Learning to Rank using Fuzzy-Enhanced LSTM Model.
WI/IAT(2022)
摘要
Underlying information in re-ranking models plays a major role in personalization systems. In these systems, user profiles improve the quality of results created by search engines. A combination of the extracted underlying information and deep learning methods has yielded significant results recently. Models based on Long Short-Term Memory(LSTM) have been considered in this field. In this paper, a fuzzy-enhanced model based on the topic model is proposed to integrate the topic-based user profile into the LSTM model. The topic-based user profiles are created using fuzzy logic to handle the uncertainty of the occurrence of all topics in a document. This model is used in an LSTM-based framework to re-rank search results on a input query using the sequence of queries and click history. To evaluate the proposed model, the results are compared between the model and the baseline model created using Latent Dirichlet Allocation (LDA). The result shows an improvement in the Fuzzy-enhanced LSTM model compared to the base model.
更多查看译文
关键词
Fuzzy-enhanced models, LSTM model, Ranking methods
AI 理解论文
溯源树
样例
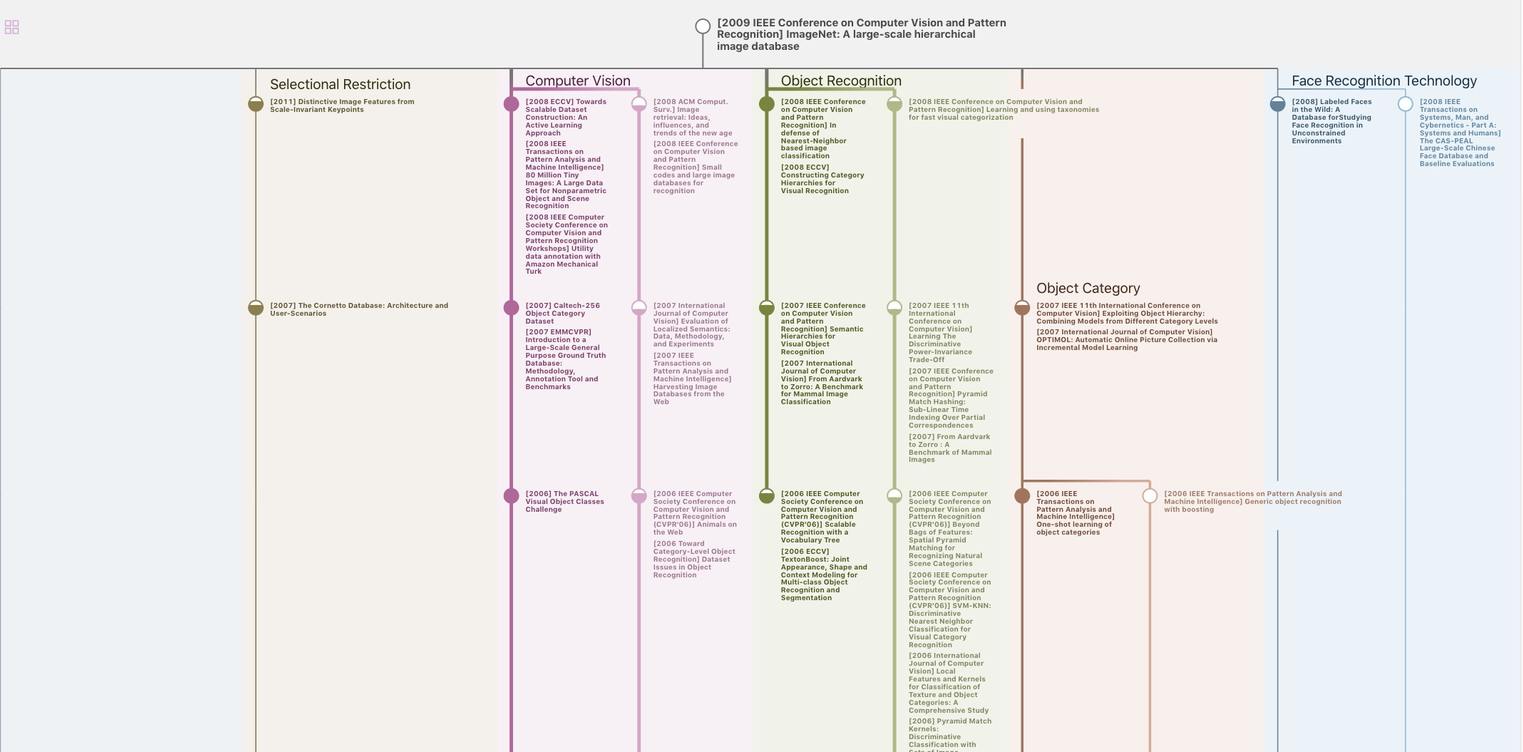
生成溯源树,研究论文发展脉络
Chat Paper
正在生成论文摘要