Differential evolution based on network structure for feature selection.
Inf. Sci.(2023)
摘要
With the accumulation of high-dimensional data, feature selection (FS) plays a significant role in tasks such as classification and clustering. Recently, many evolutionary algorithms have been developed to address FS problems from single-objective or multiobjective perspectives. However, those algorithms always ignore the complex relationships among features containing abundant available information to adjust the search direction, resulting in poor performance. To address this limitation, we propose to explore the relationship patterns hidden in feature space using the techniques of network science, and based on the exploration, we develop a new differential evolution (DE) and a new multiobjective DE (MODE) for single-objective and multiobjective FS problems, respectively. In particular, we construct a feature network to represent the complex relationships between features and propose to explore the relational patterns from the perspective of the network. After that, the features with rich information are highlighted as high-degree nodes, and some features are grouped together as clusters in the feature network. Motivated by these observations, we further propose to improve the search processes of DE and MODE and produce two network-based DEs, namely, NetG-DE and NetG-MODE. Experimental results over several datasets demonstrate that the proposed NetG-DE and NetG-MODE outperform the baseline.
更多查看译文
关键词
differential evolution,feature selection,network structure
AI 理解论文
溯源树
样例
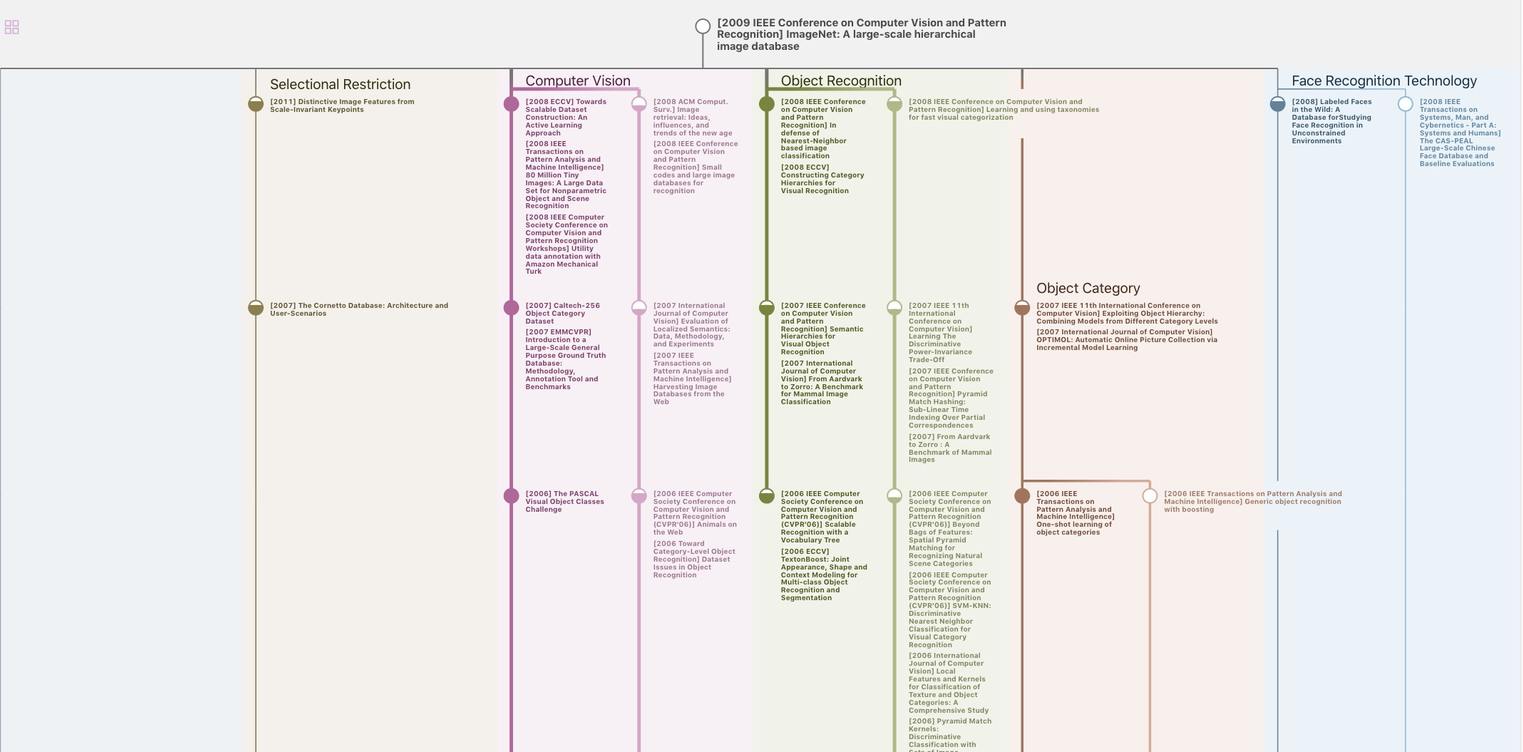
生成溯源树,研究论文发展脉络
Chat Paper
正在生成论文摘要