A Light-weight Online Learning Framework for Network Traffic Abnormality Detection.
WCNC(2023)
摘要
Network traffic monitoring plays a crucial role in maintaining the security and reliability of the communication networks. Although Machine Learning (ML) assisted abnormal traffic detection has been emerged as a promising paradigm, the existing data-driven learning-based approaches are faced with challenges on inefficient traffic feature extraction and high computational complexity, especially when taking the evolving property of traffic process into consideration. To this end, we establish an online learning framework for abnormality traffic detection by embracing Gaussian Process (GP) and Sparse Representation (SR). The contributions of this paper are twofold: 1). We utilize a special kernel, i.e., mixture of Gaussian, to better explore and exploit the evolving traffic characteristics, so as to more accurately model network traffic. 2). To combat noise and modeling error, we formulate a feature vector based on Kullback-Leibler (KL) divergence to measure the difference between normal and abnormal traffic, based on which SR is adopted to perform robust binary classification. Finally, we demonstrate the superiority of the proposed framework in terms of detection accuracy through simulation.
更多查看译文
关键词
abnormal traffic detection,abnormality traffic detection,communication networks,detection accuracy,evolving traffic characteristics,existing data-driven learning-based approaches,inefficient traffic feature extraction,network traffic abnormality detection,network traffic monitoring,normal traffic,online learning framework,traffic process
AI 理解论文
溯源树
样例
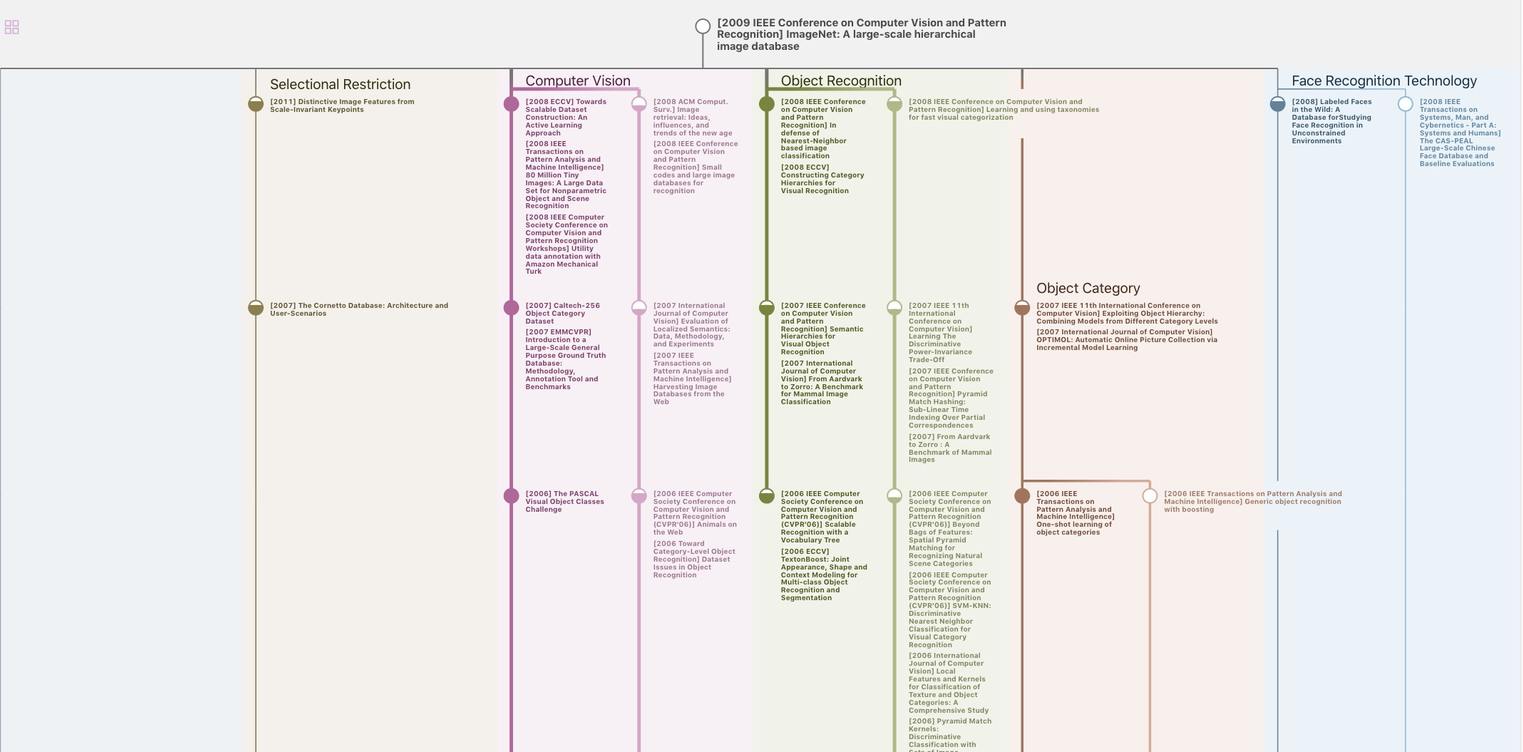
生成溯源树,研究论文发展脉络
Chat Paper
正在生成论文摘要