A Three-Layer Attentional Framework Based on Similar Users for Dual-Target Cross-Domain Recommendation.
DASFAA (2)(2023)
摘要
To address the long-standing data sparsity problem in recommender systems (RSs), cross-domain recommendation (CDR) has been proposed. Traditional CDR leverages the relatively richer information from a richer domain to improve recommendation performance in a sparser domain, which is also called single-target CDR. In recent years, dual-target CDR has been proposed to improve recommendation performance in both domains simultaneously. The existing dual-target CDR methods are based on common users between domains, where they extract the embeddings of common users and then transfer the embeddings to the two target domains to improve recommendation performance. However, in real life, the proportion of common users between domains is usually very small, which makes it hard to generate representative and high-quality user embeddings, and thus, limits the performance of the existing methods in real applications. To address this problem, in this paper, we propose a T hree-Layer A ttentional Framework based on S imilar U sers, called TASU. In addition to common users, TASU leverages information from similar users to improve the quality of user embeddings. By a three-layer attentional framework, TASU can generate more representative and high-quality user embeddings to improve recommendation performance in both domains. Extensive experiments conducted on three real-world datasets demonstrate that TASU significantly outperforms the state-of-the-art approaches.
更多查看译文
关键词
similar users,three-layer,dual-target,cross-domain
AI 理解论文
溯源树
样例
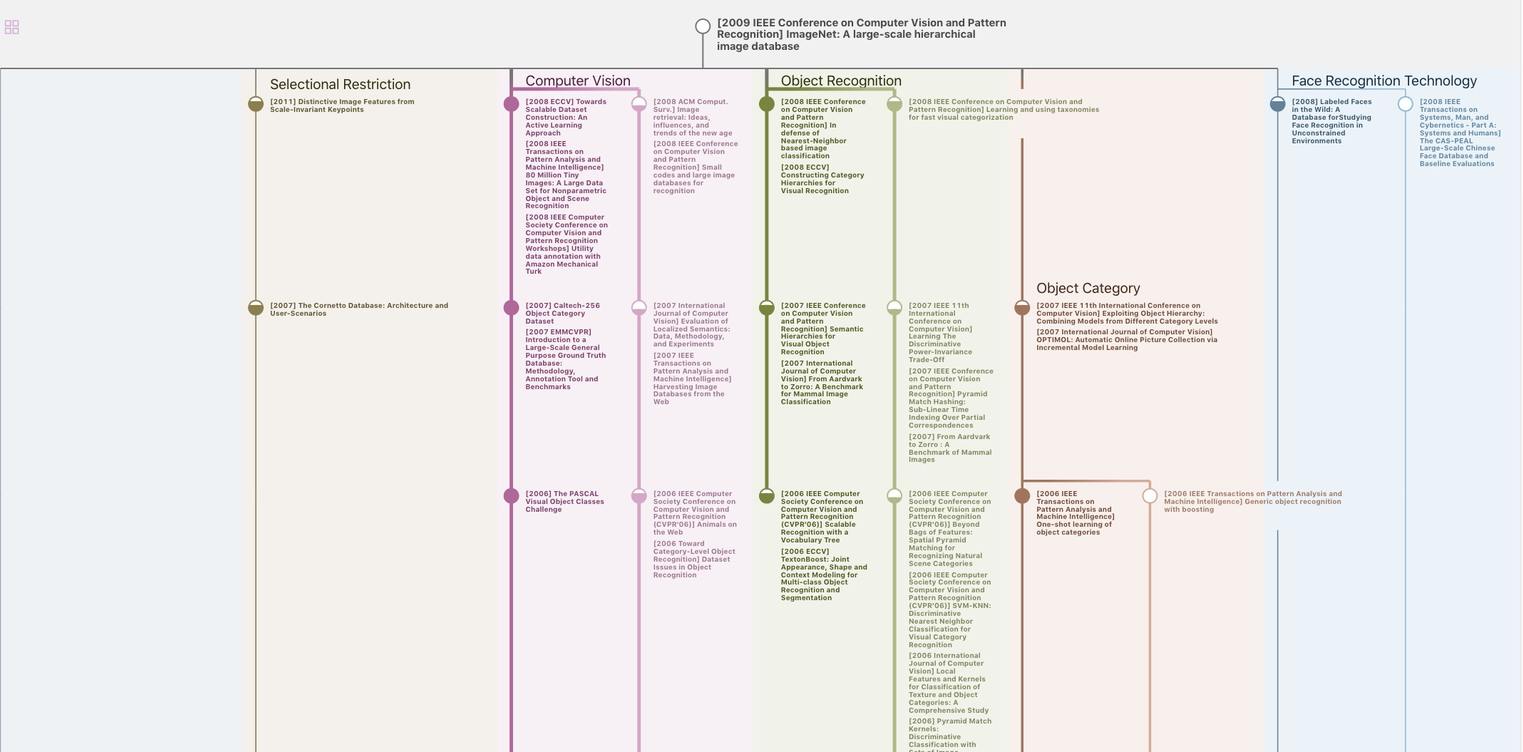
生成溯源树,研究论文发展脉络
Chat Paper
正在生成论文摘要