A Multi-Feature Fusion and Attention Network for Multi-Scale Object Detection in Remote Sensing Images.
Remote. Sens.(2023)
摘要
Accurate multi-scale object detection in remote sensing images poses a challenge due to the complexity of transferring deep features to shallow features among multi-scale objects. Therefore, this study developed a multi-feature fusion and attention network (MFANet) based on YOLOX. By reparameterizing the backbone, fusing multi-branch convolution and attention mechanisms, and optimizing the loss function, the MFANet strengthened the feature extraction of objects at different sizes and increased the detection accuracy. The ablation experiment was carried out on the NWPU VHR-10 dataset. Our results showed that the overall performance of the improved network was around 2.94% higher than the average performance of every single module. Based on the comparison experiments, the improved MFANet demonstrated a high mean average precision of 98.78% for 9 classes of objects in the NWPU VHR-10 10-class detection dataset and 94.91% for 11 classes in the DIOR 20-class detection dataset. Overall, MFANet achieved an mAP of 96.63% and 87.88% acting on the NWPU VHR-10 and DIOR datasets, respectively. This method can promote the development of multi-scale object detection in remote sensing images and has the potential to serve and expand intelligent system research in related fields such as object tracking, semantic segmentation, and scene understanding.
更多查看译文
关键词
remote sensing images, multi-scale object detection, multi-feature fusion and attention network, multi-branch convolution, attention mechanism, loss function
AI 理解论文
溯源树
样例
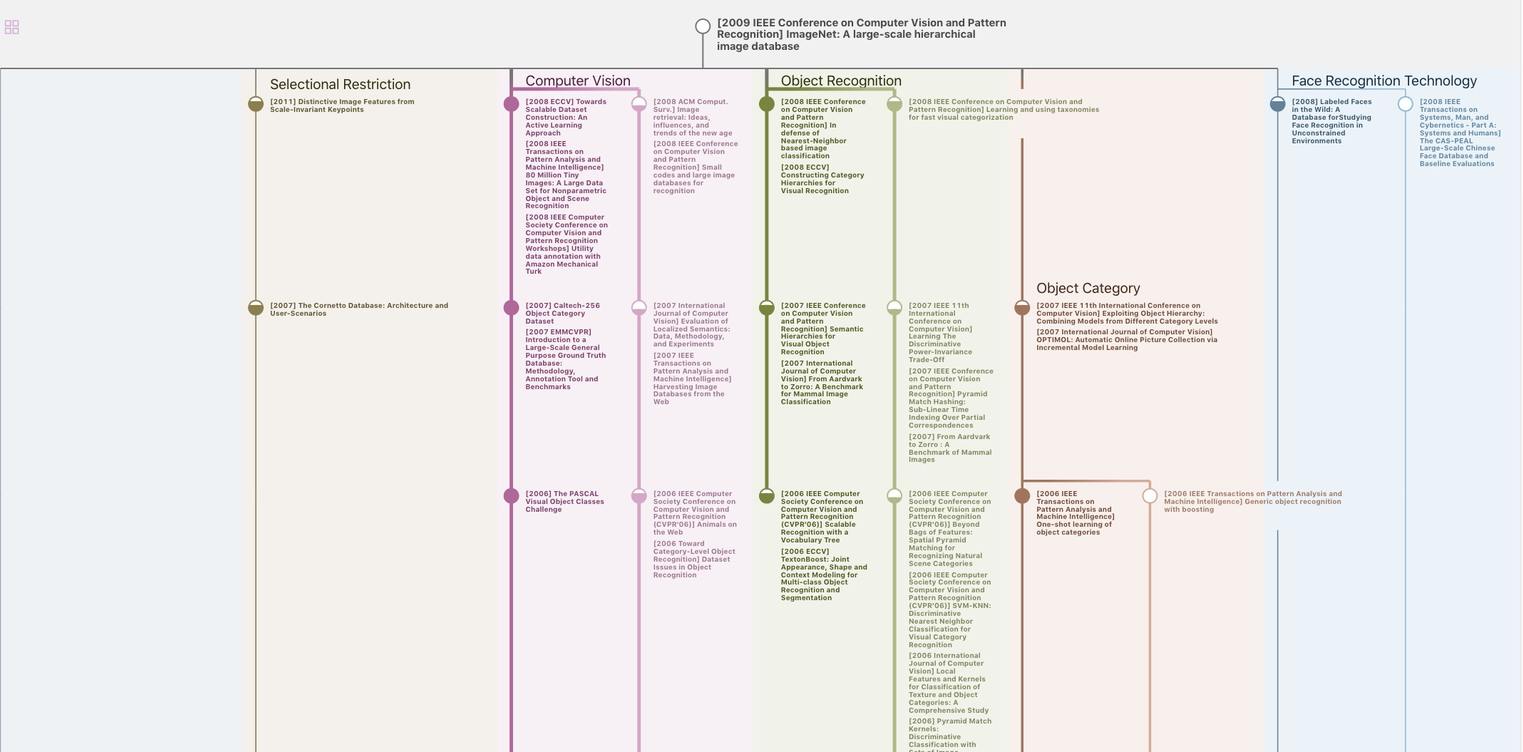
生成溯源树,研究论文发展脉络
Chat Paper
正在生成论文摘要