Achieving Provable Byzantine Fault-tolerance in a Semi-honest Federated Learning Setting.
PAKDD (2)(2023)
摘要
Federated learning (FL) is a suite of technology that allows multiple distributed participants to collaboratively build a global machine learning model without disclosing private datasets to each other. We consider an FL setting in which there may exist both a) semi-honest participants who aim to eavesdrop on other participants’ private datasets; and b) Byzantine participants who aim to degrade the performances of the global model by submitting detrimental model updates. The proposed framework leverages the Expectation-Maximization algorithm first in E-step to estimate unknown participant membership, respectively, of Byzantine and benign participants, and in M-step to optimize the global model performance by excluding malicious model updates uploaded by Byzantine participants. One novel feature of the proposed method, which facilitates reliable detection of Byzantine participants even with HE or MPC protections, is to estimate participant membership based on the performances of a set of randomly generated candidate models evaluated by all participants. The extensive experiments and theoretical analysis demonstrate that our framework guarantees Byzantine Fault-tolerance in various federated learning settings with private-preserving mechanisms.
更多查看译文
关键词
fault-tolerance,semi-honest
AI 理解论文
溯源树
样例
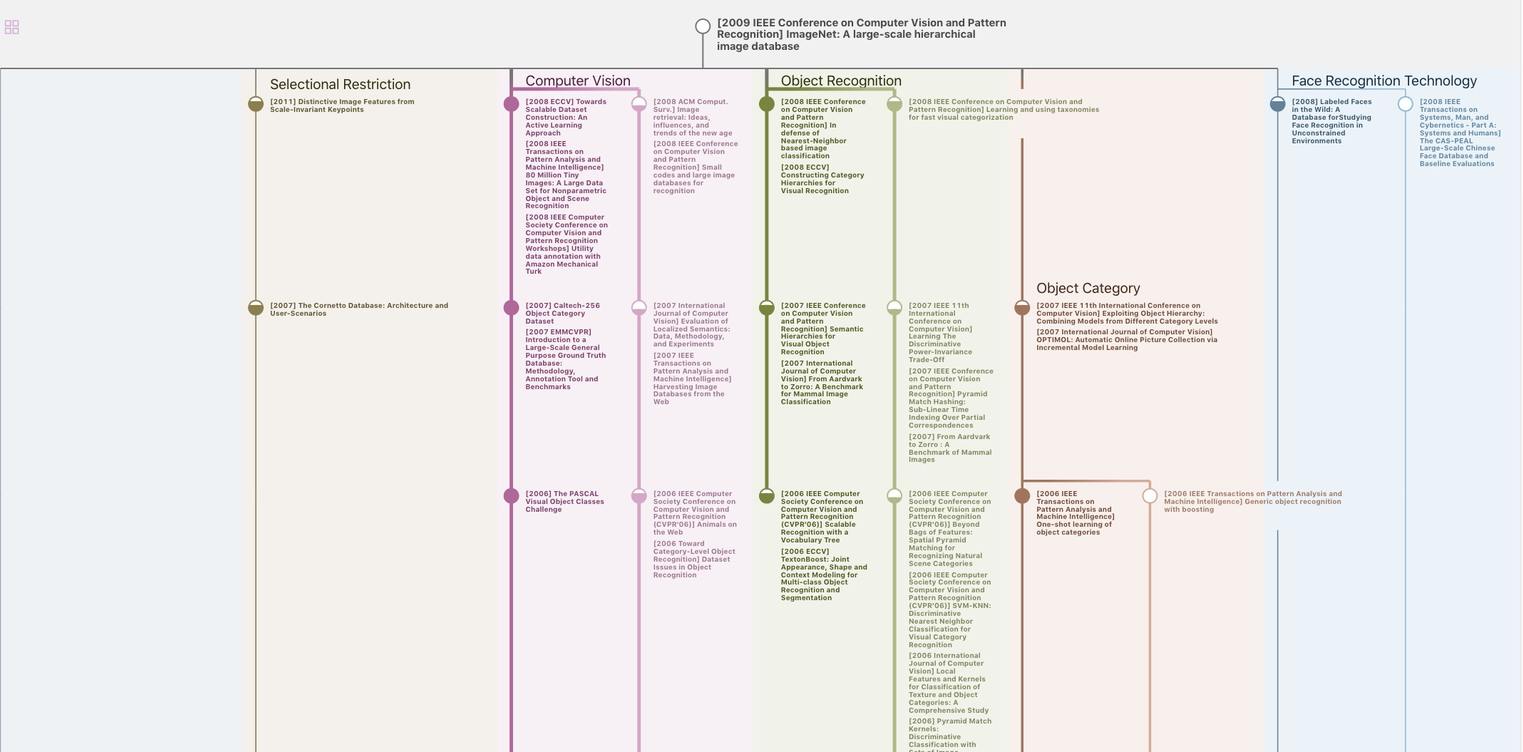
生成溯源树,研究论文发展脉络
Chat Paper
正在生成论文摘要