Few-Shot Network Intrusion Detection Based on Model-Agnostic Meta-Learning with L2F Method.
WCNC(2023)
摘要
Network Intrusion Detection (NID) plays an important role in identifying network threats and ensuring the security of computer and communication systems. However, the existing NID methods face two shortages: 1) Most methods are data-hungry without considering the difficulty of collecting anomaly traffic data, such as zero-day attacks. 2) Few-shot Learning (FSL)-based methods have been proposed recently to relieve the first problem. Whereas these methods rely on the specific model, leading their universality unsatisfactory in different NID application scenarios. Then, we propose a few-shot Model-Agnostic Meta-Learning (MAML) NID framework to tackle the above challenges. We extract both statistical and sequence features from raw network traffic and explicitly find an optimal solution for the NID model by constructing intrusion detection tasks in the 2-way K-shot under the few-shot learning settings. Unfortunately, the MAML forcibly shares initialization, leading to conflicts among tasks and the inability to converge to the optimal position quickly. Therefore, we introduce the L2F (Learn to Forget) attenuation mechanism to dynamically control the conflicts' influence. We conduct sufficient experiments to validate the suitability of our method on few-shot new tasks in various models and achieve the highest detection rate of 98.68% with 2K (K=5,10,15) training samples.
更多查看译文
关键词
Network Intrusion Detection,Few-shot Learning,Model-Agnostic Meta-Learning,L2F Mechanism
AI 理解论文
溯源树
样例
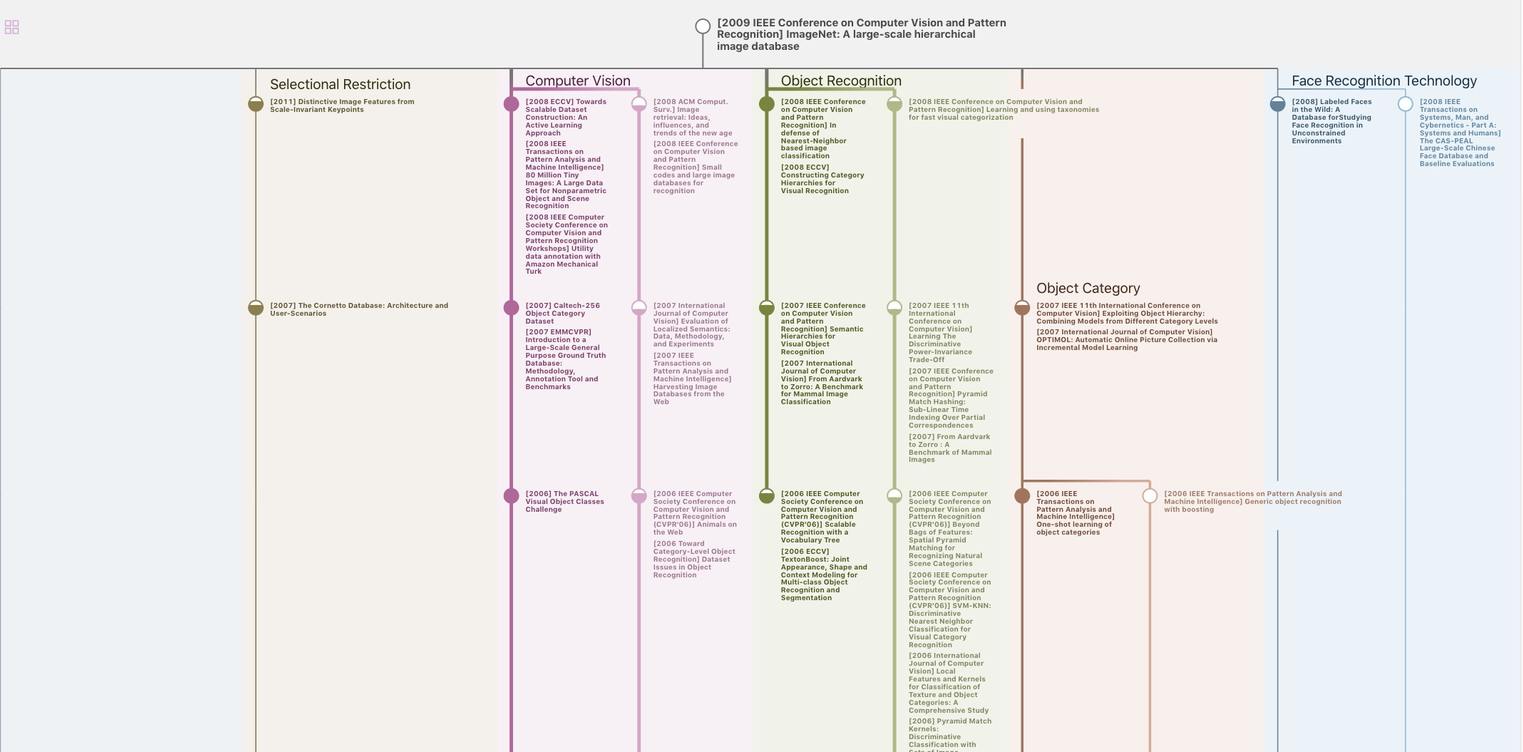
生成溯源树,研究论文发展脉络
Chat Paper
正在生成论文摘要