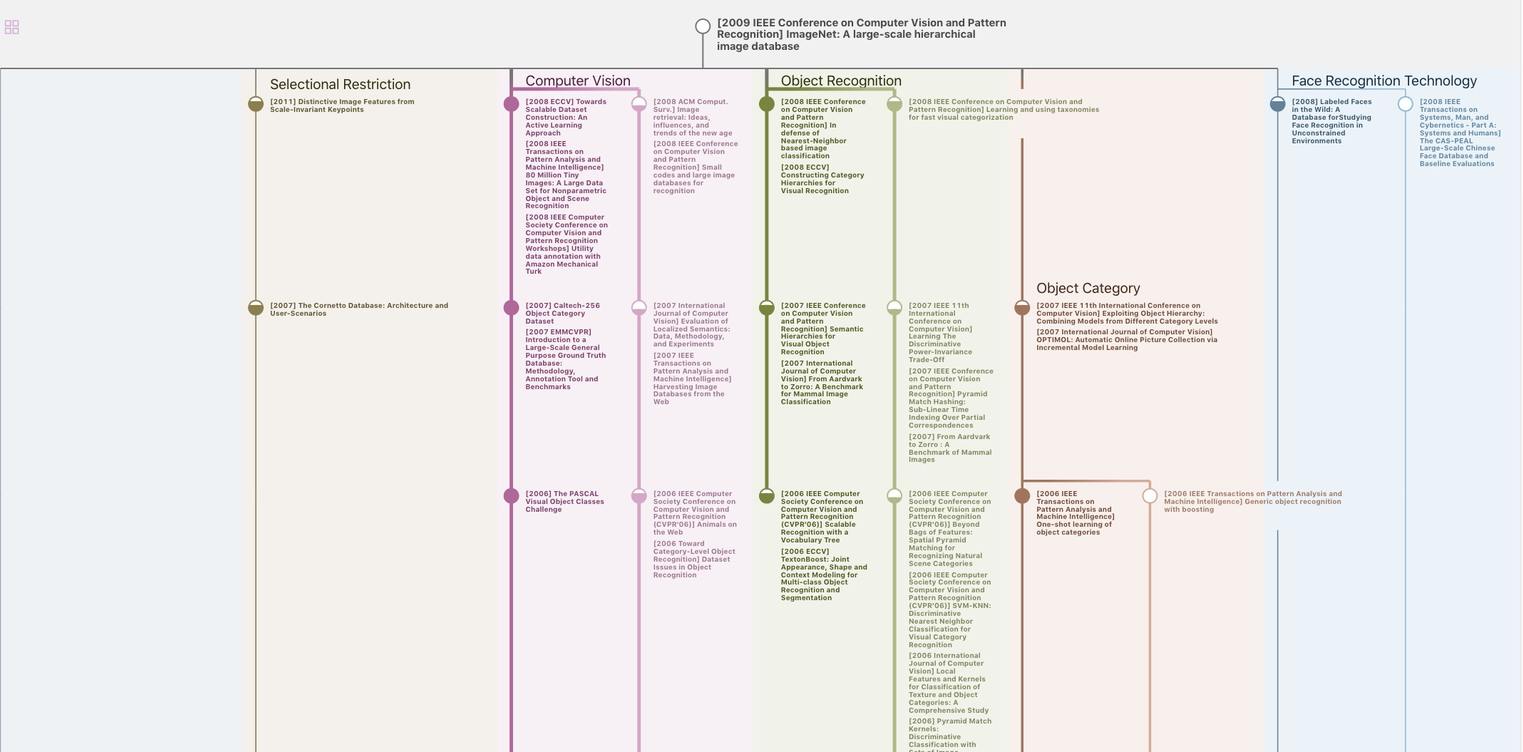
生成溯源树,研究论文发展脉络
Chat Paper
正在生成论文摘要