Communication Efficient Heterogeneous Federated Learning based on Model Similarity.
WCNC(2023)
摘要
Federated Learning is now widely used to train neural networks under distributed datasets. One of the main challenges in Federated Learning is to address network training under local data heterogeneity. Existing work proposes that taking similarity into account as an influence factor in federated learning can improve the speed of model aggregation. We propose a novel approach that introduces Centered Kernel Alignment (CKA) into loss function to compute the similarity of feature maps in the output layer. Compared to existing methods, our method enables fast model aggregation and improves global model accuracy in non-IID scenario by using Resnet50.
更多查看译文
关键词
Federated Learning, Centered Kernel Alignment, Non-IID Data, Heterogeneity, Communication Efficient
AI 理解论文
溯源树
样例
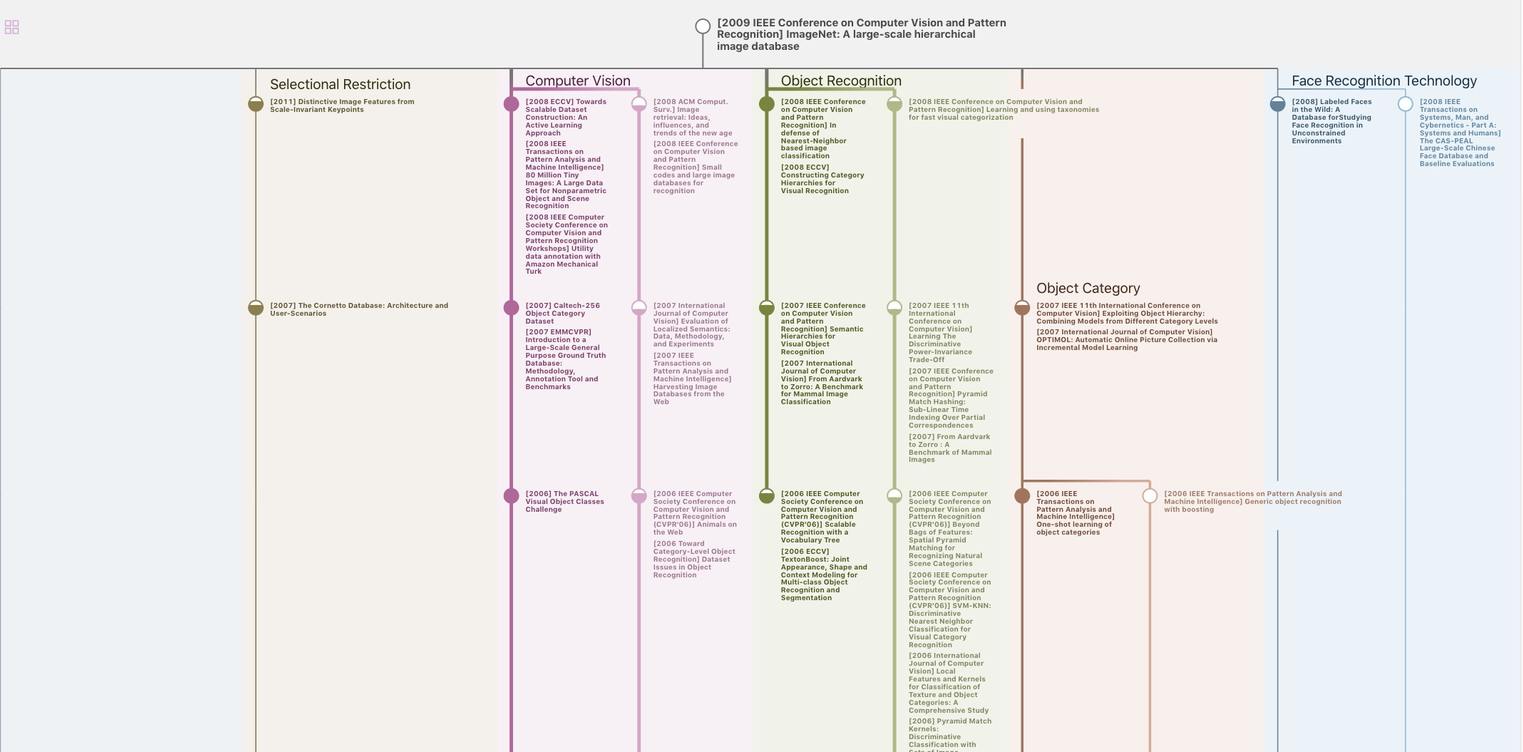
生成溯源树,研究论文发展脉络
Chat Paper
正在生成论文摘要