Arya: Arbitrary Graph Pattern Mining with Decomposition-based Sampling.
NSDI(2023)
摘要
Graph pattern mining is compute-intensive in processing massive amounts of graph-structured data. This paper presents Arya, an ultra-fast approximate graph pattern miner that can detect and count arbitrary patterns of a graph. Unlike all prior approximation systems, Arya combines novel graph decomposition theory with edge sampling-based approximation to reduce the complexity of mining complex patterns on graphs with up to tens of billions of edges, a scale that was only possible on supercomputers. Arya can run on a single machine or distributed machines with an Error-Latency Profile (ELP) for users to configure the running time of pattern mining tasks based on different error targets. Our evaluation demonstrates that Arya outperforms existing exact and approximate pattern mining solutions by up to five orders of magnitude. Arya supports graphs with 5 billion edges on a single machine and scales to 10-billion-edge graphs on a 32-server testbed.
更多查看译文
AI 理解论文
溯源树
样例
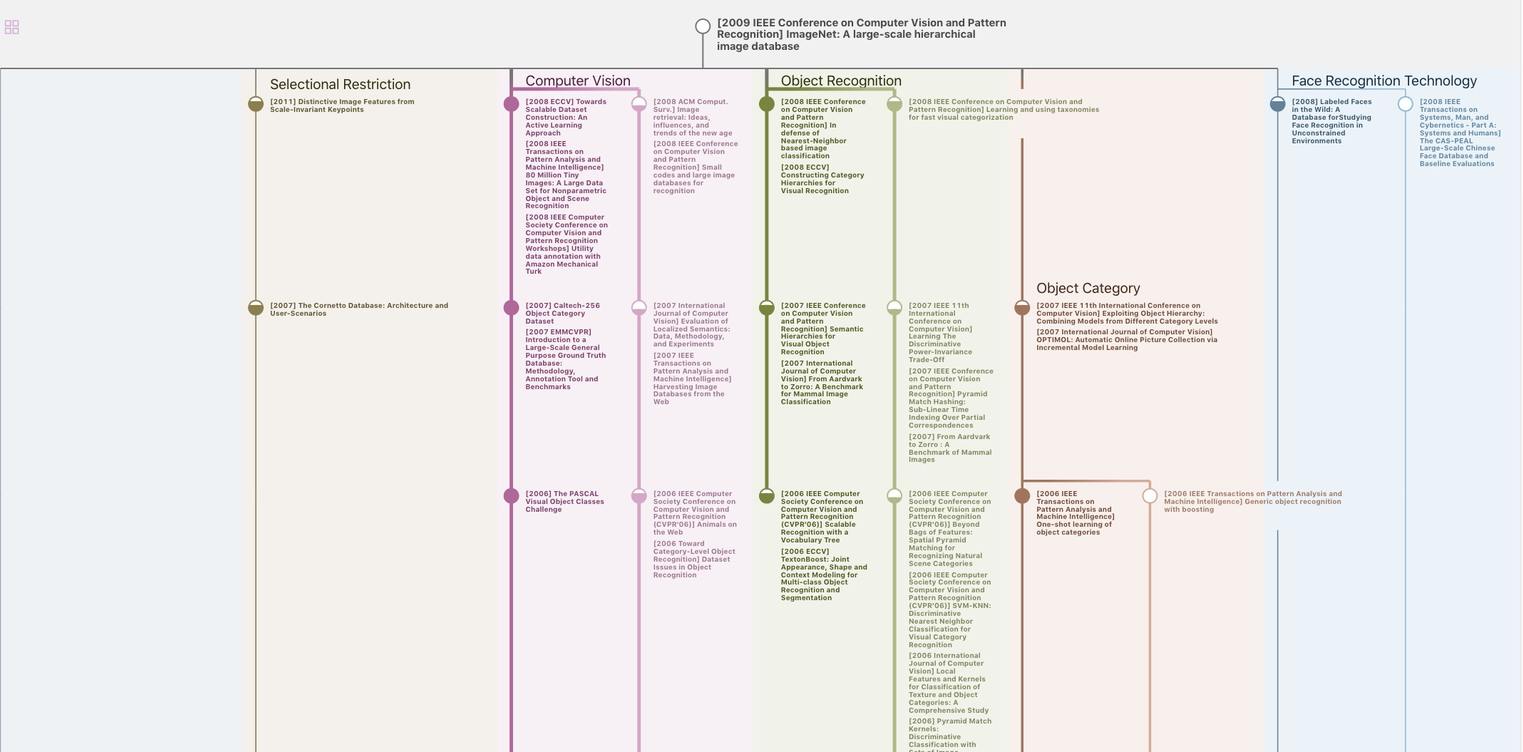
生成溯源树,研究论文发展脉络
Chat Paper
正在生成论文摘要