A New Reconstruction Attack: User Latent Vector Leakage in Federated Recommendation.
DASFAA (2)(2023)
Abstract
Federated Recommendation (FR) has received considerable attention in the past few years. For each user in FR, its latent vector and interaction data are kept on its local device and thus are private to others. However, keeping the training data locally can not ensure the user’s privacy is compromised. In this paper, we show that the existing FR is vulnerable to a new reconstruction attack in which the attacker leverages the semi-trusted FR server to lunch the reconstruction attack. In this attack, the server rigidly follows the protocol of FR, but the attacker may compromise the system security by analyzing the gradient updates received by the server. Specifically, we design Generative Reconstruction Network (GRN), a model reconstructing attack against FR aiming to generate the target user’s (i.e., the victim) latent vector including user’s sensitive information. Moreover, a server-side generator is designed to take random vectors as inputs and outputs generated latent vectors. The generator is trained by the distance between the real victim’s gradient updates and the generated gradient updates. We explain that the generator will successfully learn the target latent vector distribution to probe into the victim’s privacy. The experimental results demonstrate the proposed attack’s effectiveness and superiority over the baseline attacks.
MoreTranslated text
Key words
user latent vector leakage,federated recommendation,new reconstruction attack
AI Read Science
Must-Reading Tree
Example
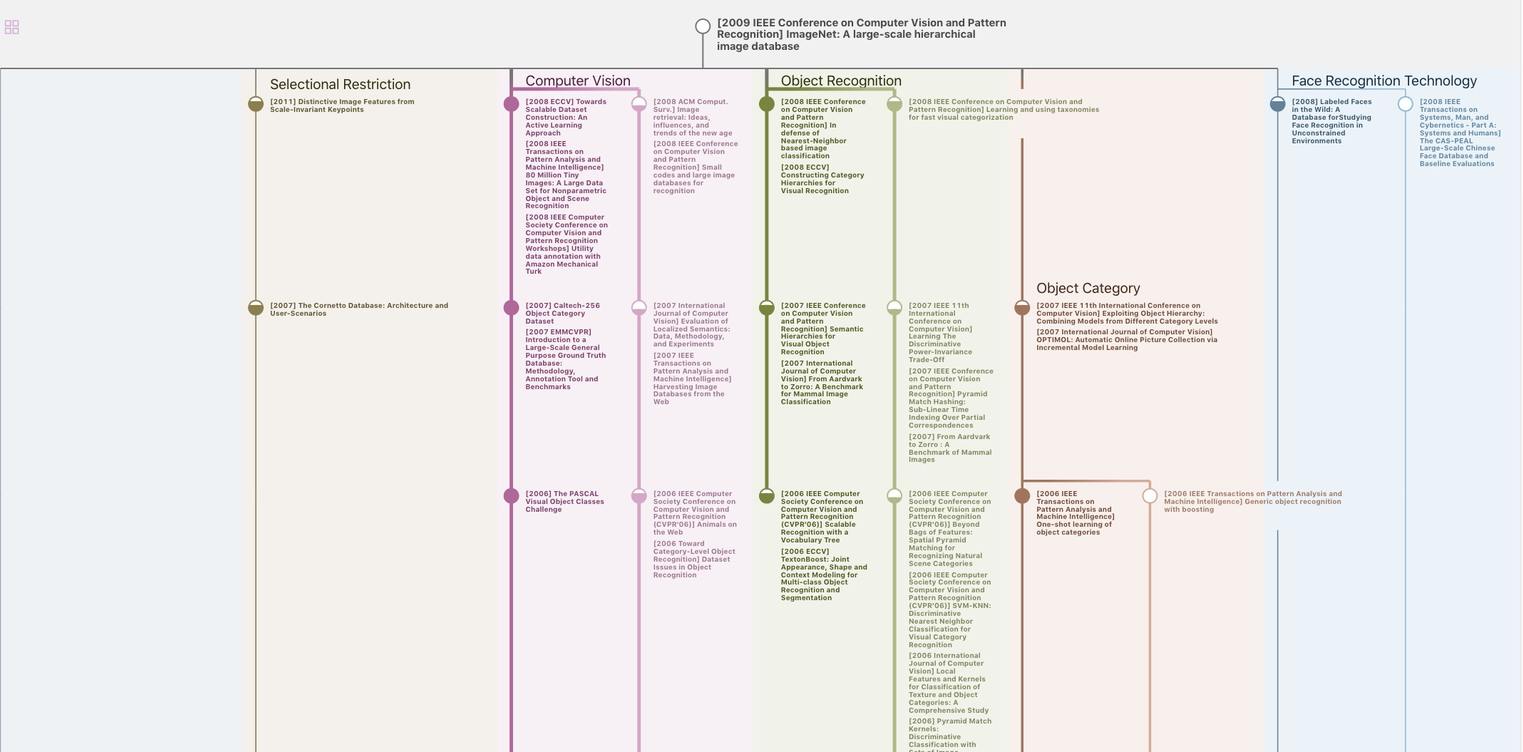
Generate MRT to find the research sequence of this paper
Chat Paper
Summary is being generated by the instructions you defined