Federated Learning for Online Resource Allocation in Mobile Edge Computing: A Deep Reinforcement Learning Approach.
WCNC(2023)
摘要
Federated learning (FL) is increasingly considered to circumvent the disclosure of private data in mobile edge computing (MEC) systems. Training with large data can enhance FL learning accuracy, which is associated with non-negligible energy use. Scheduled edge devices with small data save energy but decrease FL learning accuracy due to a reduction in energy consumption. A trade-off between the energy consumption of edge devices and the learning accuracy of FL is formulated in this proposed work. The FL-enabled twin-delayed deep deterministic policy gradient (FL-TD3) framework is proposed as a solution to the formulated problem because its state and action spaces are large in a continuous domain. This framework provides the maximum accuracy ratio of FL divided by the device's energy consumption. A comparison of the numerical results with the state-of-the-art demonstrates that the ratio has been improved significantly.
更多查看译文
关键词
Federated learning, mobile edge computing, online resource allocation, deep reinforcement learning
AI 理解论文
溯源树
样例
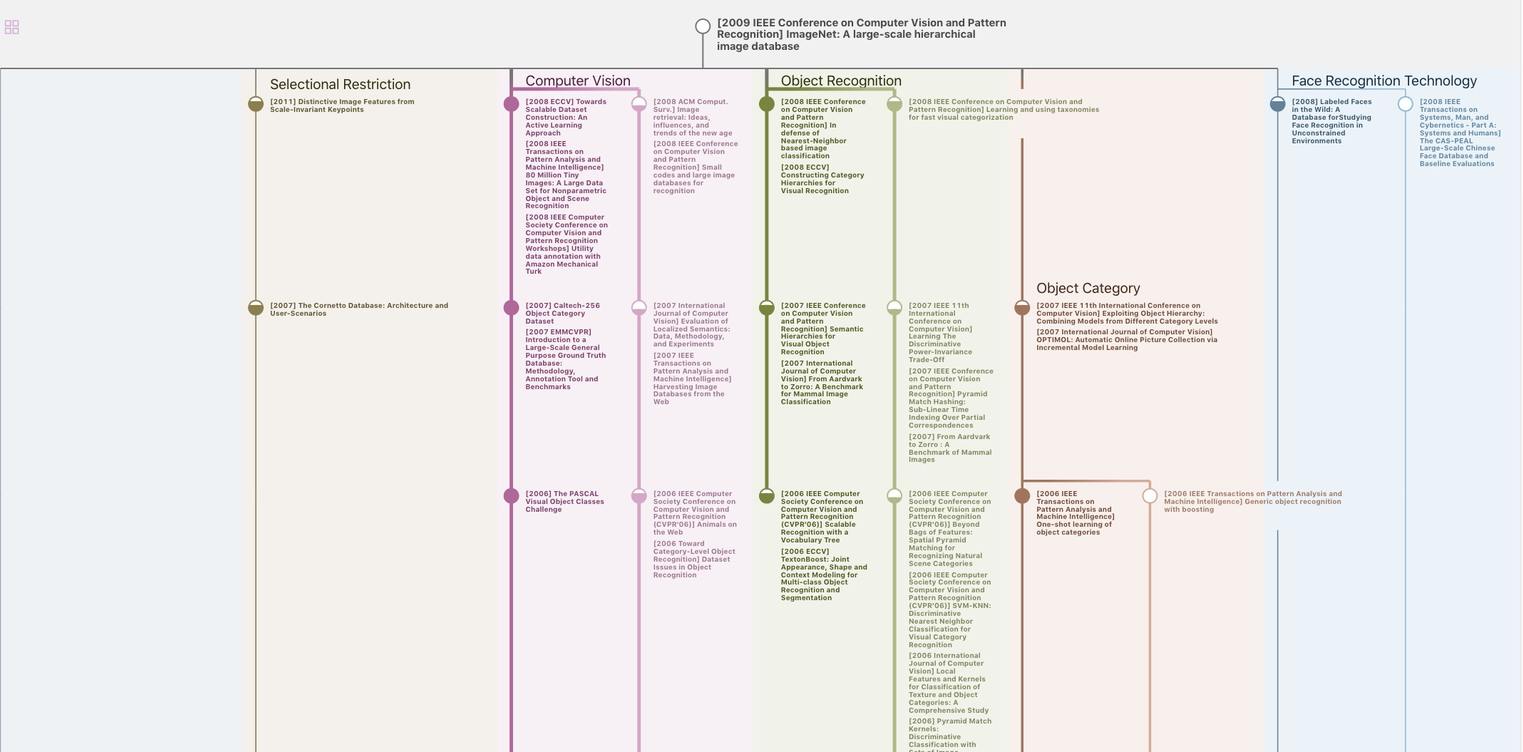
生成溯源树,研究论文发展脉络
Chat Paper
正在生成论文摘要