Prototype Softmax Cross Entropy: A New Perspective on Softmax Cross Entropy.
SCIA (2)(2023)
Abstract
In this work, we consider supervised learning for image classification. Inspired by recent results in the field of supervised contrastive learning, we focus on the loss function for the feature encoder. We show that Softmax Cross Entropy (SCE) can be interpreted as a special kind of loss function in contrastive learning with prototypes. This insight provides a completely new perspective on cross entropy, allowing the derivation of a new generalized loss function, called Prototype Softmax Cross Entropy (PSCE), for use in supervised contrastive learning. We prove both mathematically and experimentally that PSCE is superior to other loss functions in supervised contrastive learning. It only uses fixed prototypes, so no self-organizing part of contrastive learning is required, eliminating the memory bottleneck of previous solutions in supervised contrastive learning. PSCE can also be used equally successfully for both balanced and unbalanced data.
MoreTranslated text
Key words
prototype softmax cross entropy
AI Read Science
Must-Reading Tree
Example
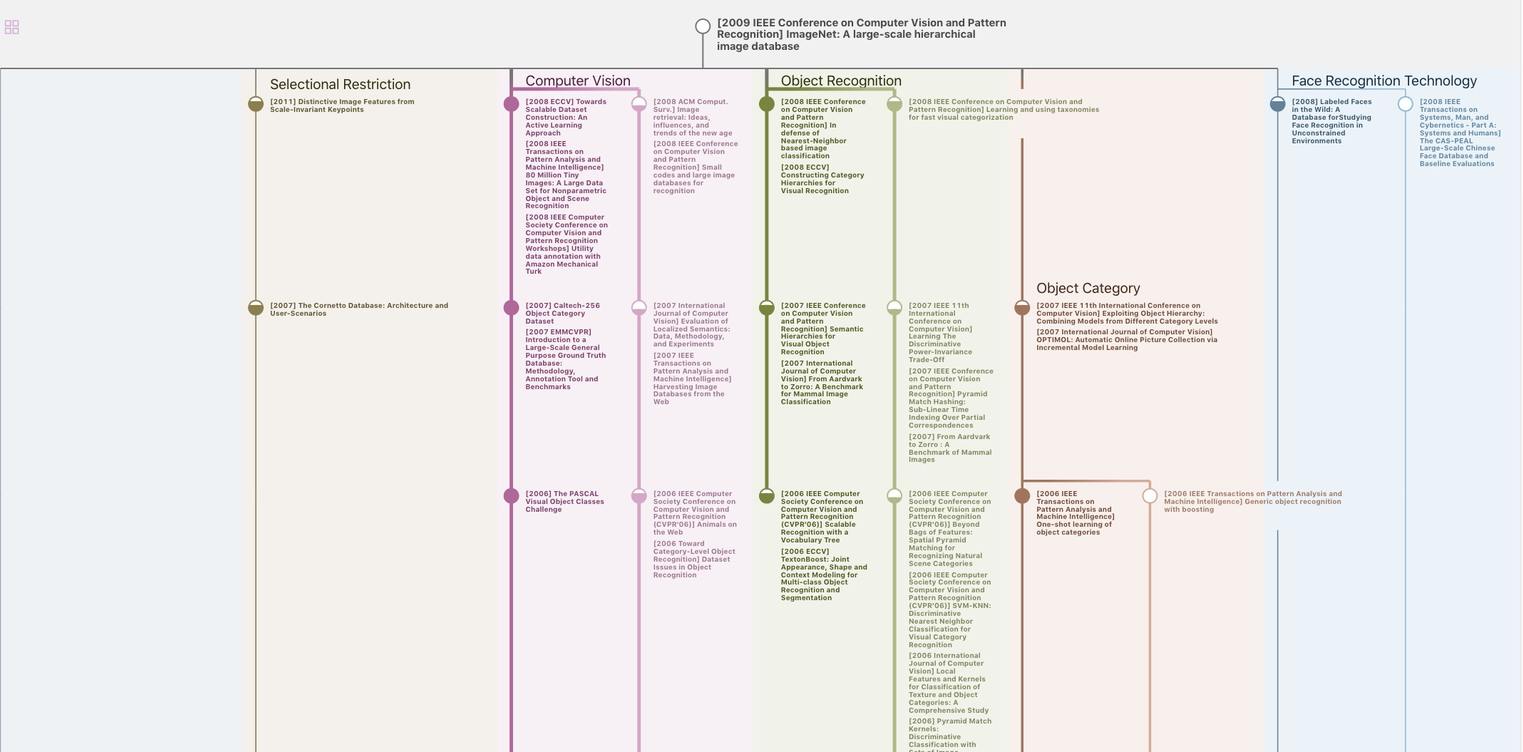
Generate MRT to find the research sequence of this paper
Chat Paper
Summary is being generated by the instructions you defined