Comparing Machine Learning Algorithms for Soil Salinity Mapping Using Topographic Factors and Sentinel-1/2 Data: A Case Study in the Yellow River Delta of China.
Remote. Sens.(2023)
摘要
Soil salinization is a critical and global environmental problem. Effectively mapping and monitoring the spatial distribution of soil salinity is essential. The main aim of this work was to map soil salinity in Shandong Province located on the Yellow River Delta of China using Sentinel-1/2 remote sensing data and digital elevation model (DEM) data, coupled with soil sampling data, and combined with four regression models: support vector regression (SVR), stepwise multi-regression (SMR), partial least squares regression (PLSR) and random forest regression (RFR). For these purposes, 60 soil samples were collected during the field survey conducted from 9 to 14 October 2019, corresponding to the Sentinel-1/2 and DEM data. Then we established a soil salinity and feature dataset based on the sampled data and the features extracted from Sentinel-1/2 and DEM data. This study adopted the feature importance of the RF model to screen all features. The results showed that the CRSI index made the greatest contribution in retrieving soil salinity in this region. In this paper, 18 sampling points were used to validate and compare the performance of the four models. The results reveal that, compared with the other regression models, the PLSR model has the best performance (R-2 = 0.66, and RMSE = 1.30). Finally, the PLSR method was used to predict the spatial distribution of soil salinity in the Yellow River Delta. We concluded that the model can be used effectively for the quantitative estimation of soil salinity and provides a useful tool for ecological construction.
更多查看译文
关键词
soil salinity, DEM, Sentinel-1, 2, machine learning, Yellow River Delta, China
AI 理解论文
溯源树
样例
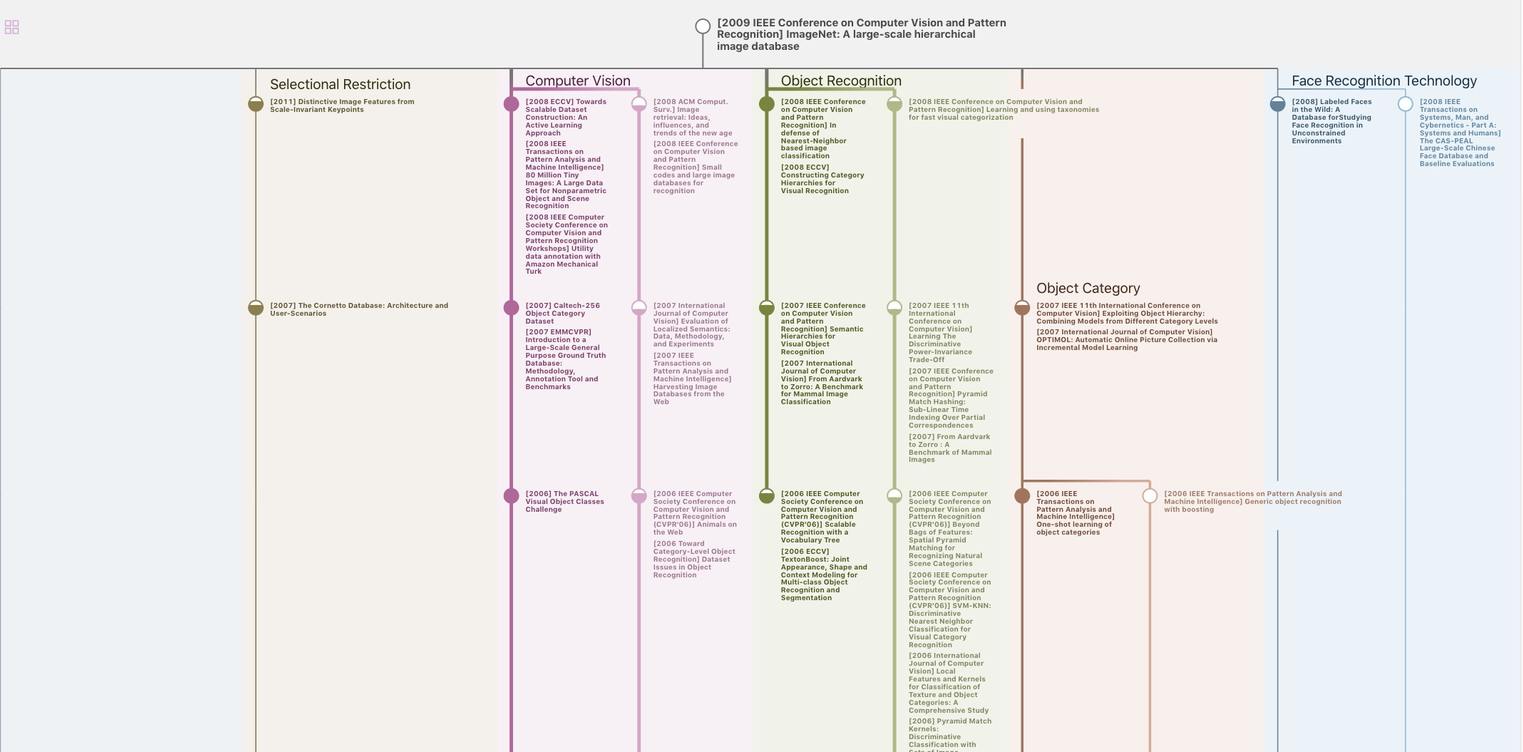
生成溯源树,研究论文发展脉络
Chat Paper
正在生成论文摘要