Explainable Artificial Intelligence for Energy-Efficient Radio Resource Management.
WCNC(2023)
摘要
As wireless systems evolve, the problems of radio resource management (RRM) become harder to solve. Once the additional constraint of energy-efficient utilization of resources is factored in, these problems become even more challenging. Thus, experts started developing solutions based on complex artificial intelligence (AI) models that, unfortunately, suffer from a performance-explainability trade-off. In this work, we propose an explainable AI (XAI) methodology for addressing this tradeoff. Our methodology can be used to generate feature importance explanations of AI models through three XAI methods: (i) Kernel SHapley Additive exPlanations (SHAP), (ii) Counterfactual Explanations for Robustness, Transparency, Interpretability, and Fairness of Artificial Intelligence models (CERTIFAI), and (iii) Anchors. For Anchors, we formulate a new feature importance score based on the feature's presence within the rules built by the method. We then use the generated explanations to improve the understanding of the model and reduce its complexity through a feature selection process. By applying our methodology to a reinforcement learning (RL) agent designed for energy-efficient RRM, we were able to reduce its complexity by approximately 27% according to various metrics, without losing performance. Additionally, we show the possibility to replace the AIbased inference process with an Anchors-based inference process with similar performance and higher interpretability for humans.
更多查看译文
关键词
5G Networks, Energy Efficiency, Radio Resource Management, Reinforcement Learning, Explainable AI
AI 理解论文
溯源树
样例
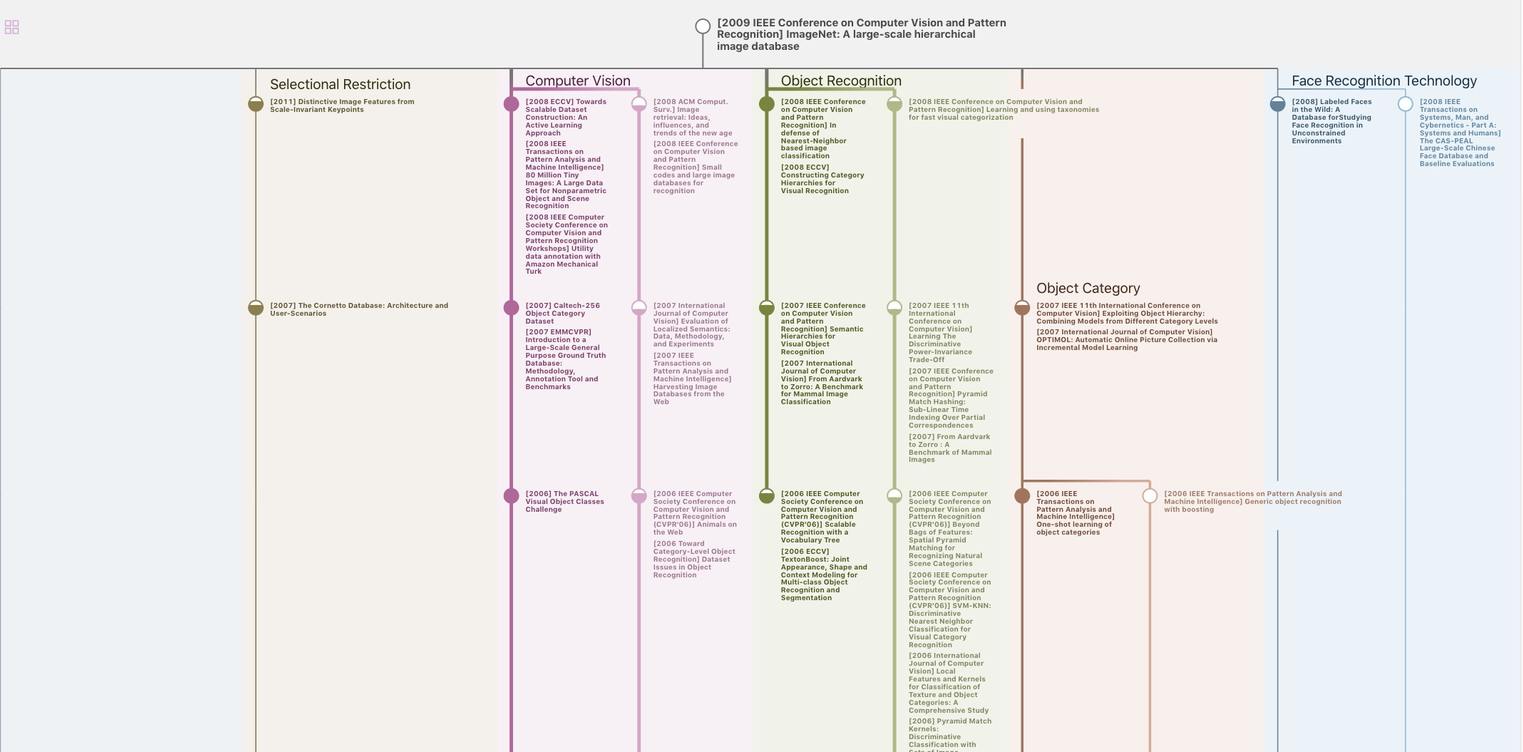
生成溯源树,研究论文发展脉络
Chat Paper
正在生成论文摘要