Low-rank with sparsity constraints for image denoising.
Inf. Sci.(2023)
摘要
Recent works on providing proper sparse or low-rank priors have shown to result in good quality image restoration performance. The nonlocal self-similarity (NSS) of images indicates that groups stacked by similar patches not only have low-rank characteristic, but also have sparse characteristic. However, many of the existing methods for image modeling use one or the other of these characteristics, but not both, which may limit the performance of image denoising. We propose in this paper a novel model for image denoising, designated as the bilateral weighted sparse coding and low-rank (BWSCLR) model, which exploits simultaneously the low-rank property and the bilateral weighted sparse coding under the NSS prior to better restore the texture and the edges of images contaminated by noise. Furthermore, a block coordinate descent algorithm involving three steps is proposed to obtain the solution for our model. A number of experiments on denoising different image scenarios, including gray-scale and color images are carried out, and the results clearly show the superior performance of the proposed BWSCLR method over that of the state-of-the-art algorithms not only in terms of the quantitative metrics such as PSNR and SSIM, but also in perceptual quality.
更多查看译文
关键词
image denoising,sparsity constraints,low-rank
AI 理解论文
溯源树
样例
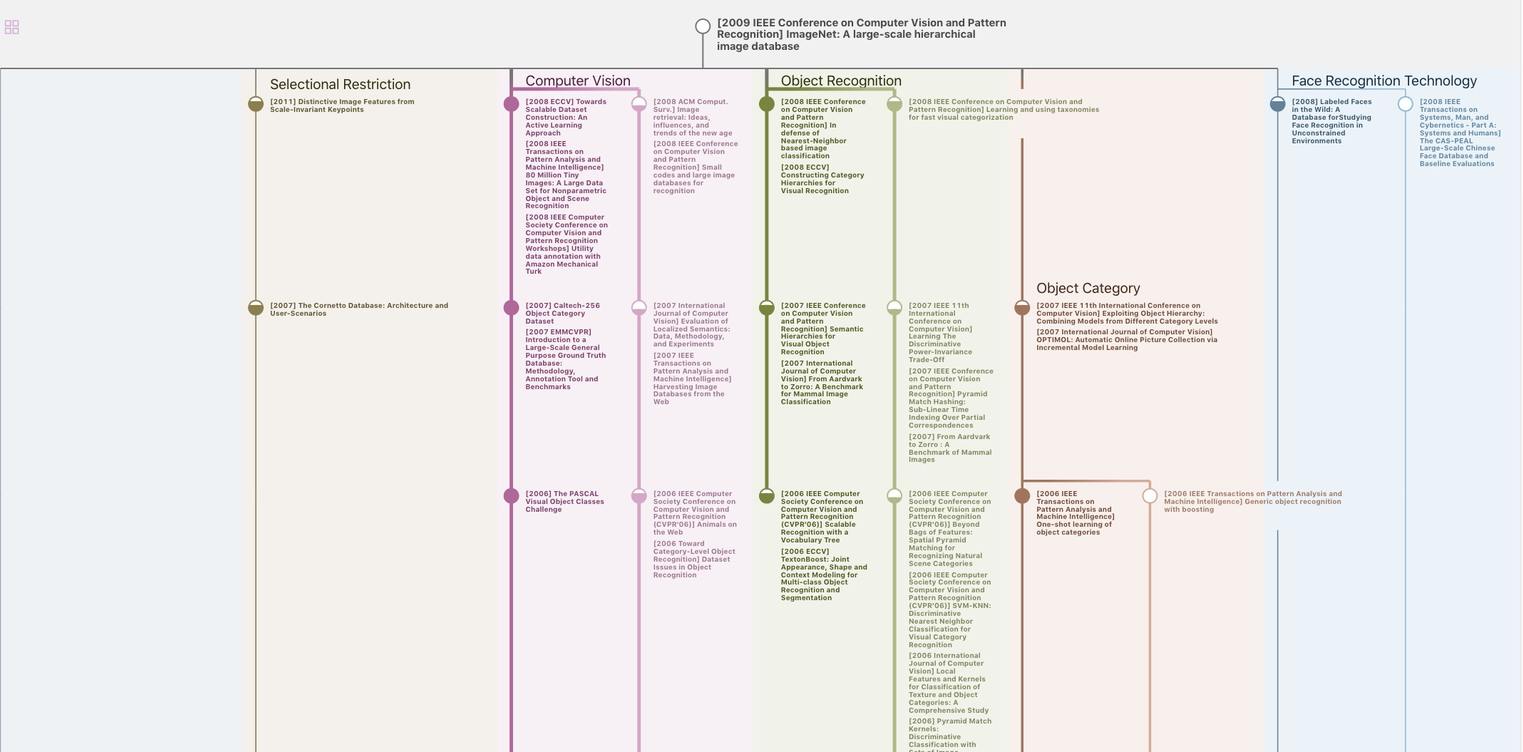
生成溯源树,研究论文发展脉络
Chat Paper
正在生成论文摘要