Stratification-based semi-supervised clustering algorithm for arbitrary shaped datasets.
Inf. Sci.(2023)
摘要
Semi-supervised clustering is not only an important branch of semi-supervised learning but also an improvement direction for clustering. Semi-supervised clustering algorithms designed based on Kmeans, such as the classical Seeded-Kmeans and Constrained-Kmeans, where supervision information is used to guide clustering iterations, have the same disadvantages as the original Kmeans algorithm: they are confined to the assumption of isotropic spherical clusters, leading to the narrow adaptability in handling data of various characteristics. To solve the problem, we propose the scattered centroids initialization clustering algorithm based on Stratification (SCICS). First, based on the concept of influence space, a method for modeling the cluster-level location of any object is presented, according to which we can obtain well-defined cluster decision boundaries through stratification. On this basis, by extending the seed thought, we propose a semi-supervised subclustering algorithm that can break through the limitations of partitional clustering methods that rely on strict assumptions on particular cluster distributions. Experiments on artificial and real-world datasets show that the proposed algorithm gains the ability of clustering arbitrary shaped data and surpasses the competitors in terms of performance and adaptability.
更多查看译文
关键词
Semi-supervised clustering, Kmeans, Seeded-Kmeans, Partitional clustering, Influence space
AI 理解论文
溯源树
样例
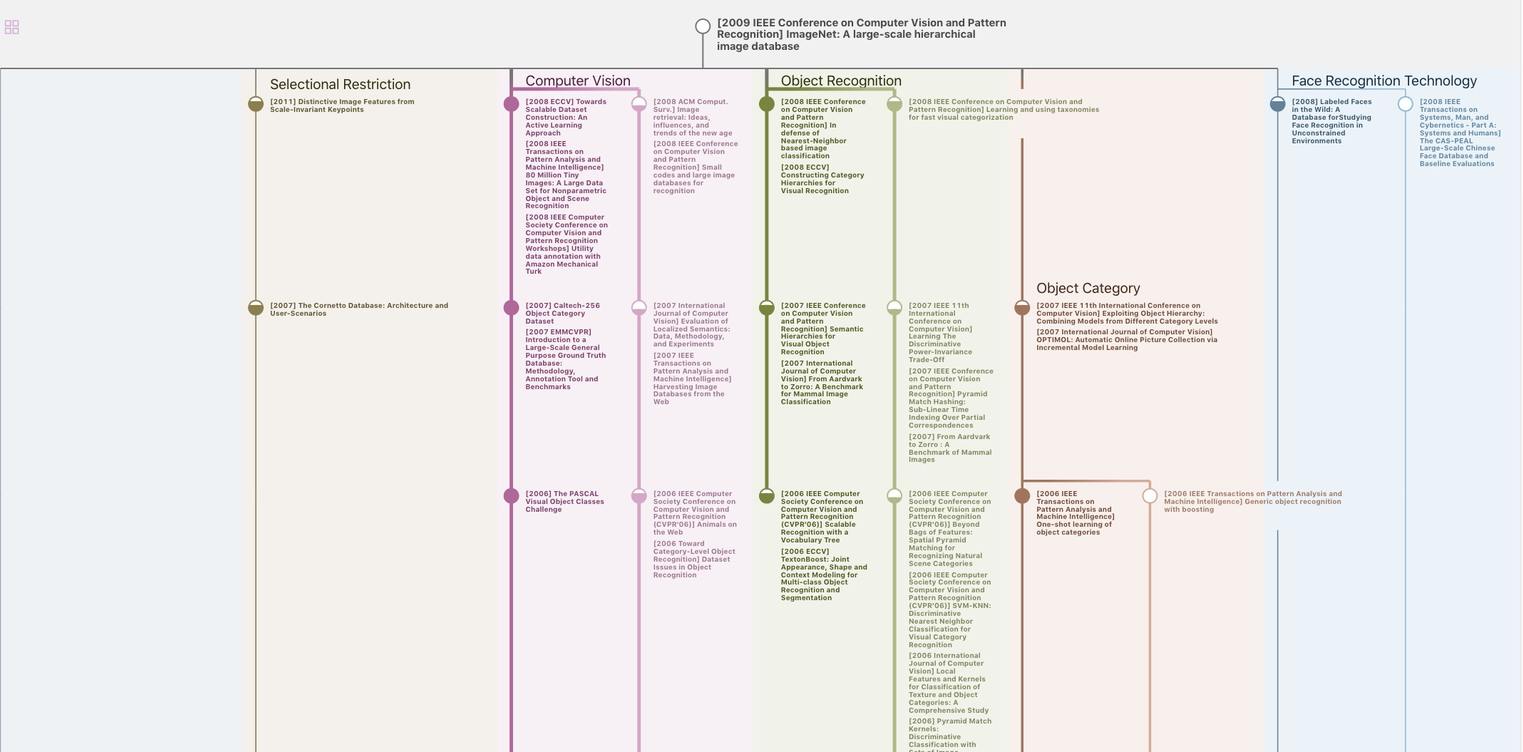
生成溯源树,研究论文发展脉络
Chat Paper
正在生成论文摘要